Bioengineering | Bioscience | Computer Science | Statistics
AI for cells helps illuminate their identity
Inspired by large language models that power ChatGPT, an AI algorithm offers a new way to annotate cells.
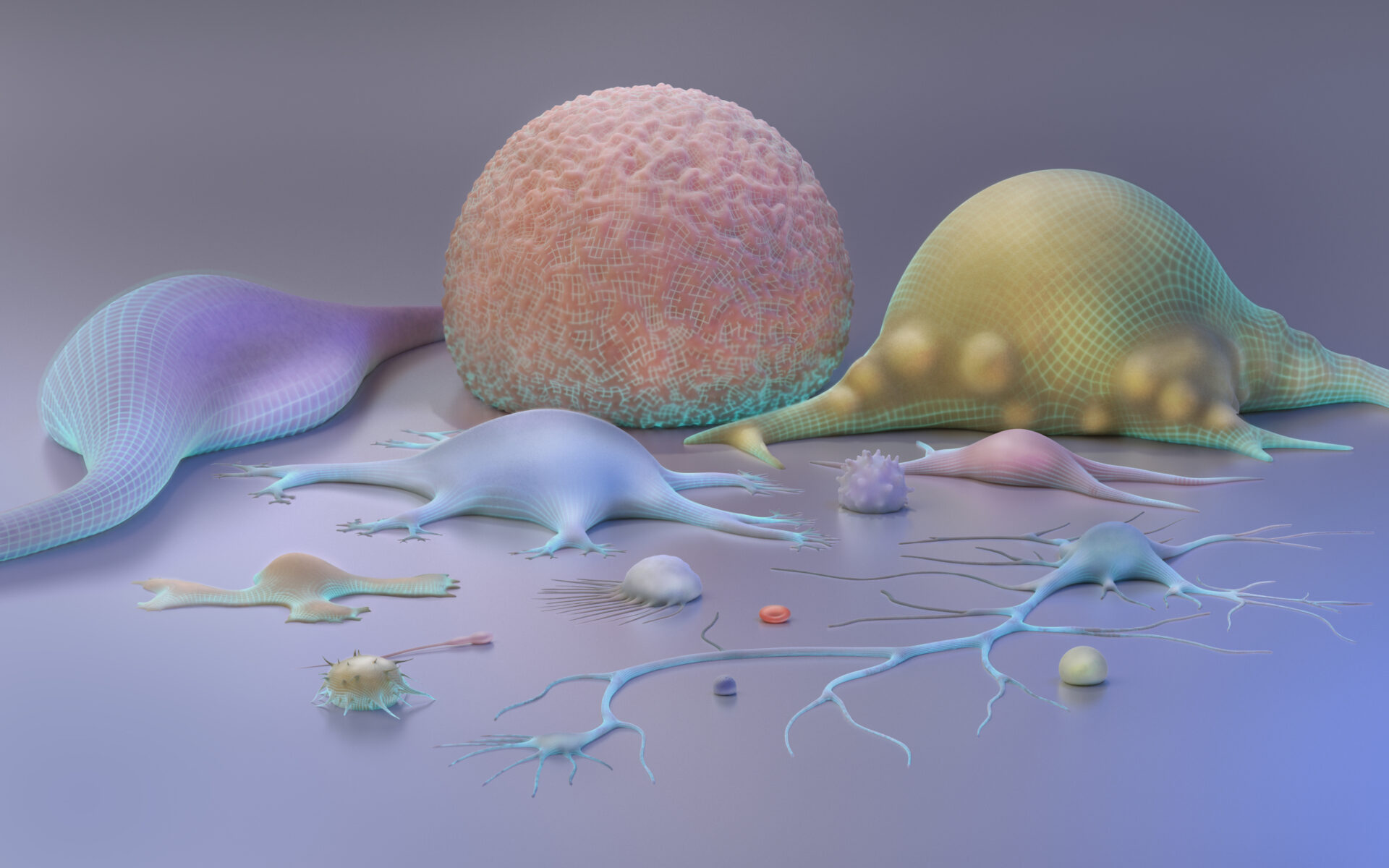
In 2022, computational biologist Jesper Tegnér saw a paper that captured his attention. A team of Chinese and American scientists had proposed a novel AI-powered technique — called single-cell Bidirectional Encoder Representation from Transformers (scBERT) — that appeared to solve one of the field’s most challenging problems: cell type annotation.
Intrigued, Tegnér wanted to test the method for himself and see if it could be applied to other datasets.
Identifying a cell, based on its RNA transcript or the genes it expresses, is an exacting but important task. Information gleaned from the study of single cells allows scientists to better understand their makeup, function and interaction with other cells. This offers critical insights into human diversity, as well as diseases that result from genetic mutations.
This could lead to new treatment options in the future, says Tegnér. “Researchers are trying to identify what kinds of cells are in different organs and see if we can reprogram them with stem-cell therapy.”
Given that there are up to 36 trillion cells in the average adult, trying to manually “read” each individual RNA transcript is painfully time consuming. “Fortunately, scientists are now developing tools to do this in a more automated way,” says Tegnér. “And those that use machine learning are very exciting.”
Of these, scBERT appears promising. Similar to large language AI models that power ChatGPT — where users input text queries and obtain a sweep of word-based material ranging from emails to essays and poems to computer code — scBERT employs a specialized deep learning model, called a transformer, that is trained on vast quantities of data to read RNA “transcriptional grammar” of single cells. From this, it predicts a cell’s identity based on “the context and correlation between the different genes in that same cell,” explains research scientist Sumeer Khan who co-led the work.
The team has successfully reproduced the 2022 results, affirming that scBERT can effectively annotate cells and detect new cell types. However, the AI model performed poorly when applied to a new dataset with a skewed distribution of cell types. “Sometimes when you oversample one cell type, the system may not learn very well on other cell types,” explains Alberto Maíllo, a computer engineer at Tegnér’s lab.[1][2]
To address this, the researchers then devised various mitigation methods, including sampling the data in different ways and altering the architectural design of the AI model. “With these techniques, we found we are actually doing better than the original study,” says Tegnér.
The team is continuing to work on other AI-powered approaches for cell-type annotation. What they discover may one day help scientists create personalized medicine, in line with the Saudi Vision 2030 aim of transforming healthcare, Tegnér says. “Large language-type biology models give you greater precision and more detailed data: They are the key to moving from average medicine to personalized medicine.”
Reference
- Khan, S.A., Maillo, A., Lagani, V., Lehmann, R., Kiani, N.A, Gomez-Cabrero, D., & Tegner, J.N. Resuability report: Learning the transcriptional grammar in single-cell RNA sequencing data using transformers. Nature Machine Intelligence (2023).| article.
- Tegner, J.N. Translating single-cell genomics into cell types. Nature Machine Intelligence (2023).| article.
You might also like
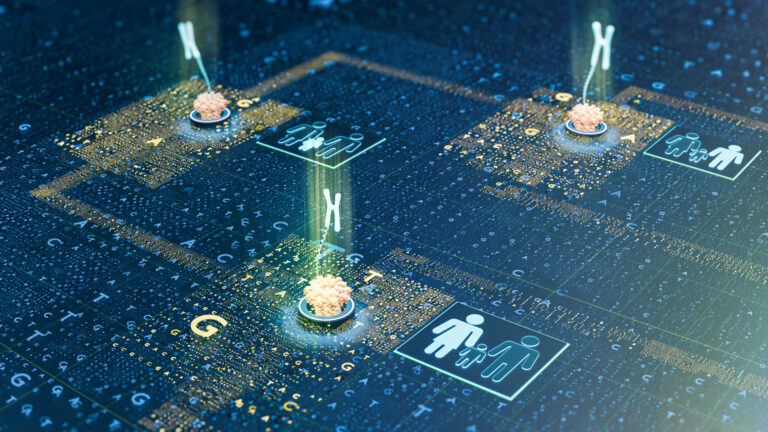
Bioengineering
Pioneering technique transforms genetic disorder diagnoses
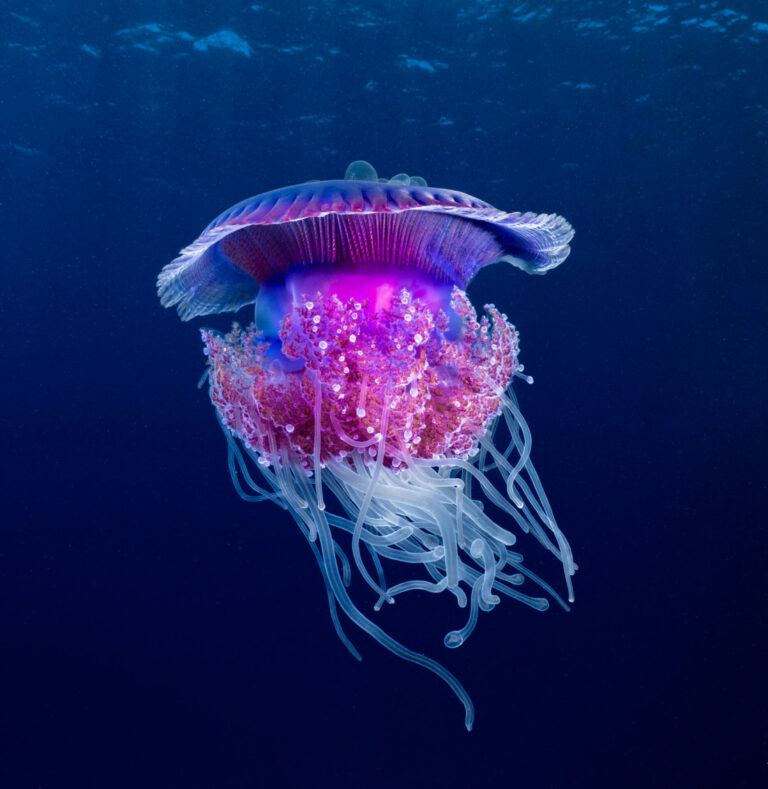
Bioscience
Cataloging the complexity of the ocean genome
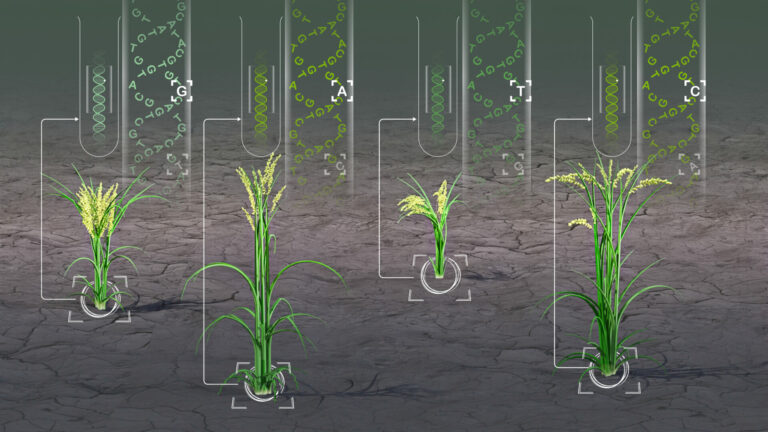
Bioengineering
Analytic tool reveals more cream of the crops
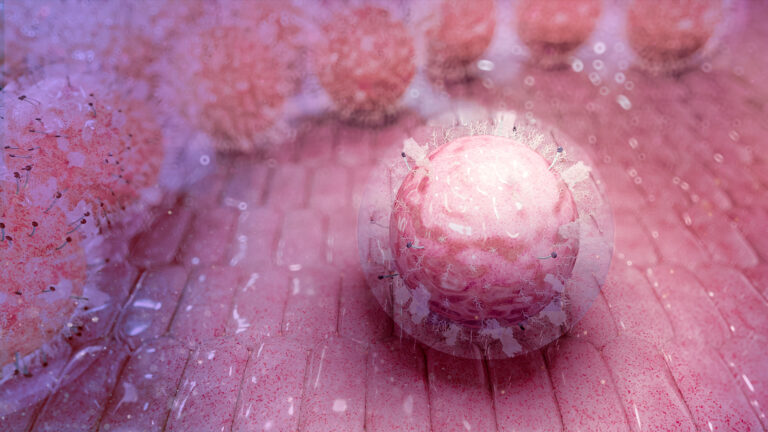
Bioengineering
Often overlooked stem cells hold hidden powers for blood disease treatments
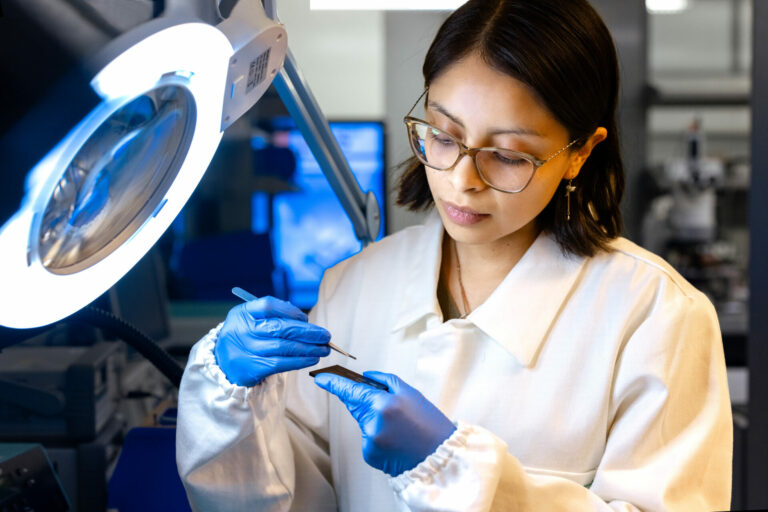
Bioengineering
Giving voice to the voiceless through assistive technology
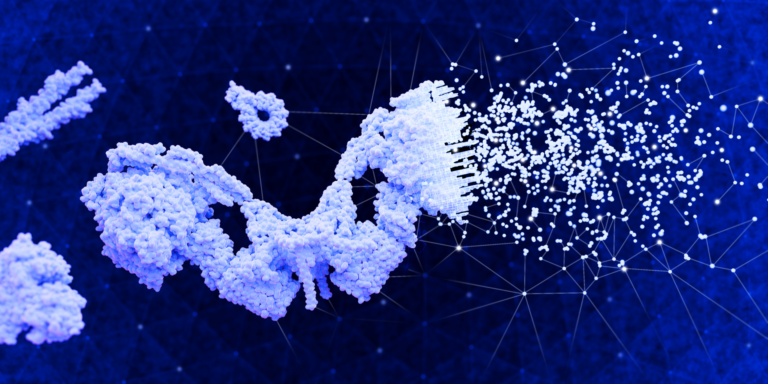
Bioengineering
AI tool predicts function of unknown proteins
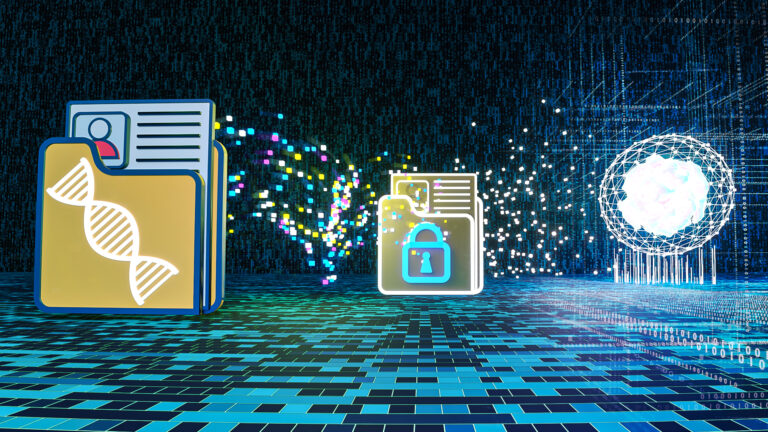
Bioengineering
Shuffling the deck for privacy
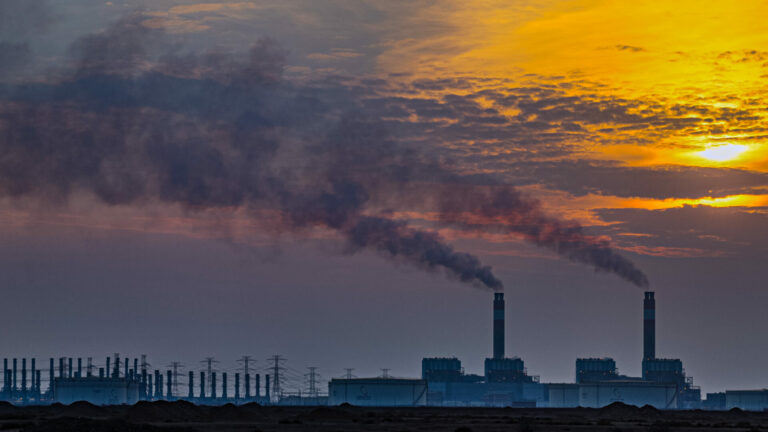
Bioscience