Applied Mathematics and Computational Sciences | Statistics
Finer forecasting to improve public health planning
A method for forecasting high-dimensional functional time series could improve the accuracy of mortality predictions across multiple populations.
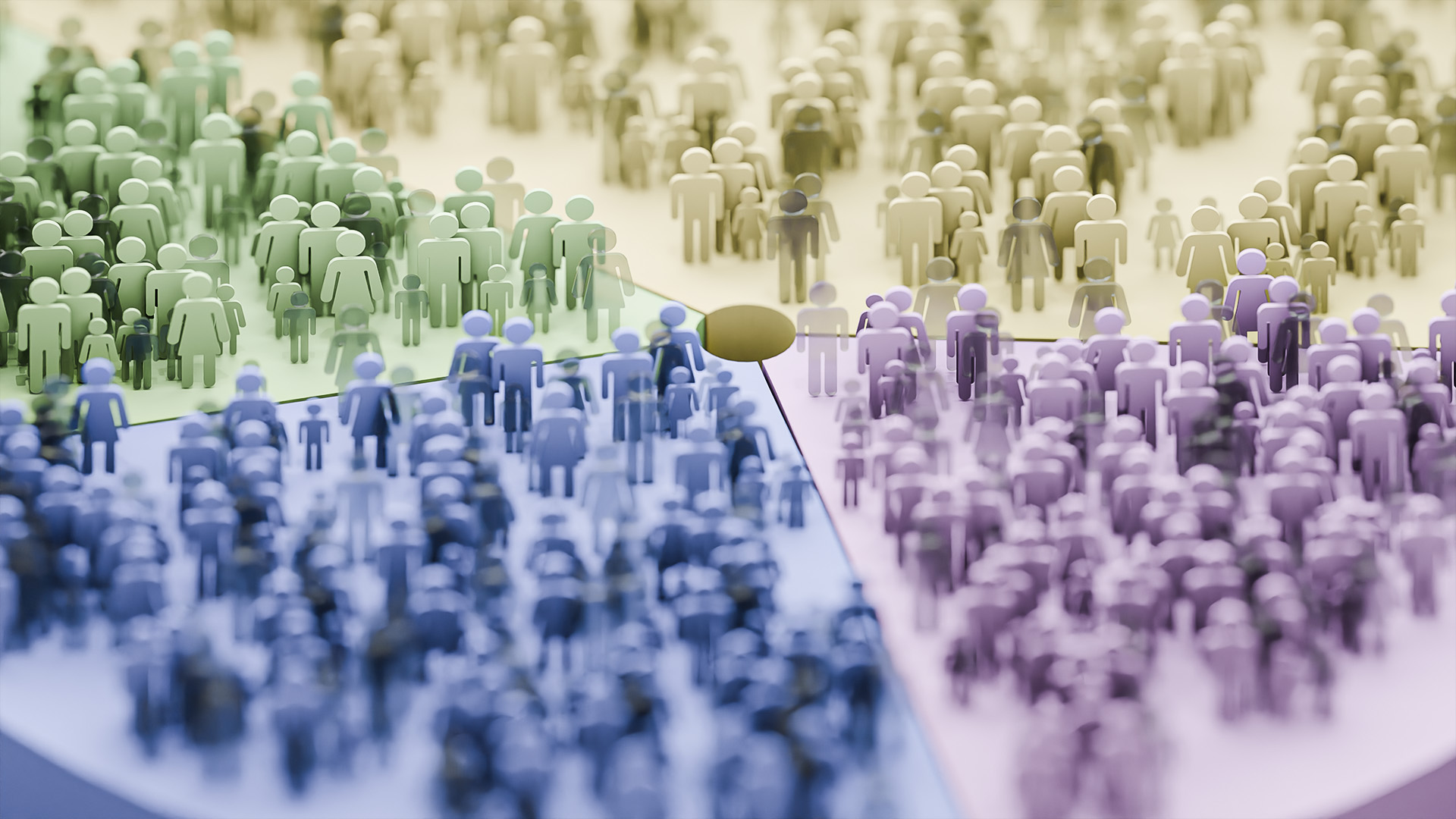
Applying a statistical approach, KAUST researchers have developed a model that improves mortality forecasting and can be applied to population sub-groups, based on factors such as age and gender, among others.
In most countries around the world, current drops in mortality rates are associated with aging populations. Governments and other policymakers require accurate forecasting for planning and decision-making. In the field of mortality forecasting, for example, precise predictions can inform policy decisions, insurance premiums and public health strategies. Researchers have developed a method for forecasting high-dimensional functional time series (HDFTS) that significantly improves the accuracy of mortality predictions[1].
Mortality forecasting is a challenging task that often produces suboptimal outcomes when forecasts focus on single populations. Populations can be further categorized based on gender, state, ethnic group, socioeconomic status and other factors. KAUST researchers Cristian Jiménez-Varón and statistician Ying Sun, in collaboration with HanLin Shang from Macquarie University in Australia, have addressed this issue by using a novel approach to model mortality for several populations simultaneously.
Their method involves breaking down HDFTS into two main components: a deterministic component (such as population or state) that remains constant over time and a functional residual component that varies over time. The breakdown is achieved through the estimation of a two-way functional analysis of variance (ANOVA) model, incorporating factors such as states and genders. The final mortality forecasts are derived by combining the deterministic component with the forecasted residuals.
Sun highlights the potential transformative impact of this research. “Our method has the potential to advance mortality forecasting by enabling more precise predictions. These predictions can inform various decisions, ranging from public health strategies to insurance premium calculations,” she says.
They applied their forecasting method to age- and sex-specific mortality data from different states in the United States, departments in France, and prefectures in Japan. By incorporating information on serial dependence and correlation across multiple populations, the proposed method produces more accurate mortality forecasts than other methodologies reported in recent literature.
According to lead author Jiménez-Varón, the team’s approach is significant as it not only enhances the accuracy of point forecasts but also ensures the reliability of interval forecasts. “This improvement enables more nuanced and detailed predictions of mortality, contributing to a more comprehensive understanding of the subject,” he says.
“Our proposed methodology could also be applied to other areas, such as university performance completion rates which can be disaggregated by age, gender, faculty, local or international status, and other criteria.”
Reference
- Jiménez-Varón, Cristian F., Sun, Y. & Shang, H-L. Forecasting high-dimensional functional time series: Application to sub-national age-specific mortality. Journal of Computational and Graphical Statistics, published 19 March 2024.| article
You might also like
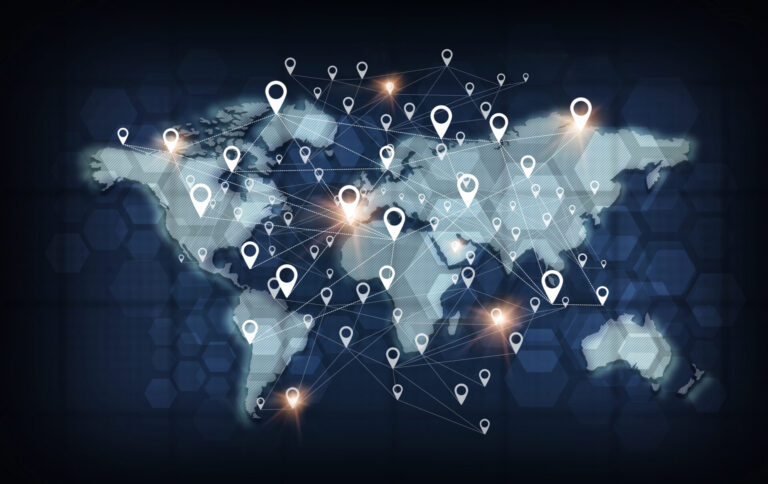
Statistics
Joining the dots for better health surveillance
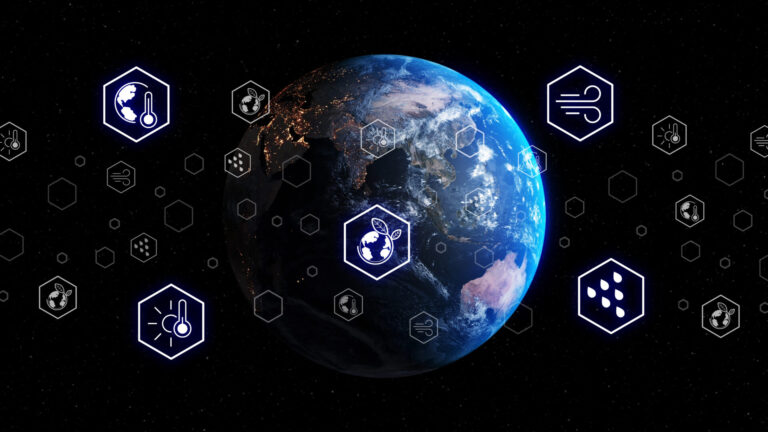
Statistics
Easing the generation and storage of climate data
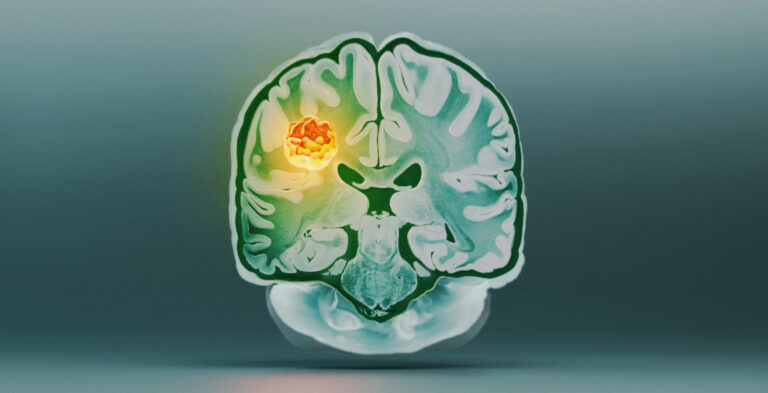
Applied Mathematics and Computational Sciences
Bringing an old proof to modern problems
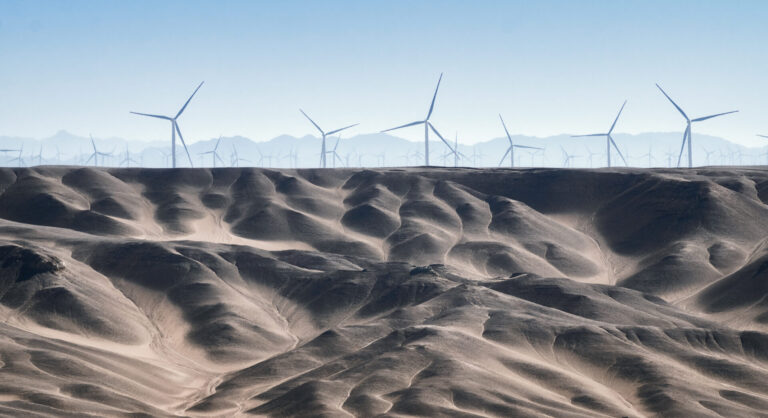
Applied Mathematics and Computational Sciences
Accounting for extreme weather to boost energy system reliability
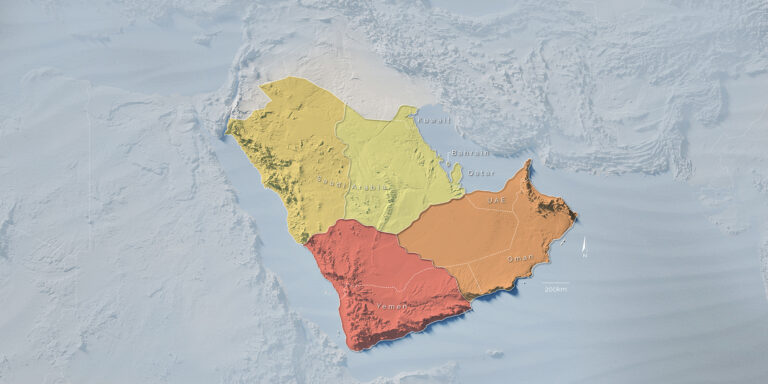
Applied Mathematics and Computational Sciences
Past and future drought patterns across the Arabian Peninsula
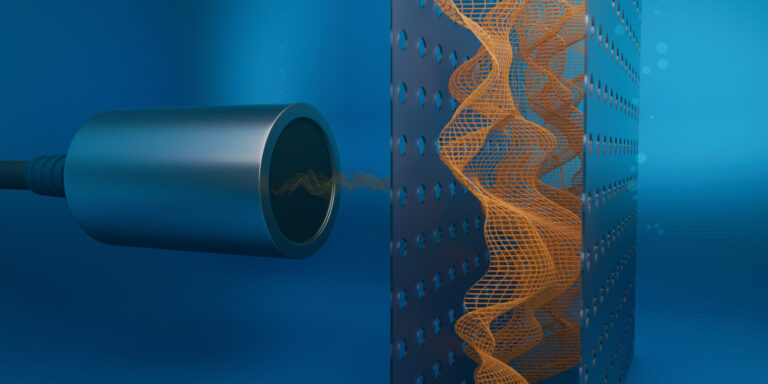
Applied Mathematics and Computational Sciences
New pattern for underwater resonators
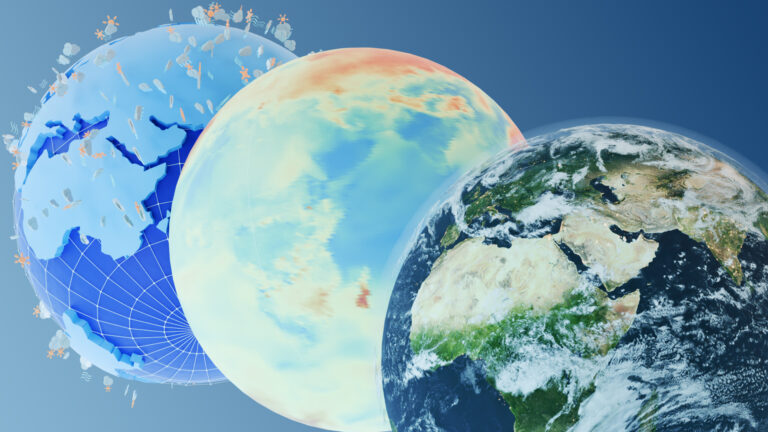
Statistics
A high-resolution boost for global climate modeling
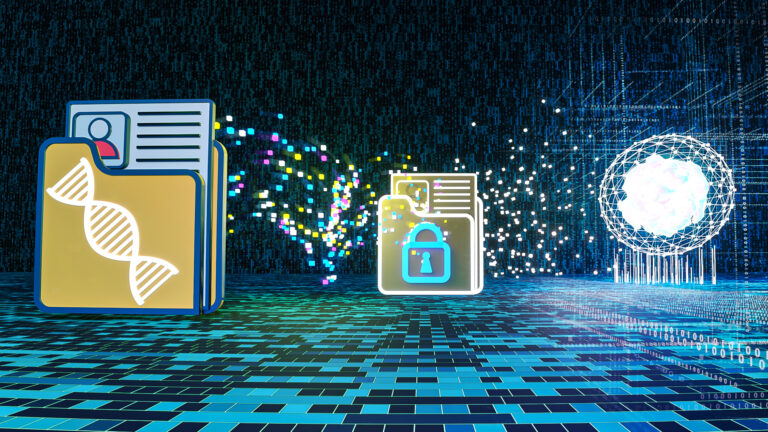
Bioengineering