Bioengineering
Finding patterns in big data
A statistical approach that can find patterns in large data sets, even in the event of missing or incorrect figures, is proving useful from genomic studies through to planning a marine reserve.
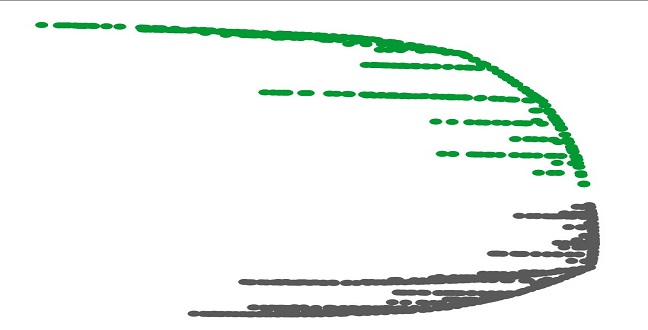
What do protecting endangered fish species and understanding human genetic data have in common? They both rely on statistical tools to find patterns in highly complex datasets. An international team, led by Tim Ravasi from KAUST, has found a way to identify such patterns1.
Genomics researchers often use a dimensionality reduction tool — principal component analysis (PCA) — to identify patterns. PCA looks at the Euclidian distance, the straight line, between any two data points, and plots it into a low dimensional space so that data are separated along only a few axis.
But, according to Ravasi from KAUST’s Biological and Environmental Science and Engineering Division, “PCA was not invented for genome wide datasets but for pattern recognition in data that are more linear. Biological systems are not always linear, data may be missing or contain mistakes, and if a sample is missing linearity then PCA fails.”
Ravasi’s team wanted to employ a tool that could deal with missing or wrong data and did not require the researcher’s prior knowledge to set parameters. His ideal was that “you only take data and the algorithm separates clusters.”
In addition to PCA, the team used a statistical method called non-centered minimum curvilinear embedding (nc-MCE). Instead of a straight line between two data points, the shortest path on a curve is computed which allows for a more fine-grained separation of the data.
The approach proved useful for a genetics application. Technologies such as DNA microarrays can rapidly probe tens of thousands of individual DNA bases so-called single nucleotide polymorphism (SNPs) that are known to differ between individuals. These data can shed light on the genetic differences between populations and predict the propensity for certain diseases — but only if they can be read correctly. A matrix of SNP data may have more than 50 thousand coordinates and so, to see similarities and differences, clusters need to be found.
Ravasi’s team showed that when applied to SNP data from African, European and two Asian populations, PCA was able to separate the individuals by continent, but nc-MCE could also distinguish between the Chinese and Japanese individuals. So while PCA can distinguish between geographical regions, nc-MCE allows a more detailed structuring and can pinpoint differences in ancestry. “The approach is universal” says Ravasi.
Another current application is a collaboration that uses ecological data to find patterns of habitats for different species of fish that live around the barrier reef adjacent to KAUST — the goal is to protect certain fish species by creating marine protected areas with ‘no fishing’ zones.
References
- Alanis-Lobato G., Cannistraci C.V., Eriksson A., Manica A. & Ravasi T. Highlighting nonlinear patterns in population genetics datasets. Scientific Reports 5, 8140 (2015).| article
You might also like
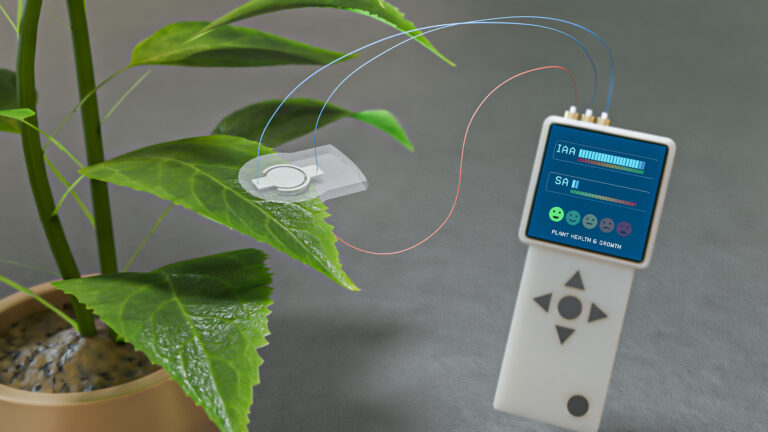
Bioengineering
Sensing stress to keep plants safe
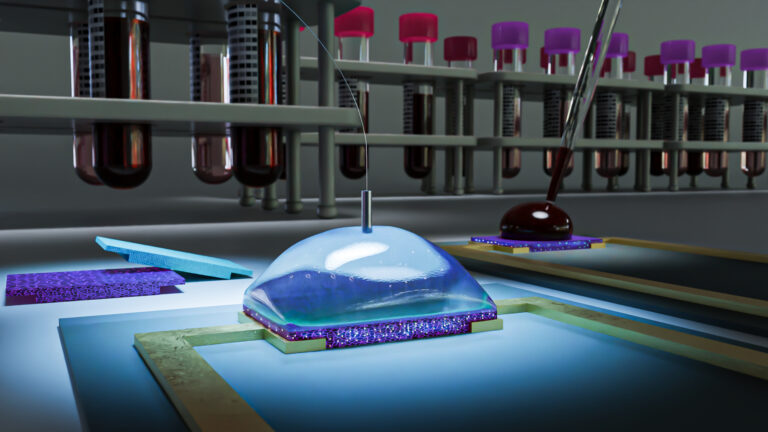
Bioengineering
Building better biosensors from the molecule up
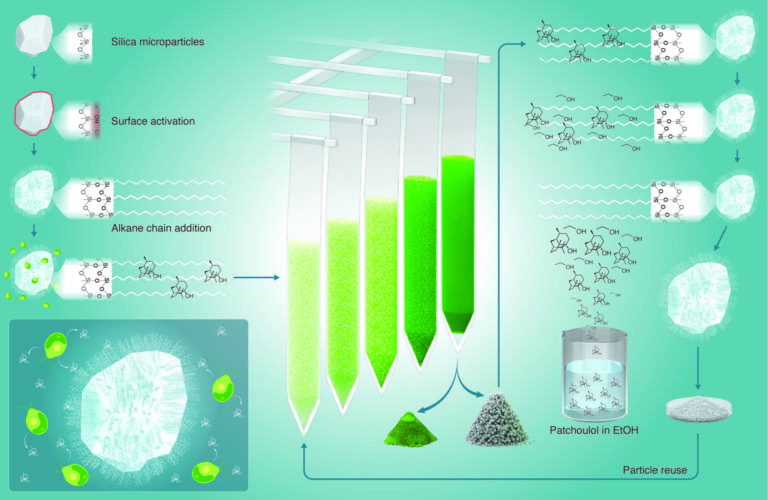
Bioengineering
High value harvests from designer algae
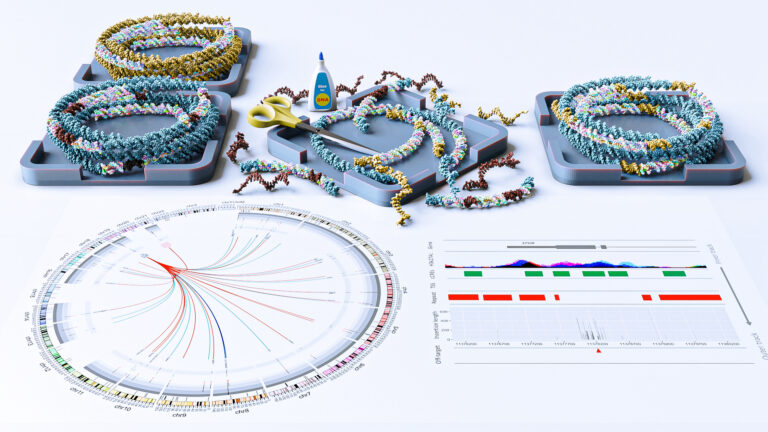
Bioengineering
Stray DNA fragments challenge CRISPR precision
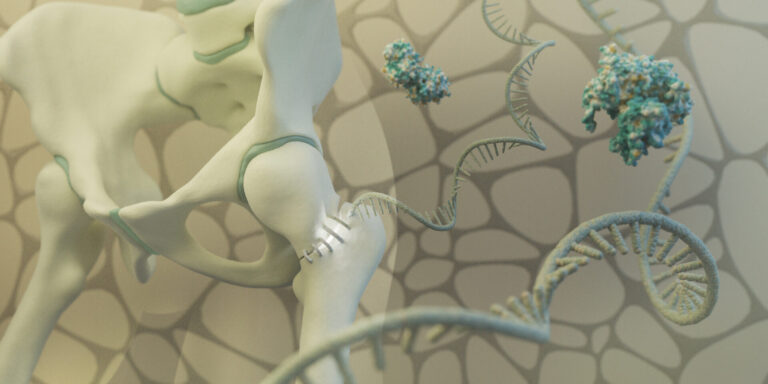
Bioengineering
Ancient RNAs kickstart bone repair
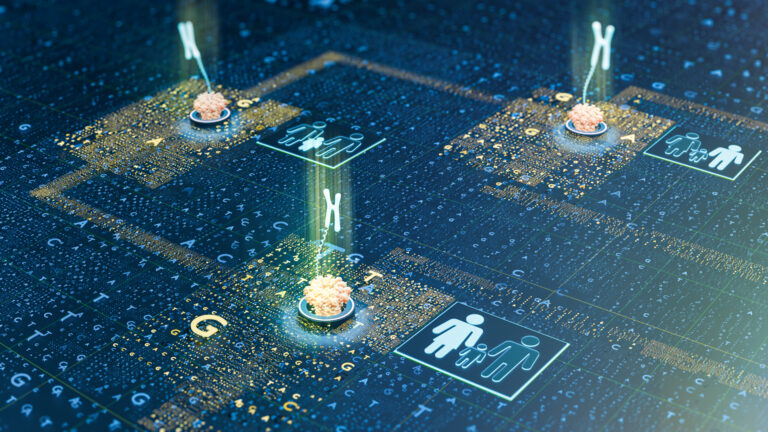
Bioengineering
Pioneering technique transforms genetic disorder diagnoses
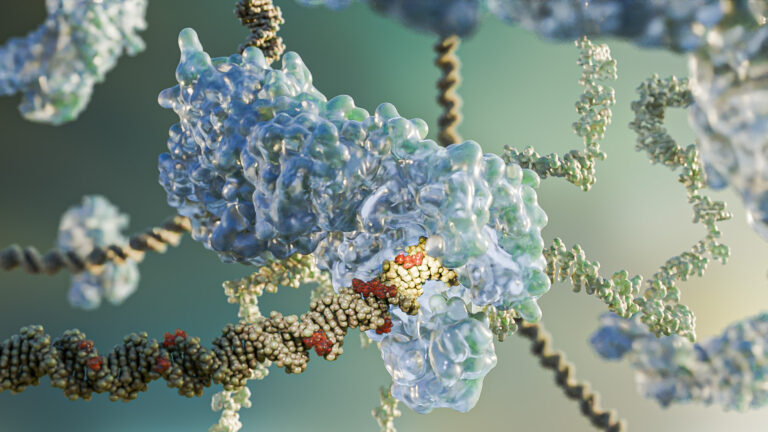
Bioengineering
Defeating the deletion makes genome editing safer and more precise
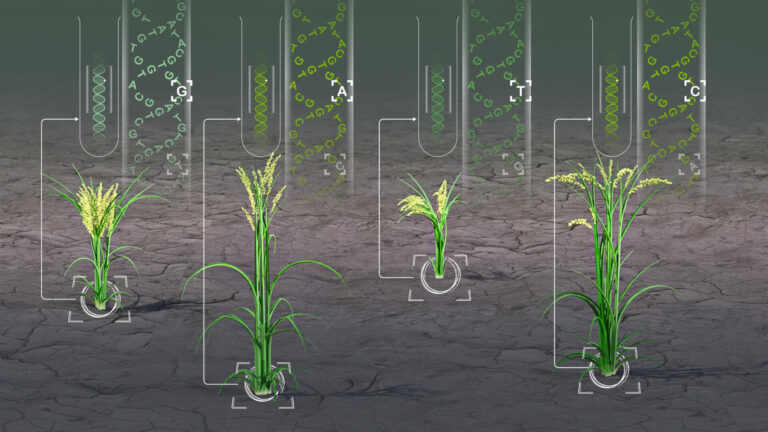
Bioengineering