Computer Science
Peeling back the layers of deep machine learning
A layer-based approach raises the efficiency of training artificial intelligence models.
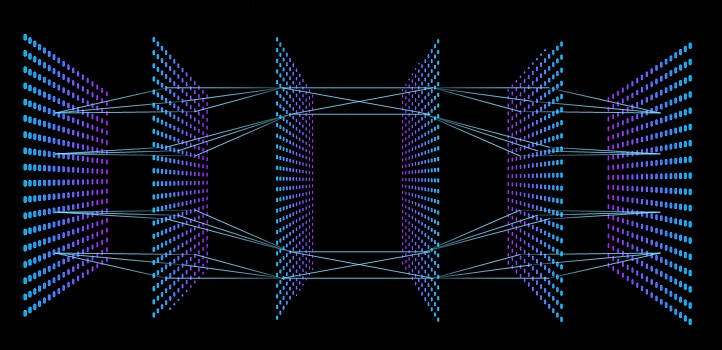
Diving into the mechanics of how deep neural networks (DNNs) are trained in machine learning, KAUST researchers have clarified a key discrepancy between the theory and practice of training on distributed computing platforms.
As artificial intelligence algorithms become more sophisticated and capable in their decision-making ability, the DNNs at their heart become increasingly onerous to train. Training DNNs requires enormous volumes of data and is a computationally intensive task that often relies on parallel processing across many distributed computers. However, this type of distributed computing demands a huge amount of data transfer between the parallel processes, and the actual speed up remains far from optimal.
Making distributed deep learning more efficient by reducing the amount of data that needs to be shared among computational nodes is one of the hottest topics in machine learning. Aritra Dutta, Panos Kalnis and their colleagues in the Extreme Computing Research Center have been working at the interface between distributed systems and machine learning to tackle just such problems.

Layer-by-layer computation and compression (left) of the deep neural network on distributed computing systems can be faster than compression after global computation (right).
© Dutta et al. 2020
“We have been working on implementing a uniform framework for compression methods for distributed deep learning for some time,” says Dutta. “However, there are daunting challenges to implement such a framework. The first step in this research has been to close a fundamental knowledge gap between theory and practice; that existing compressed communication methods assume that the compression operators are applied to the entire model, when popular distributed machine learning toolkits perform compression layer by layer.”
DNNs, like all neural networks, are composed of “layers” of neuron-like switches that turn on or off in response to an input. The simplest and shallowest of neural networks comprise only an input and an output layer and perform simple classification and decision tasks. DNNs have many hidden layers of neurons that each have slightly different sensitivities, and the ability for the DNN to discriminate and classify inputs, such as faces or object types, improves as labeled data is put through the network.
The point made by the researchers in their paper is that existing compression methods assume information is shared among distributed computing nodes after each pass of the full neural net. However, in practice, information is passed among computing nodes after each layer is processed, which is actually faster.
“Running distributed machine learning training jobs is routine industrial practice. Our work shows that practitioners should, in most cases, adopt layer-by-layer compression techniques to achieve faster training,” says Dutta.
References
- Dutta, A., Bergou, E.H., Abdelmoniem, A.M., Ho, C.-Y., Sahu, A.N., Canini, M. & Kalnis, P. On the discrepancy between the theoretical analysis and practical implementations of compressed communication for distributed deep learning. Proceedings of Thirty-Fourth AAAI Conference on Artificial Intelligence (2020).| article
You might also like
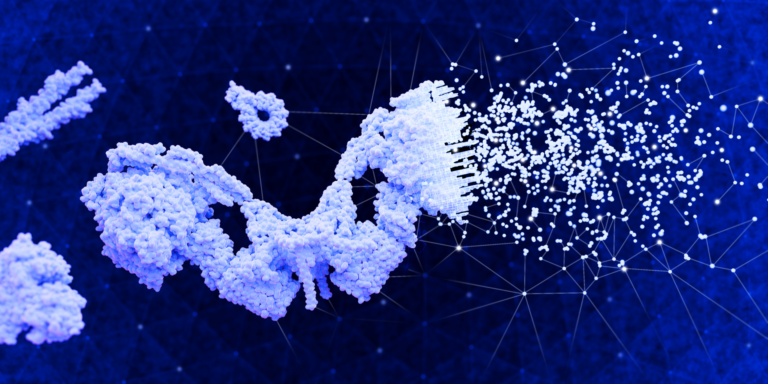
Bioengineering
AI tool predicts function of unknown proteins
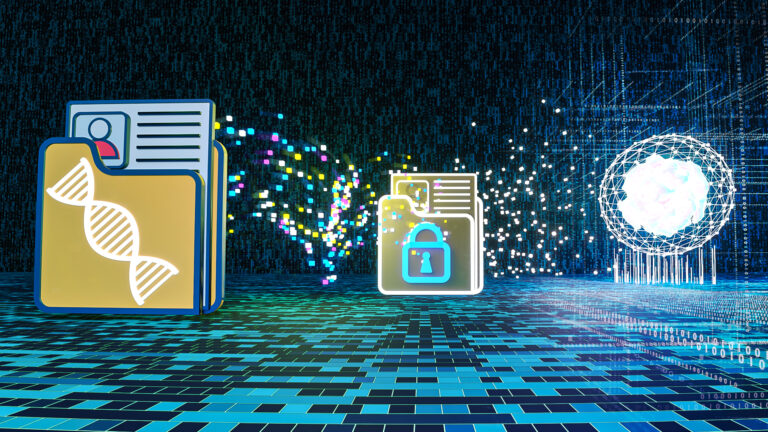
Bioengineering
Shuffling the deck for privacy
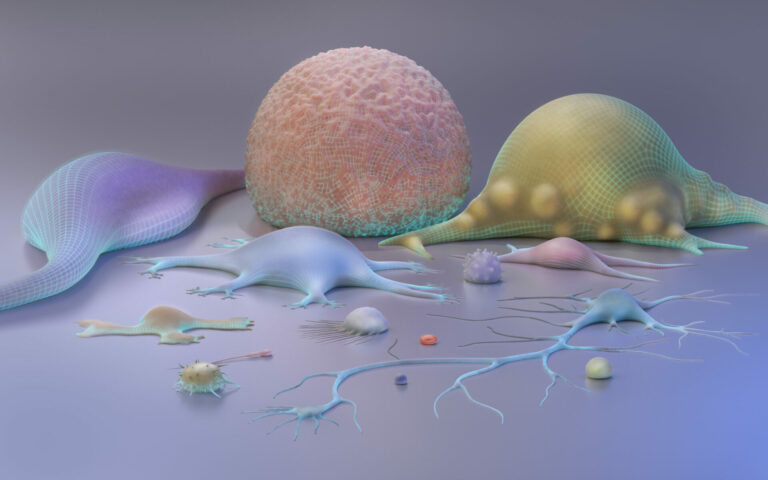
Bioengineering
AI for cells helps illuminate their identity
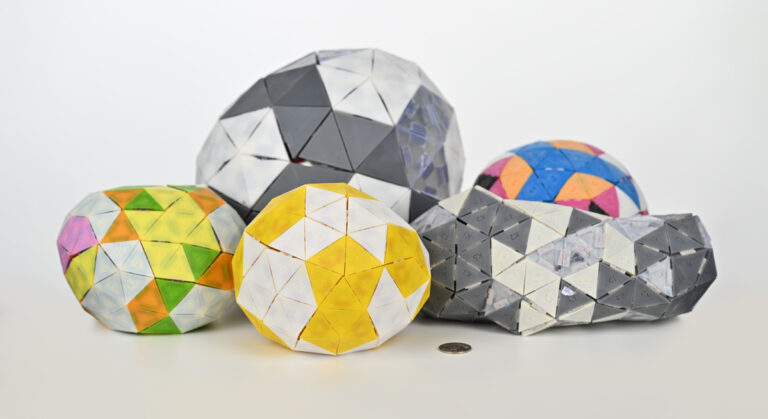
Computer Science
Dr. KID turns biological models into fun puzzles
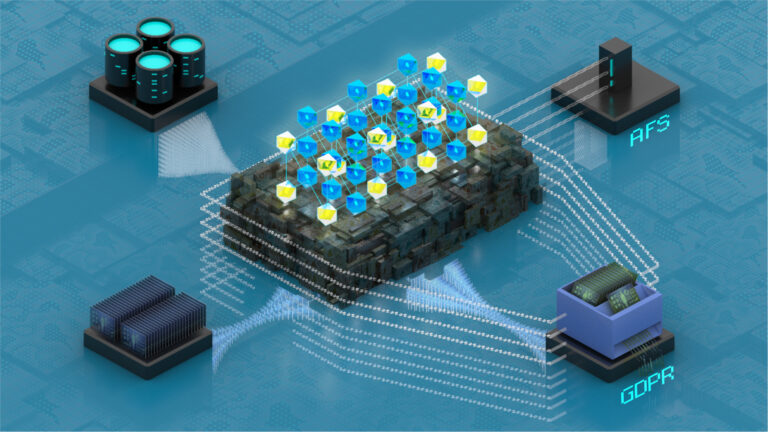
Bioengineering
Safeguarding the right to be forgotten
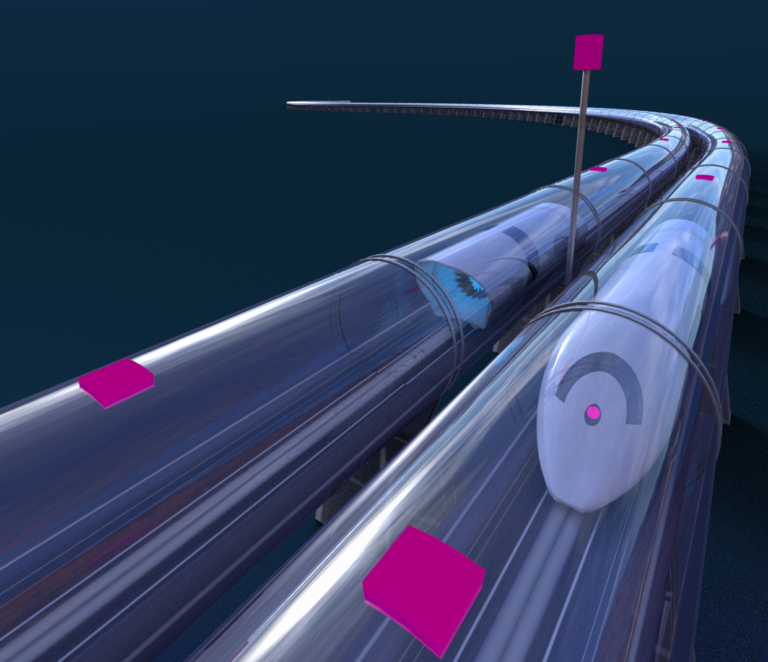
Applied Mathematics and Computational Sciences
Staying online in the high-speed tube of the future
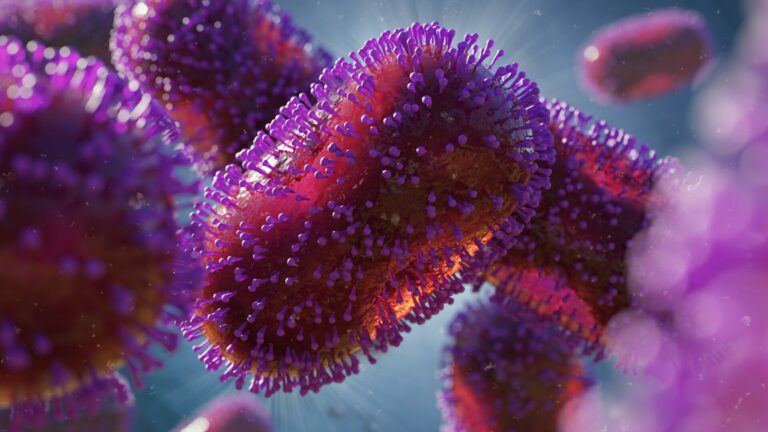
Bioengineering
Lab-grown skin helps unlock secrets of mpox virus infection
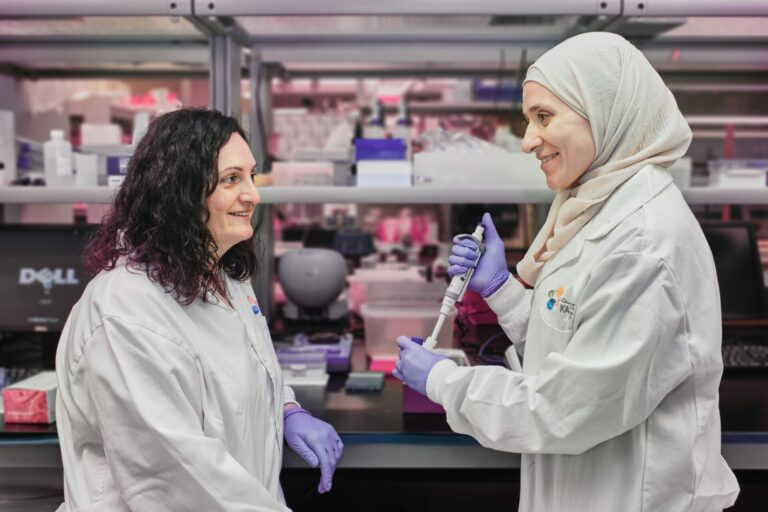
Bioengineering