Statistics
Extreme analysis of seizures
The statistics of extreme events helps to better understand epileptic seizures.

A new and powerful approach for analyzing seizures in epilepsy patients has been developed using electroencephalogram (EEG) records of brainwave patterns. The method holds promise for better understanding the mechanisms of seizures.
“Despite being a well-studied neuronal disorder, predicting an epileptic seizure event remains difficult,” says Matheus Guerrero, a Ph.D. student who led the study. “This work is the first attempt to understand the epileptic seizure process from the vantage point of extreme value theory – a field of statistics dealing with large deviations from the median of probability distributions.”
As Guerrero explains, the brain is permeated by electric fields generated by neuronal activity. When a seizure occurs, there is a sudden and uncontrolled disturbance of these electrical patterns. Working with Raphaël Huser from the Extreme Statistics Group and Hernando Ombao from the Biostatistics Group, Guerrero’s study focused on whether extreme value theory could be used to better understand seizure events from EEG records.
“Current statistical methods for analyzing EEGs consider the oscillatory and cross-dependence patterns in the entire distribution of brainwaves,” Guerrero says. “However, these methods completely neglect the specific patterns that might occur in the tails of the distributions, which is where relatively rare extreme events like seizures occur.”
The team was specifically looking for cross-interactions in brainwave patterns between different areas of the brain during seizure events, which are expected to differ significantly from those during nonseizure periods. Conventional statistical methods are unable to differentiate between dependence and independence in the tail versus the overall probability distribution, meaning they might miss potentially important seizure-specific connections among unexpected brain regions.
“We concentrated our attention on damaged areas of the brain with high EEG amplitudes,” says Guerrero. “Using this approach, we were able to model brain connectivity in the extremal setting of a seizure event.”
The method, called Conex-Connect, captures changes in brain connectivity at various EEG frequencies that could not be captured by existing methods. Applying the method to real EEG seizure data revealed that extremal dependence is high but notably stable pre-seizure, while it is weaker and more chaotic after seizure onset. Importantly, Conex-Connect can capture changes in brain connectivity in different frequency bands, which other methods cannot.
“The results from Conex-Connect could be used to create inputs for machine learning algorithms in order to predict seizures,” Guerrero says. “Our work shows the importance and relevance of extreme value theory in neuroscience, opening a promising new field for brain-related research.
References
- Guerrero, M.B, Huser, R. & Ombao, H. Conex–Connect: Learning patterns in extremal brain connectivity from multi-channel EEG data. Annals of Applied Statistics (2022).| article
You might also like
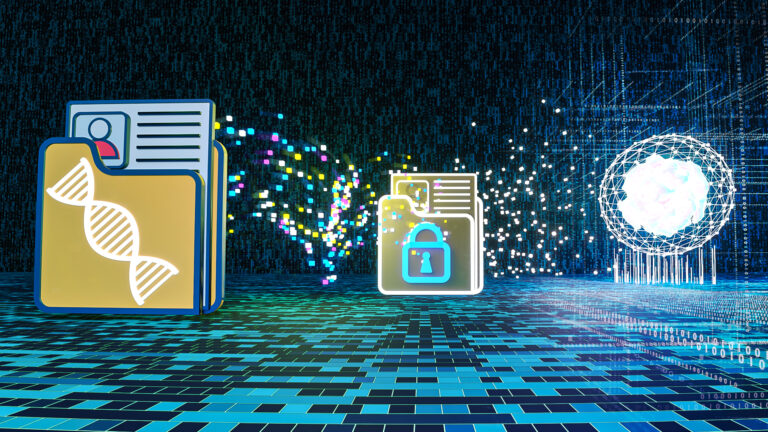
Bioengineering
Shuffling the deck for privacy
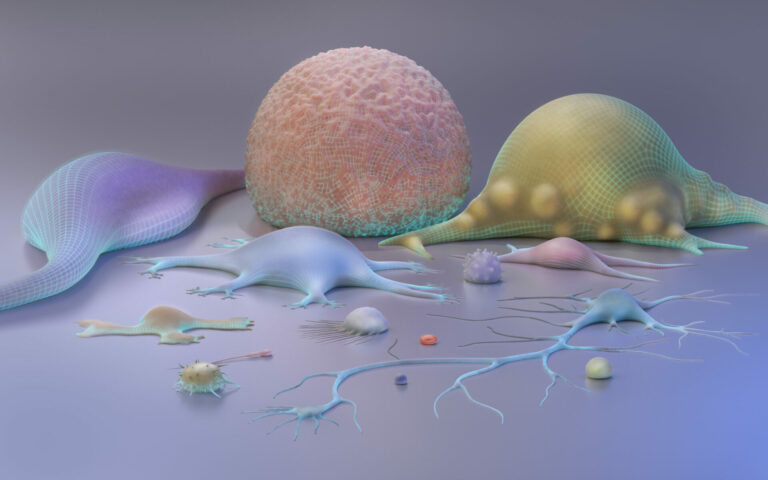
Bioengineering
AI for cells helps illuminate their identity
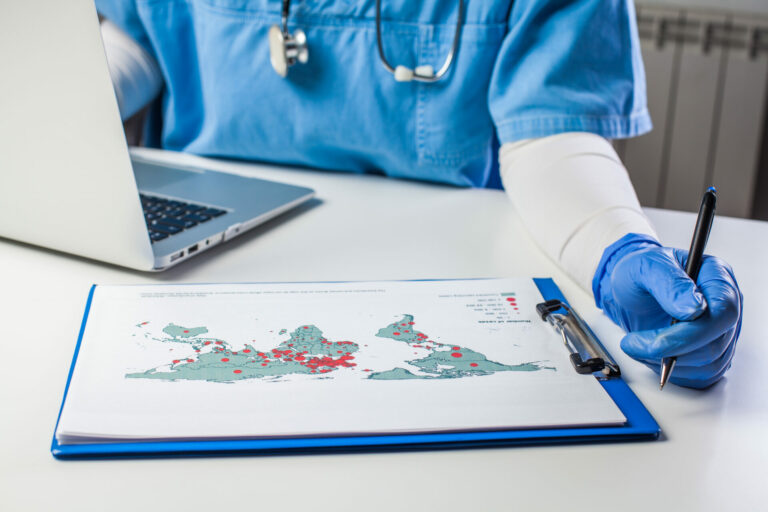
Applied Mathematics and Computational Sciences
Global look at sex differences in young people's mortality
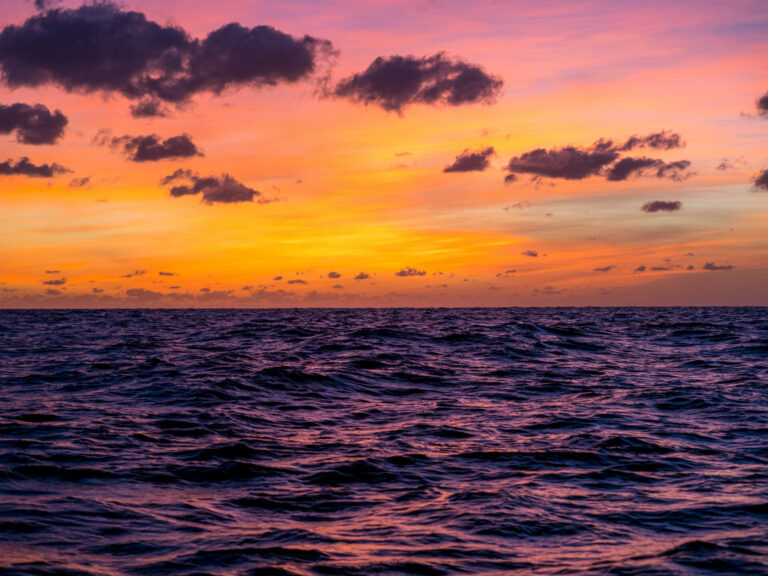
Applied Mathematics and Computational Sciences
Going likelihood-free with neural networks
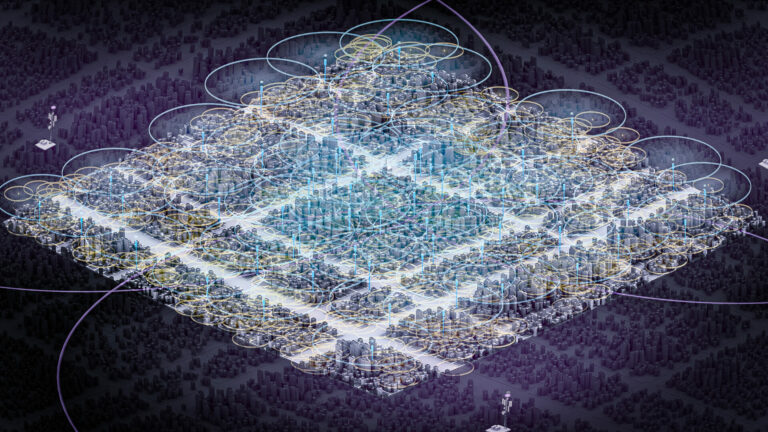
Applied Mathematics and Computational Sciences
A simple solution for frequency sharing
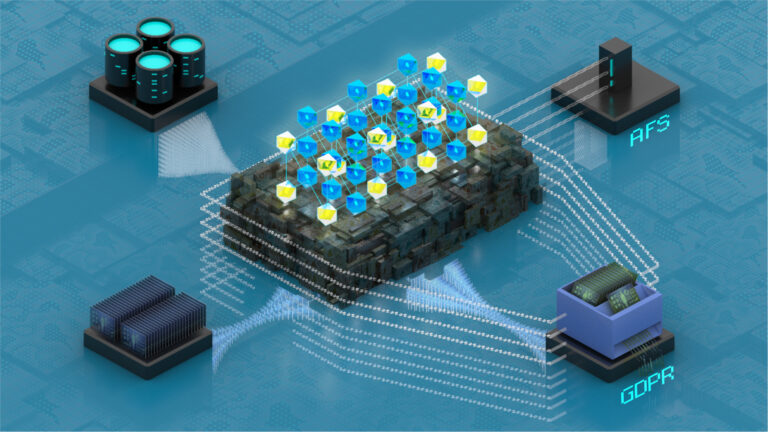
Bioengineering
Safeguarding the right to be forgotten
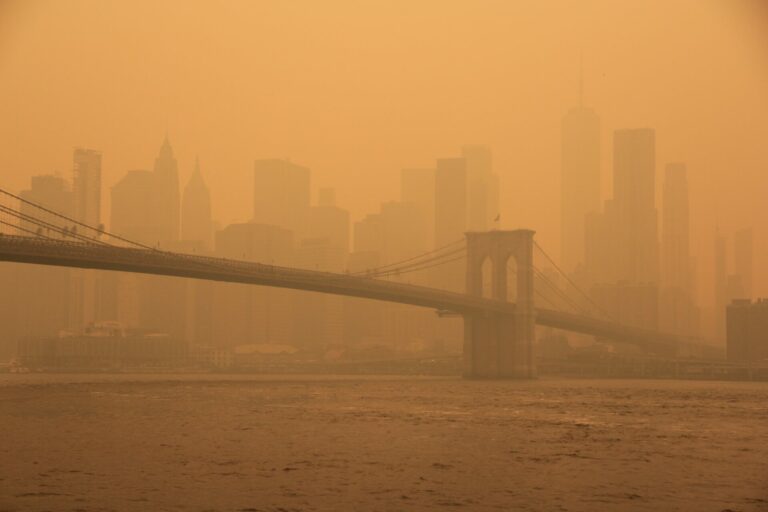
Applied Mathematics and Computational Sciences
Neural networks give deeper insights
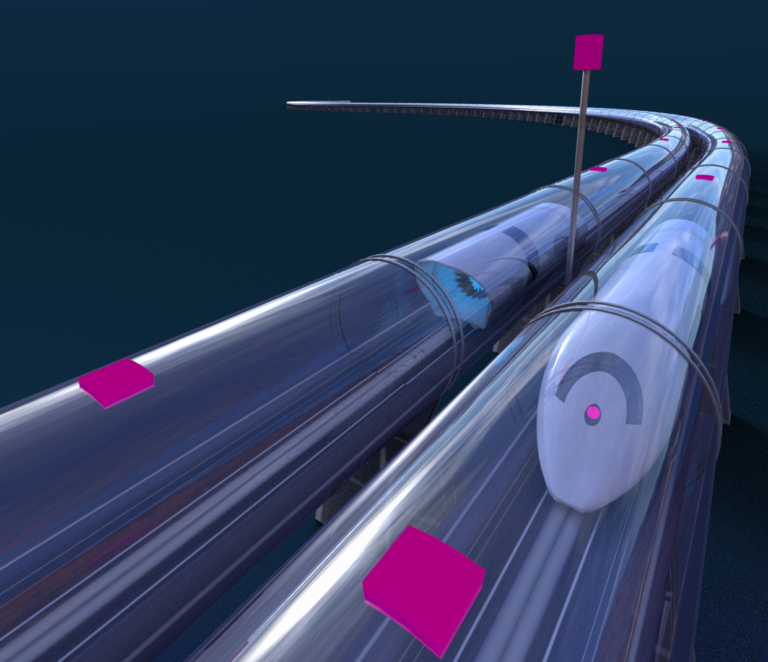
Applied Mathematics and Computational Sciences