Bioengineering | Bioscience | Computer Science | Statistics
Safeguarding the right to be forgotten
An open-source software can help align artificial intelligence applications in healthcare with data privacy regulations.
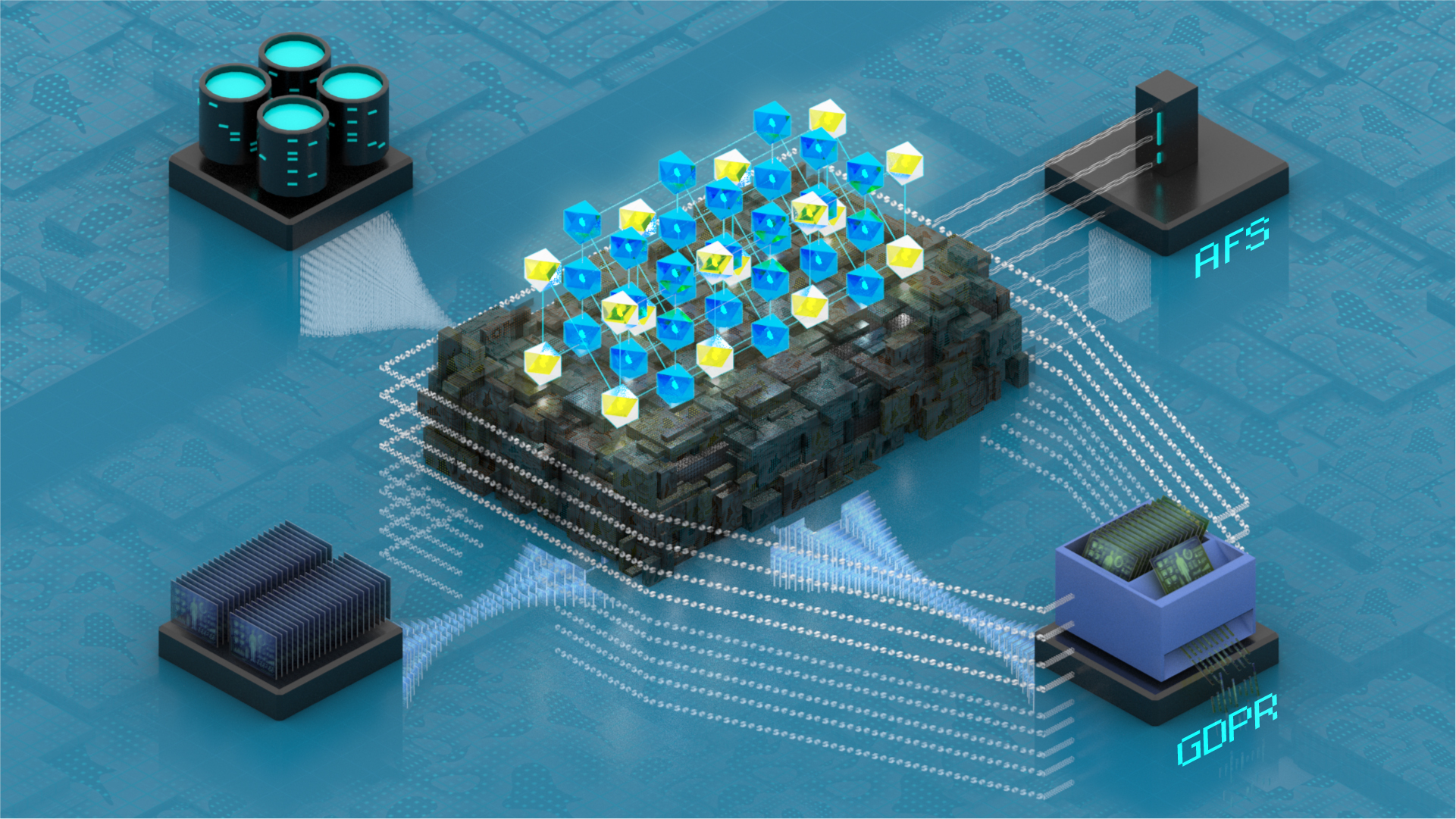
Audit to forget software (AFS) to remove patients’ information from pretrained artificial intelligence (AI) models in healthcare settings has been developed by KAUST to secure their right to data privacy.[1]
As patient data are increasingly used to teach AI models or machine learning algorithms to make disease diagnosis and treatment decisions, there is growing concern about their right to withdraw private information. “With the development of data science, machine learning and deep learning techniques, patients’ right to be forgotten is usually neglected or violated,” says Juexiao Zhou, Ph.D. candidate at KAUST.
Under the supervision of Xin Gao, Zhou and colleagues demonstrate how AFS can revoke patient data from deep learning models while maintaining model performance. “Our software solution unifies auditing and forgetting processes, ensuring that sensitive data is effectively removed from the target model,” Zhou explains.
AFS employs auditing to determine whether the data of certain patients were used to train the target AI model. Using the results from auditing, AFS guides the forgetting process, which involves the unlearning of information related to the target dataset from the pretrained model. “We designed an approach, termed ‘knowledge purification,’ to selectively transfer the necessary knowledge from the pretrained model to the model that will undergo forgetting in a way that preserves the model performance to the maximum extent,” he adds.
The researchers applied the software to four different datasets of different sizes and forms, including medical images and electronic health records. They showed how AFS could generate smaller models without significantly affecting their accuracy of prediction, demonstrating the versatility and effectiveness of AFS for handling forgetting requests. “Our results highlight the usability of AFS and its potential applications in real-world intelligent healthcare to enhance privacy protection and data revocation rights,” Zhou says.
Zhou anticipates that this type of software will be widely used. “AFS could be applied in other fields that are using AI, such as finance or education, where there are also concerns about users’ data privacy and the right to be forgotten.”
As the team continues to optimize the software by incorporating more auditing metrics, they have also started exploring the possibility of applying AFS to the forgetting of private information in large language models (LLMs), such as SkinGPT-4.
AFS represents a significant step toward ensuring data privacy in the context of evolving AI technologies.
Reference
- Zhou, J., Li, H., Liao, X., Zhang, B., He, W., Li, Z., Zhou, L., & Gao, X. A unified method to revoke the private data of patients in intelligent healthcare with audit to forget. Nature Communications 14, 6255 (2023).| article.
You might also like
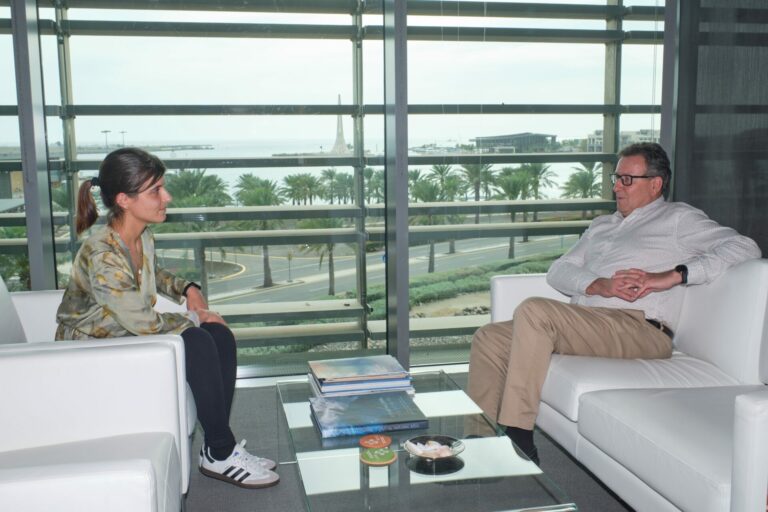
Bioscience
Cataloging the complexity of the ocean genome
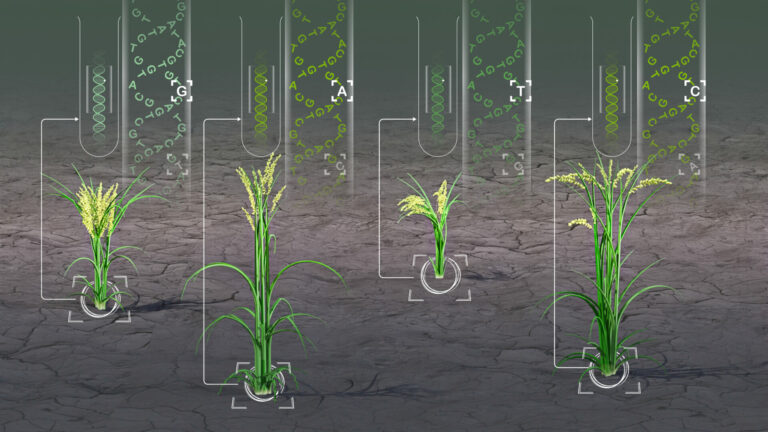
Bioengineering
Analytic tool reveals more cream of the crops
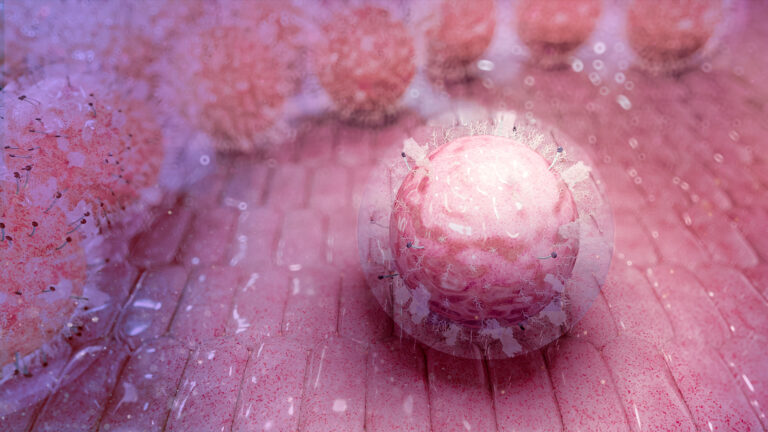
Bioengineering
Often overlooked stem cells hold hidden powers for blood disease treatments
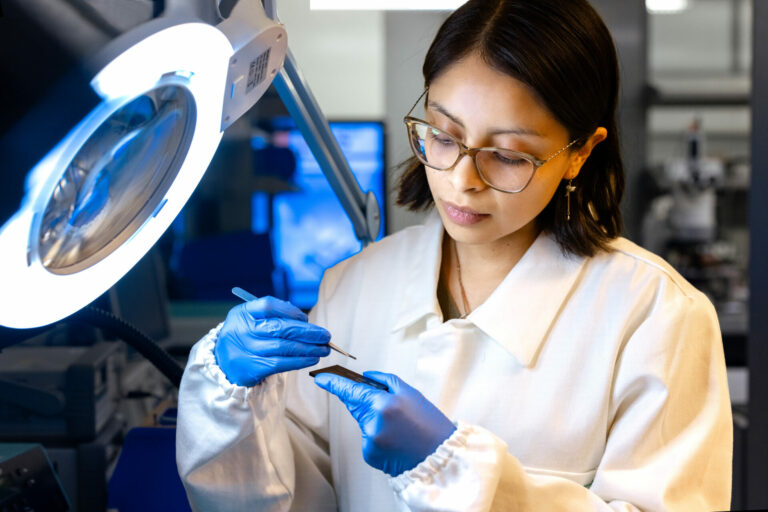
Bioengineering
Giving voice to the voiceless through assistive technology
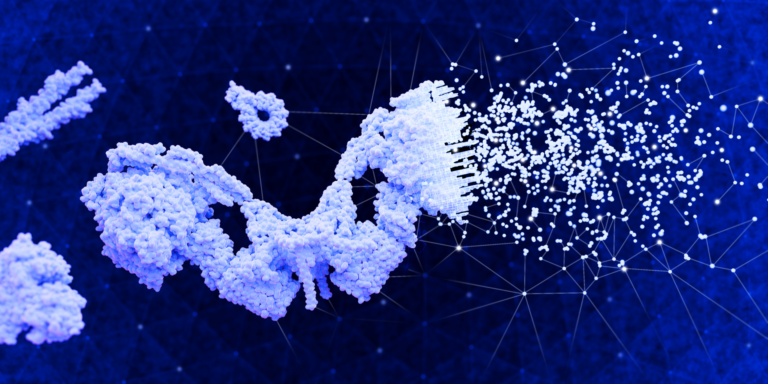
Bioengineering
AI tool predicts function of unknown proteins
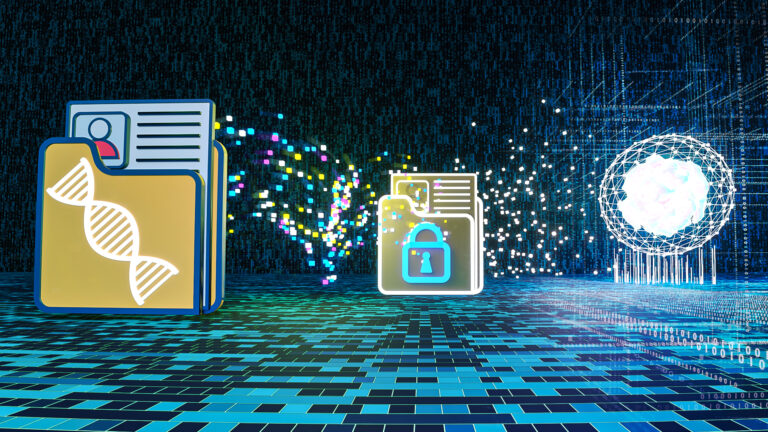
Bioengineering
Shuffling the deck for privacy
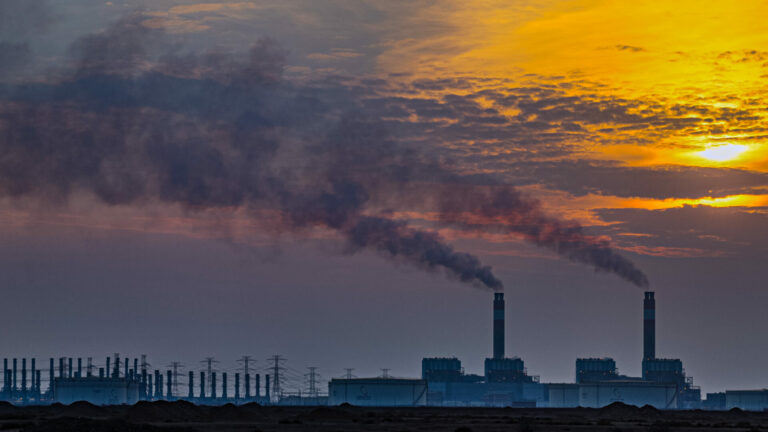
Bioscience
Sediments reveal core stressors on Red Sea ecosystems
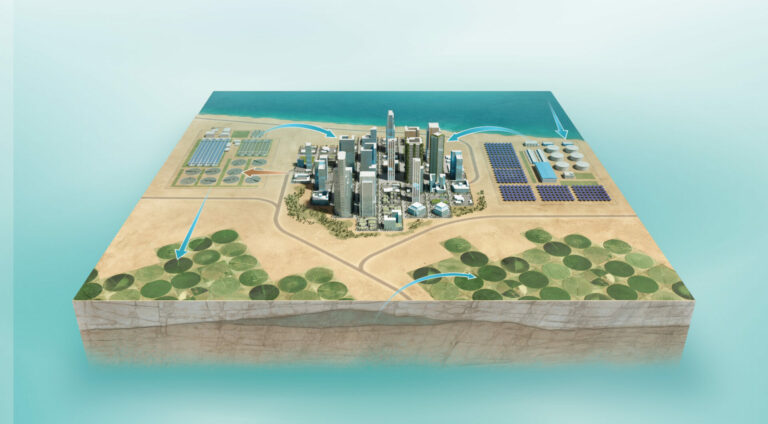
Bioengineering