Statistics
Pulling rank on spatial statistics
A technique that uses the power of computing could solve statistical problems cheaper and faster than current methods.
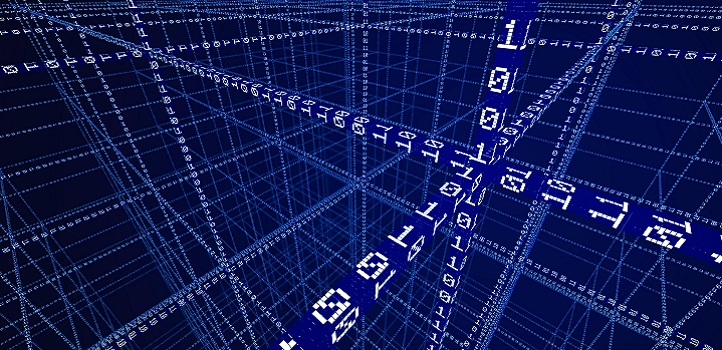
By applying the power of high-performance computing to one of the cornerstones of statistical methods, a technique developed by researchers from KAUST could analyze large datasets much more cheaply and quickly than current methods.
Spatial datasets can contain topographical, geometric or geographic information, such as environmental, climate or financial data, and comprise measurements taken across many locations and over long periods. The large size and high dimensionality of these datasets present significant statistical challenges for current statistical methods, which are unable to handle the computational burden and substantial cost—both increase rapidly as the size of the dataset grows—of analyzing such datasets.
These challenges led Marc Genton and David Keyes from KAUST, in collaboration with George Turkiyyah from the American University of Beirut in Lebanon, to develop a statistical method that exploits the hierarchical low-rank decomposition of covariance functions to significantly increase the speed of evaluating large-scale multivariate datasets with normal probabilities.
“Our aim was to be able to evaluate high-dimensional probabilities and do this faster than existing methods such that problems in statistics, which are currently intractable, become feasible,” explains Genton.
The efficient computation of multivariate normal distributed datasets, which contain correlated random variables that are grouped around a mean value, is important in many applications in statistics. However, as the dimensionality of such datasets increases, complex techniques like Monte Carlo simulations, which employ repeated random sampling and statistical analysis, must be used and can lead to computational inaccuracies at the tails of these datasets.
By exploiting the hierarchically low-rank nature of covariance matrices, in which the behavior of two random variables are related, the researchers were able to significantly reduce the computational burden, allowing them to tackle problems arising from large spatial datasets.
“The novelty of our approach arises from the collaboration between statistics and KAUST’s Extreme Computing Research Center because it allowed us to specifically bring the technology of hierarchical matrices to fundamental problems in statistical research,” says Genton.
The outcome is a practical one. The reduction in storage and arithmetic complexity required to perform matrix-vector operations, as well as factorization and inversion operations from quadratic or even cubic to log-linear, makes large classes of computations viable, class that would normally be prohibitively expensive.
“The significance of our findings lies in the fact that the problem we solved is the cornerstone of many methods in statistics and therefore opens up a whole new path of exciting research problems that were out of reach before,” says Genton.
References
-
Genton, M.G., Keyes, D.E. & Turkiyyah, G. Hierarchical decompositions for the computation of high-dimensional multivariate normal probabilities. Journal of Computational and Graphical Statistics 27, 268-277 (2017).| article
You might also like
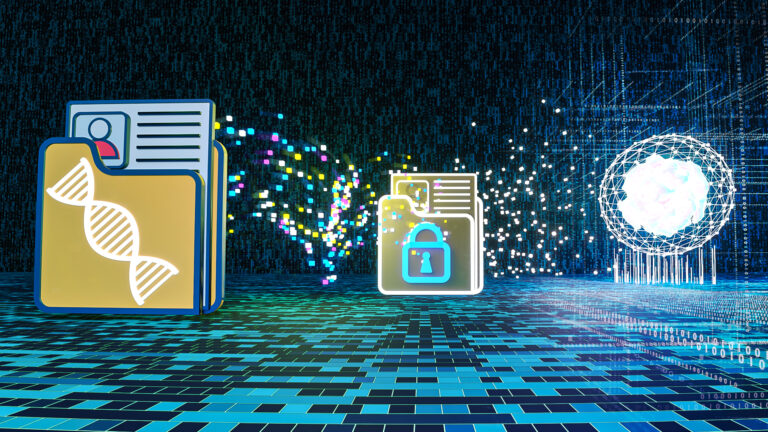
Bioengineering
Shuffling the deck for privacy
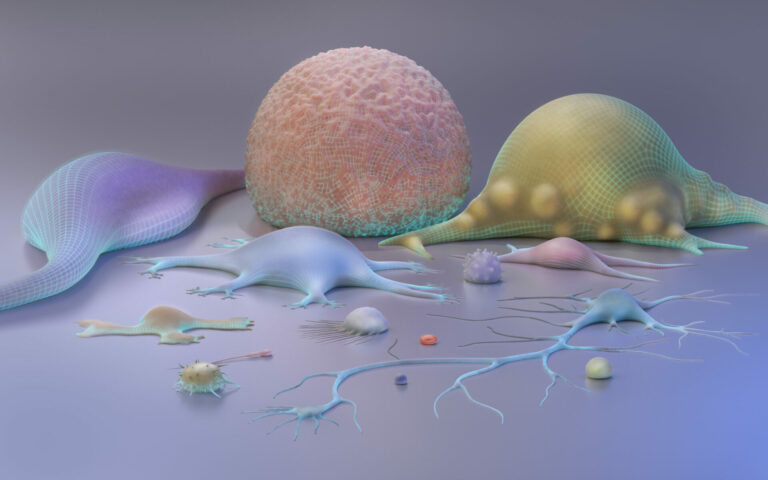
Bioengineering
AI for cells helps illuminate their identity
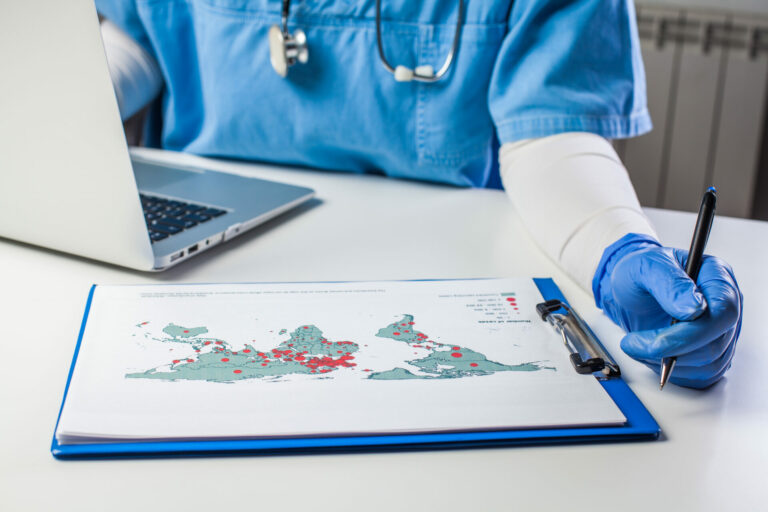
Applied Mathematics and Computational Sciences
Global look at sex differences in young people's mortality
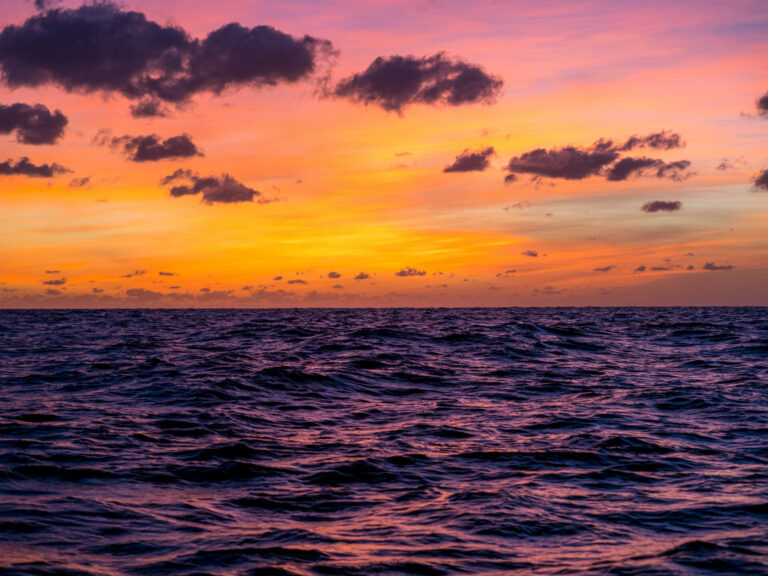
Applied Mathematics and Computational Sciences
Going likelihood-free with neural networks
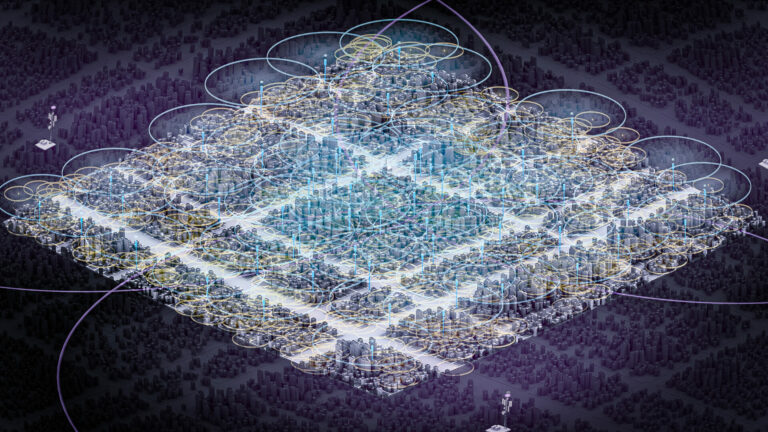
Applied Mathematics and Computational Sciences
A simple solution for frequency sharing
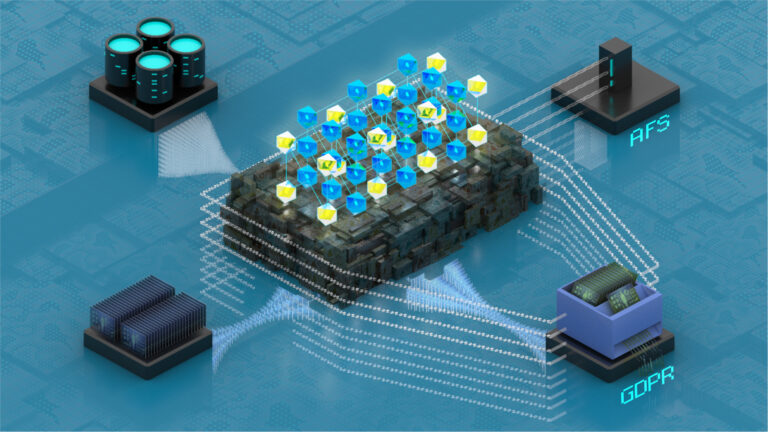
Bioengineering
Safeguarding the right to be forgotten
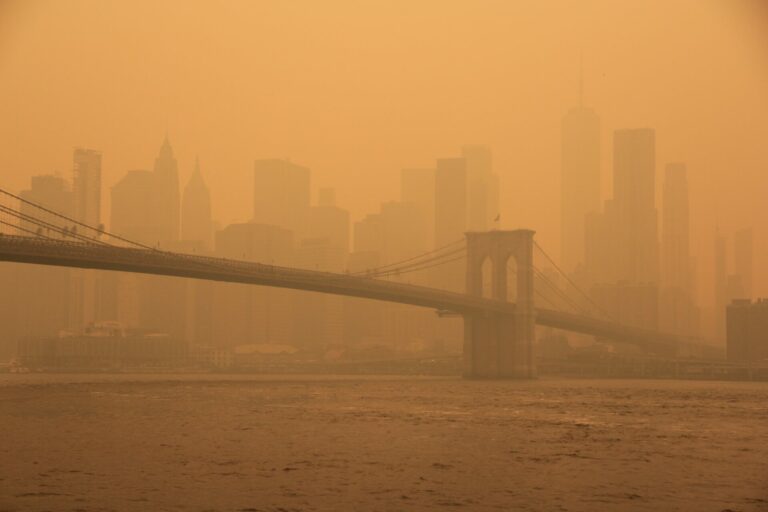
Applied Mathematics and Computational Sciences
Neural networks give deeper insights
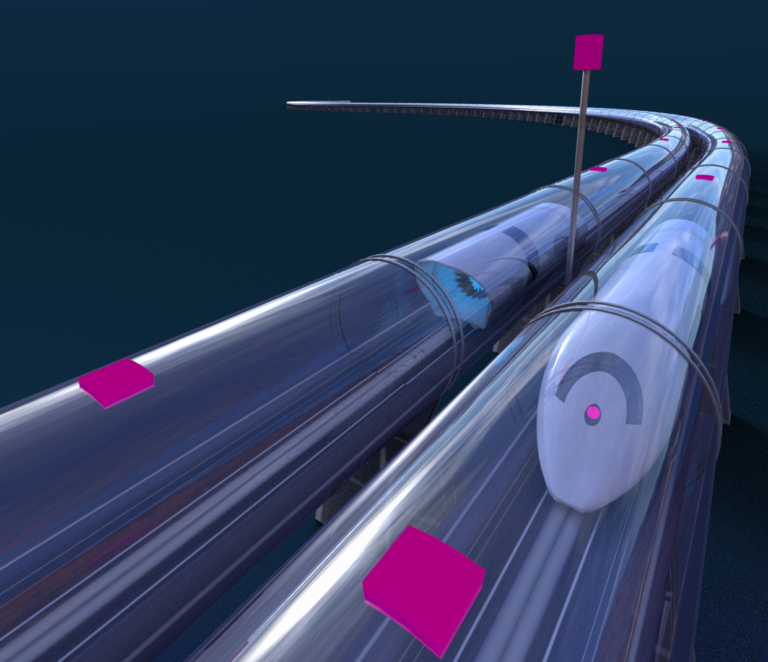
Applied Mathematics and Computational Sciences