Computer Science
Algorithm turns cancer gene discovery on its head
Prediction method could help personalize cancer treatments and reveal new drug targets.
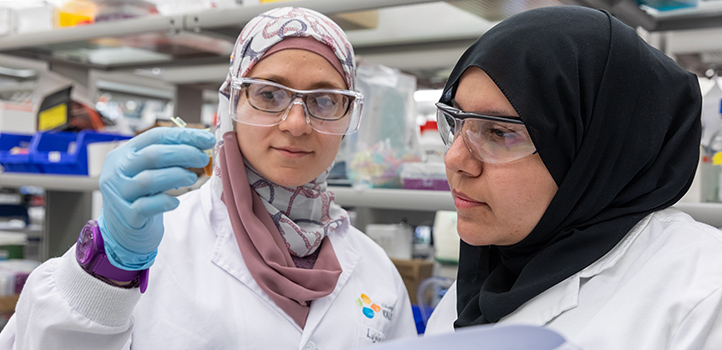
Prediction method could help personalize cancer treatments and reveal new drug targets.
A method for finding genes that spur tumor growth takes advantage of machine learning algorithms to sift through reams of molecular data collected from studies of cancer cell lines, mouse models and human patients.
By teaching the artificial intelligence system to link certain DNA mutations to altered functionality, a team led by Robert Hoehndorf from KAUST’s Computational Bioscience Research Center showed that they could identify genes with a known causative role in cancer and pick out dozens of putative new ones for 20 different tumor types.
The prediction method—described in Scientific Reports and freely available online—could help clinicians tailor medicines to the molecular subtypes of patients. It could also be used by drug companies in the hunt for new therapeutic targets.
“Our method can be used as a framework to predict and validate cancer-driver genes in any database or real population sample,” says first author of the study, Sara Althubaiti, a Ph.D. student in Hoehndorf’s lab.
Traditionally, scientists have approached the search for genes with a causal role in cancer by starting with DNA sequence data. By extensively cataloging tumor mutations shared among patients with a common type of cancer, the research community has documented hundreds of genes with a causal impact on tumor development. Experimental follow up is then used to functionally associate these genes with the hallmarks of cancer.
“Our method turns this approach on its head,” Althubaiti explains. “Essentially, our approach is knowledge-driven and we use tumor sequencing data as validation. This is unlike most approaches, which are data-driven combined with interpretation of the findings with respect to established knowledge.”
The rate of discovery for new cancer-driving genes has been declining rapidly in recent years, leading the team to seek a new computational strategy. Instead of relying on sequence data, Althubaiti and Hoehndorf built a machine learning model that takes into account many biological features of genes and pathways involved in tumor formation.

Robert Hoehndorf (left) and Sara Althubaiti discuss the performance of their algorithm.
© 2020 KAUST
The researchers designed the algorithm to recognize functional and phenotypic patterns that predispose a gene toward playing a role in driving tumor development. They validated the model using a publicly available database of some 27,000 different tumor variants as well as functional and sequence data—showing that the algorithm could accurately categorize known cancer-driving genes and detect more than 100 other likely culprits, many with specific roles in particular tumor types.
The KAUST investigators then further tested the algorithm’s performance on molecular data gathered from two cohorts of cancer patients. The first was from King Abdulaziz University Hospital in Saudi Arabia, comprising 26 tumor samples from individuals with a rare type of head and neck cancer called nasopharyngeal carcinoma. The other cohort comprised 114 colorectal cancer samples from patients treated at the University of Birmingham Hospital in the United Kingdom. In both patient groups, the model singled out candidate driver genes that were frequently mutated and shared pathogenic features of other cancer-causing genes.
Hoehndorf emphasizes the importance of the team effort involved. “This work is a good example for scientific collaboration within Saudi Arabia,” he says, “but it also demonstrates the need for multidisciplinary collaborations between computer scientists, clinical researchers and biologists.”
References
- Althubaiti, S., Karwath, A., Dallol, A. Noor, A., Alkhayyat, S.S., Alwassia, R., Mineta, K., Gojobori, T., Beggs, A.D., Schofield, P.N., Gkoutos, G.V. & Hoehndorf, R. Ontology-based prediction of cancer driver genes. Scientific Reports 9, 17405 (2019).| article
You might also like
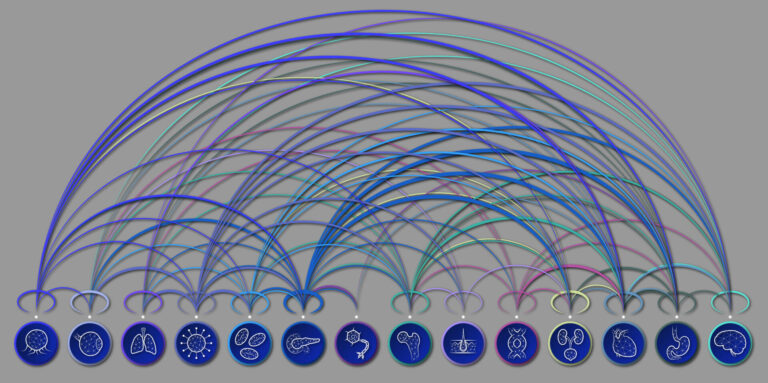
Computer Science
AI tool maps hidden links between diseases
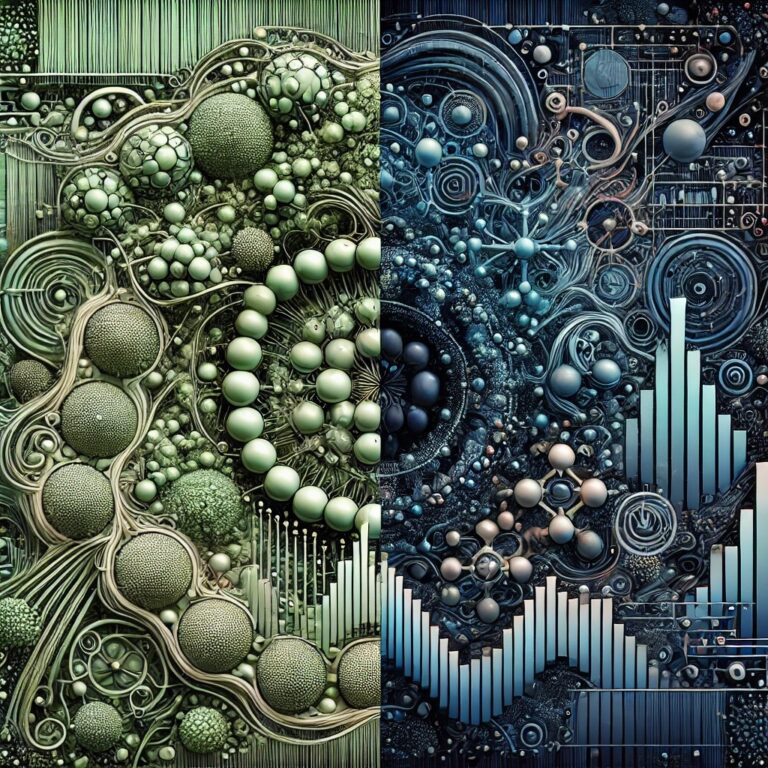
Bioscience
The theory of everything that wasn’t
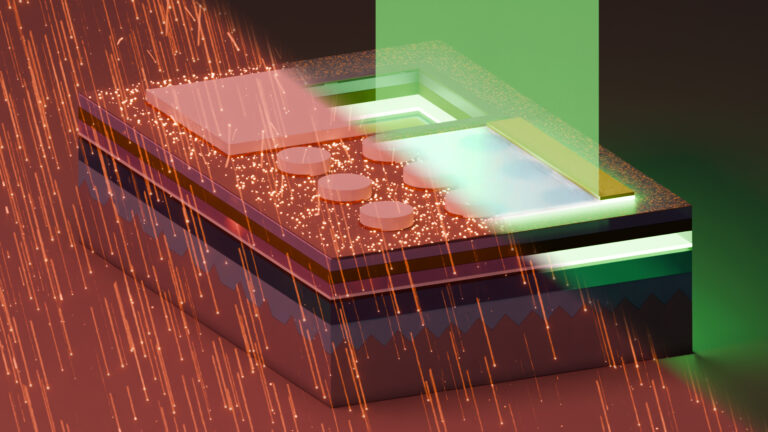
Computer Science
A new path to high-efficiency micro-LEDs
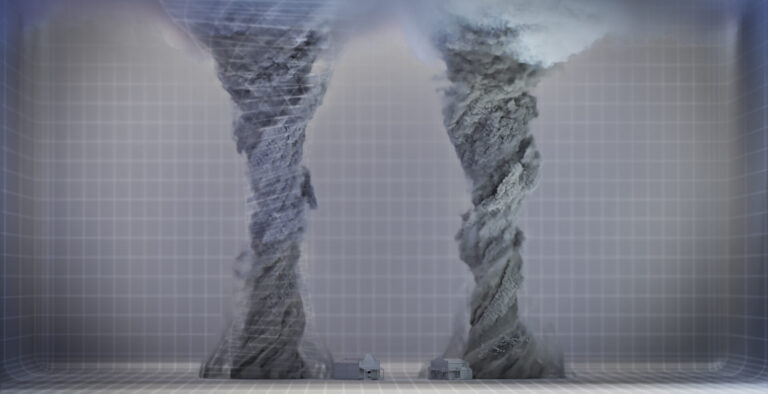
Computer Science
Cyclones meet their mathematical match
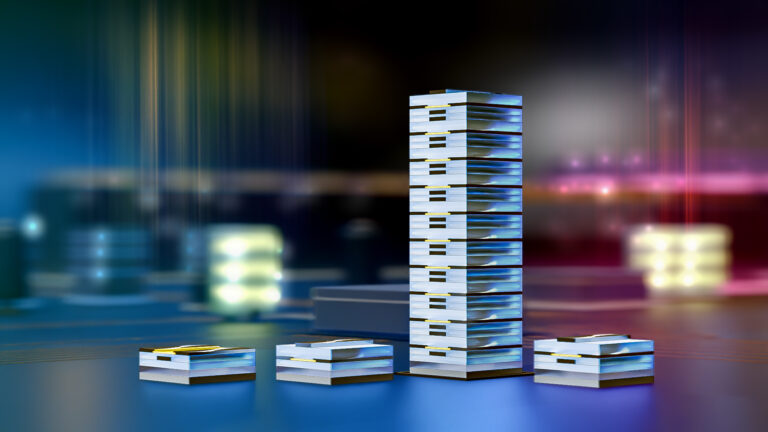
Applied Physics
Onward and upward to smaller faster devices
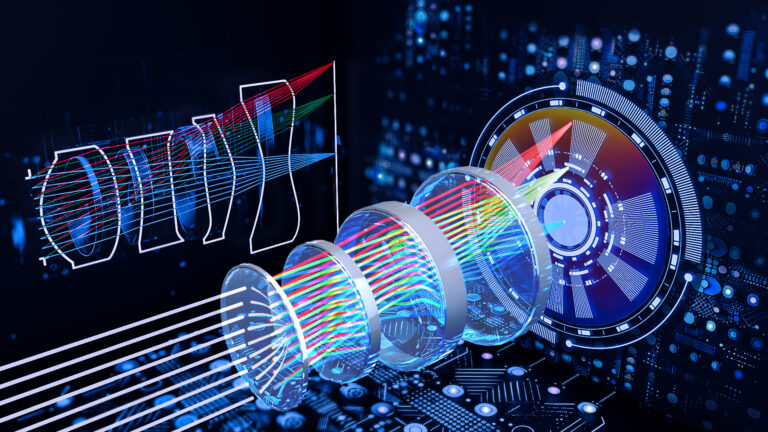
Computer Science
Deep learning improves lens design
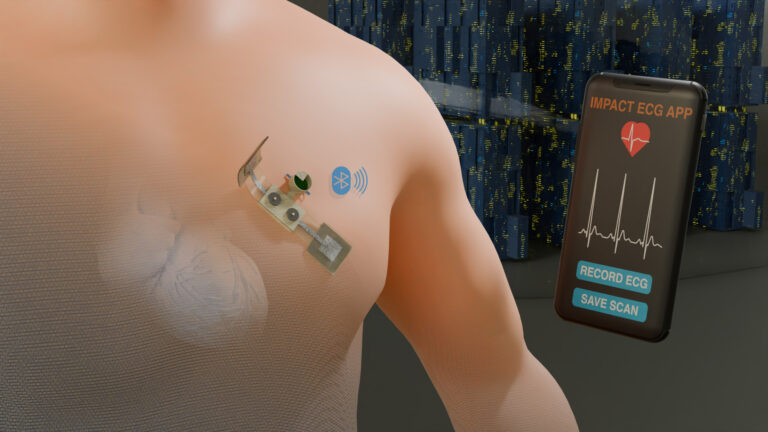
Computer Science
Better connection for monitoring helps heart patients and healthcare staff
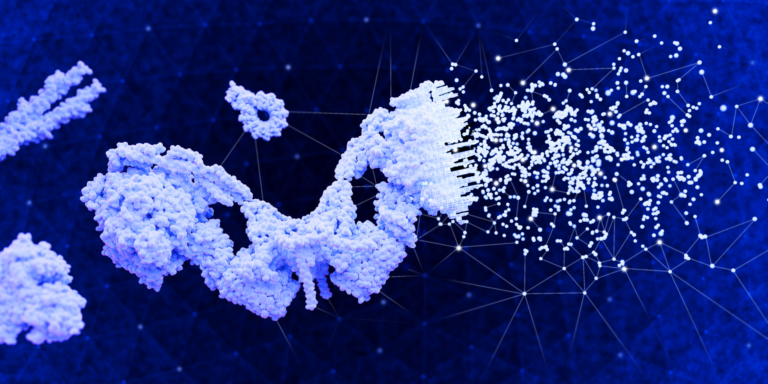
Bioengineering