Statistics
A sharper view of flood risk
Extreme weather patterns and regions at risk of flooding could be easier to spot using a new statistical model for large spatial datasets.
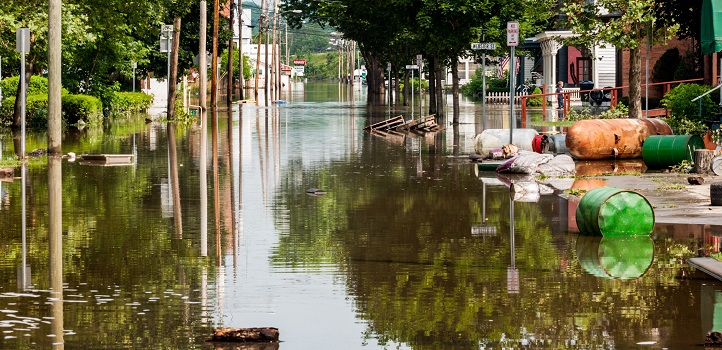
By generalizing a classical statistical model and adapting it for use in analyzing the extremes of rainfall in large datasets, researchers, including KAUST’s Raphaël Huser, have devised a more efficient and flexible analytical tool that promises to improve the prediction of flood risk and other extreme weather phenomena.
Rare extreme weather events, such as floods, extreme winds, high temperatures and drought, can be devastating, but predicting the frequency and severity of such conditions remains one of the key challenges in statistical science. Even large, long-term data sets over extensive areas may include very few extreme events, making it exceptionally difficult to predict future events with accuracy.
“There are classically two ways to model extreme events, the ‘block maximum’ approach, where we look at the largest events in blocks of time and the ‘threshold exceedance’ approach, which selects the top few percent of events across the entire timeframe of the dataset,” explains Huser, who undertook the work in collaboration with U.S.-based colleagues Gregory Bopp and Benjamin Shaby. “Previous work has developed new tools to apply the threshold exceedance approach; in this study we generalized a classical block maximum model for application to extreme precipitation.”
The block maximum approach has a long tradition in the statistics of extremes, but it has a high computational cost that limits its application to the large-scale datasets now routinely acquired in weather prediction. This approach is also unable to capture the observed weakening of the dependence between nearby conditions as events become more extreme.
The team’s approach addresses both of these shortcomings by adapting a relatively inflexible, but computationally efficient, max-stable model using Bayesian inference, which is a statistical estimation approach that provides a natural way of incorporating expert opinion and accounting for various sources of variability.
“Our Bayesian model has a lot of parameters and hidden random effects, which need to be jointly estimated,” says Huser. “Beyond the computational challenge, simply developing the model itself and deriving its theoretical properties was a major challenge. There is a reason why classical max-stable models have been used extensively for a long time—it is not straightforward to generalize them and come up with more realistic and flexible models.”
The model was able to capture the observed patterns in extreme precipitation events occurring along the coast and mountain range borders in northeast America, demonstrating its potential for predicting flood risk.
“Our model could also easily be adapted to other types of environmental datasets, such as wind and temperature, giving it very wide applicability,” notes Huser.
References
- Bopp, G., Shaby, B. & Huser, R. A hierarchical max-infinitely divisible spatial model for extreme precipitation. Journal of the American Statistical Association advance online version 2 April 2020. | article
You might also like
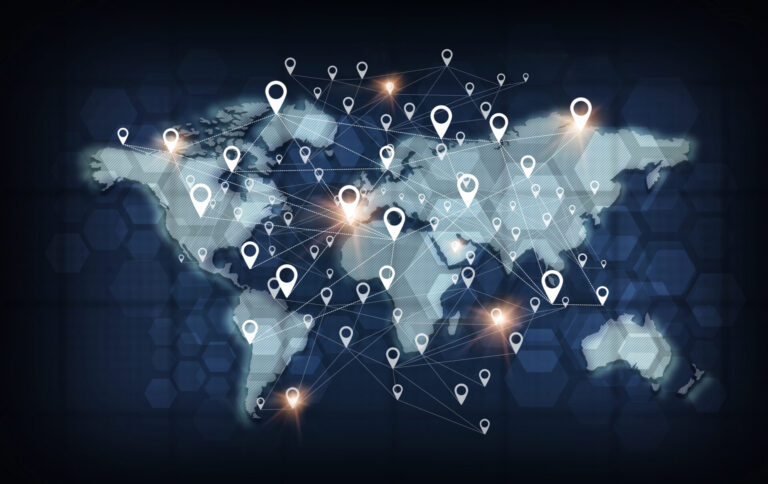
Statistics
Joining the dots for better health surveillance
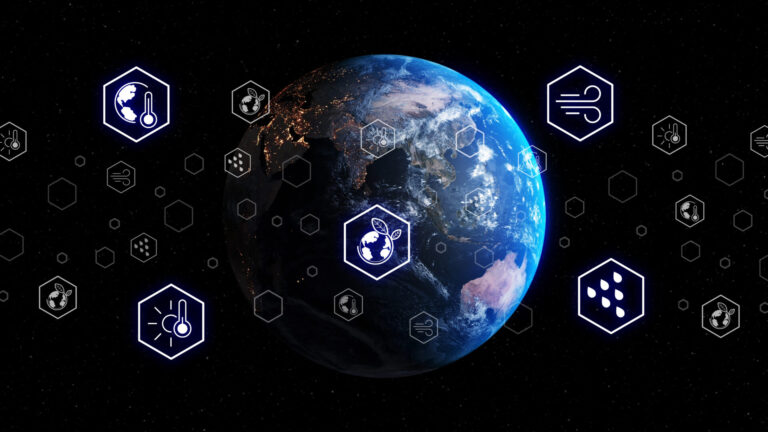
Statistics
Easing the generation and storage of climate data
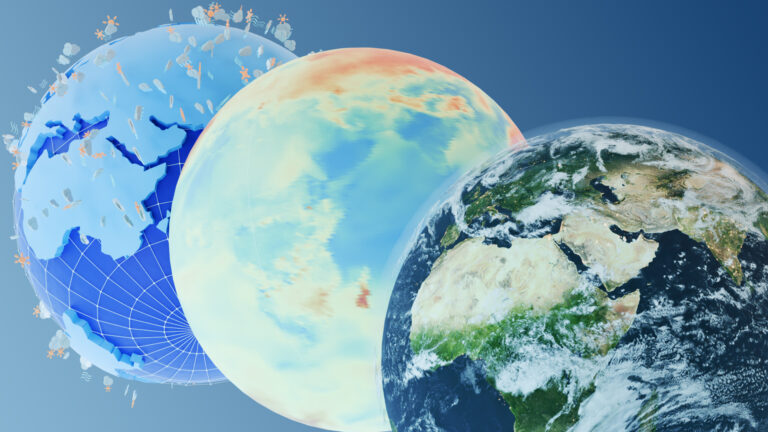
Statistics
A high-resolution boost for global climate modeling
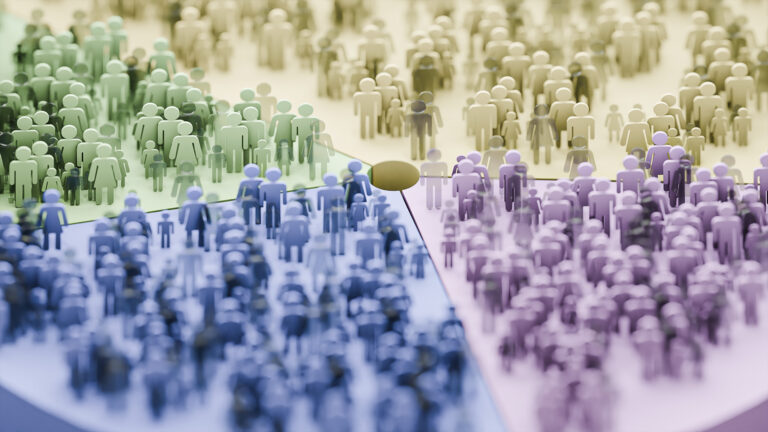
Applied Mathematics and Computational Sciences
Finer forecasting to improve public health planning
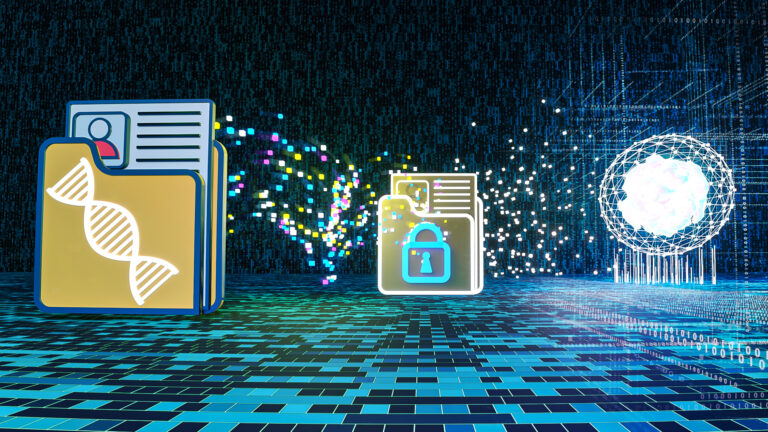
Bioengineering
Shuffling the deck for privacy
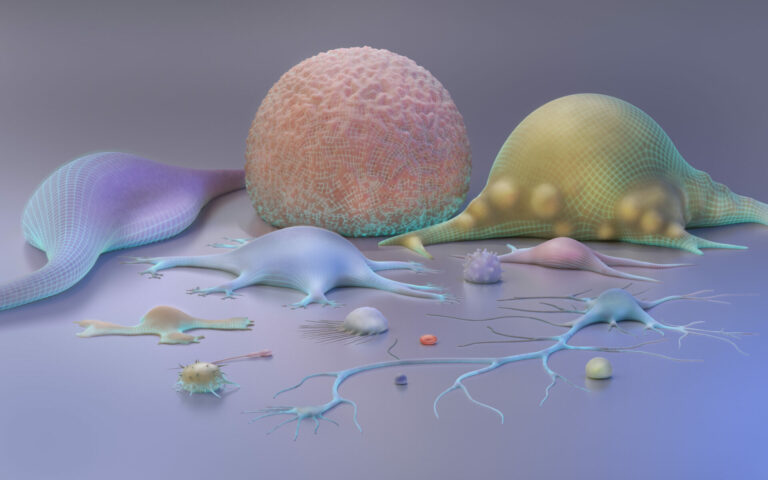
Bioengineering
AI for cells helps illuminate their identity
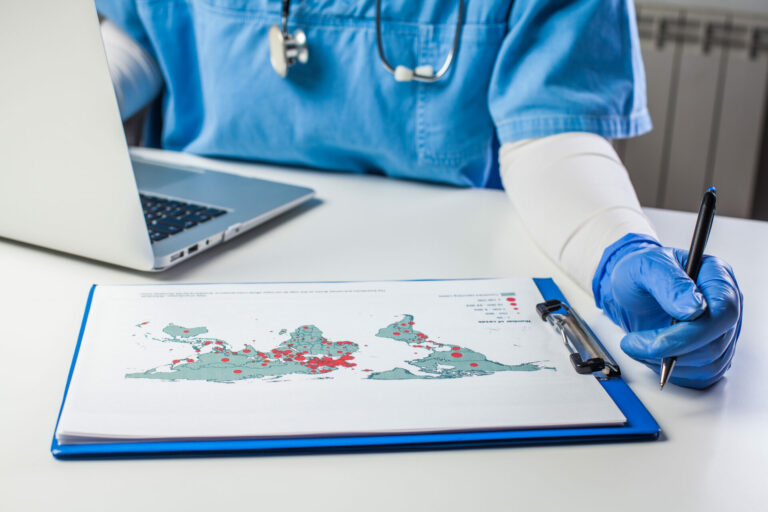
Applied Mathematics and Computational Sciences
Global look at sex differences in young people's mortality
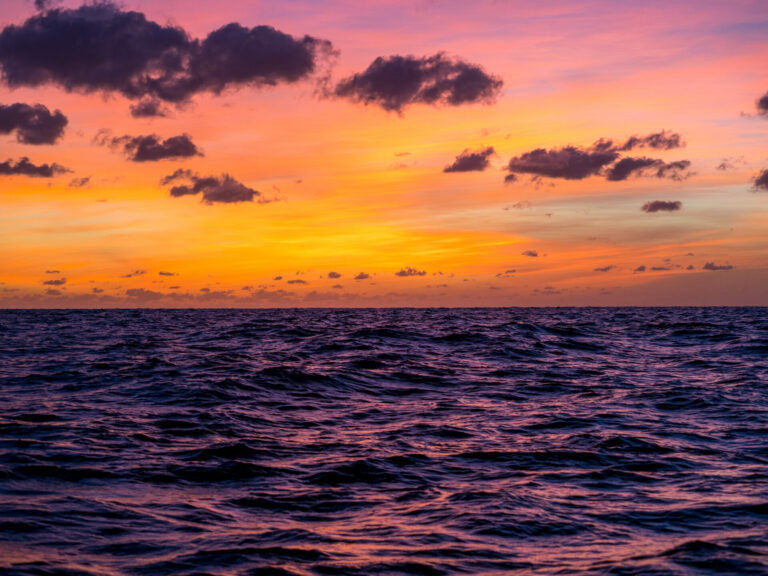
Applied Mathematics and Computational Sciences