Computer Science
Computer learns to write captions with feeling
Teaching AI to capture the full spectrum of human emotions when annotating artwork.
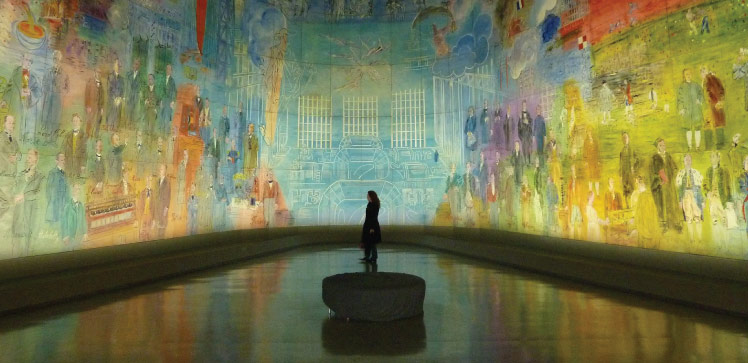
Giving artificial intelligence (AI) a more balanced perspective of artwork during training can reduce emotional bias in computer-generated captions.
Despite incredible advances in AI, it remains a challenge to replicate the human emotional response to sensory stimuli such as sights and sounds. For example, in image captioning, an AI processes visual information and associated language to generate a natural-sounding description of the picture. But art often triggers unique feelings in the beholder that AI can’t experience, such as calm, joy, awe or fear. Researchers are turning to affective captioning to bridge the gap between human and machine emotional intelligence.
“Affective captioning goes beyond factual descriptions to the subjective experience and the emotions an image evokes,” says KAUST researcher Mohamed Elhoseiny. One obstacle to reliable affective captioning is the introduction of biases at the data collection phase. “Biases are integral to human evolution,” says colleague Youssef Mohamed. “As a result, AI training datasets annotated by humans are intrinsically biased.”
“Interestingly, the contrastive approach caused people to pay more attention to the fine details in the art,”
Unlike humans, machines cannot detect or scrutinize biases, so biased data simply translates into biased decisions. Elhoseiny’s team observed this effect in ArtEmis, a popular AI training dataset of image descriptions, of which 62 percent contained positive emotions while only 26 percent had negative, leading to AIs that generated most of their captions with a positive sentiment.
To tackle this, the researchers created a new dataset of more than 260,000 image descriptions, collected using a contrasting approach to balance the bias. People were presented with a painting, as well as a set of 24 very similar paintings from which they had to select the one that they thought most closely resembled the original while eliciting the opposite emotion. In eight or more words they had to explain why.
Mohamed and his colleagues then combined the two datasets and used them to train a neural speaker — a type of deep-learning model — to generate affective captions. Neural speakers trained on the new dataset created captions with a 20 percent closer agreement to human-written captions than those generated by models trained using ArtEmis alone. “Interestingly, the contrastive approach caused people to pay more attention to the fine details in the art,” says Mohamed, “which resulted in much better captions and a wider range of emotions.”
Creating AIs that can understand human emotions will help build social acceptance and trust in them. “Our AI could be used to describe the emotional aspect of artworks to disabled people, allowing them to enjoy new experiences,” says Elhoseiny. “We are now studying how different cultures respond emotionally to artwork and hope to uncover further secrets of human intelligence along the way”, he adds. To learn more about the team’s research, visit the project website https://www.artemisdataset-v2.org/.
References
- Mohamed, Y., Khan, F.F., Haydarove, K. & Elhoseiny, M. It is okay to not be okay: overcoming emotional bias in affective image captioning by contrastive data collection. Presented in Proceedings of the IEEE/CVF Conference on Computer Vision and Pattern Recognition (2022).| article
You might also like
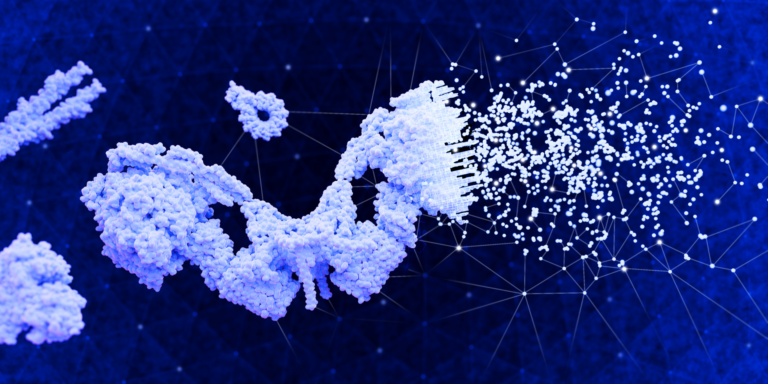
Bioengineering
AI tool predicts function of unknown proteins
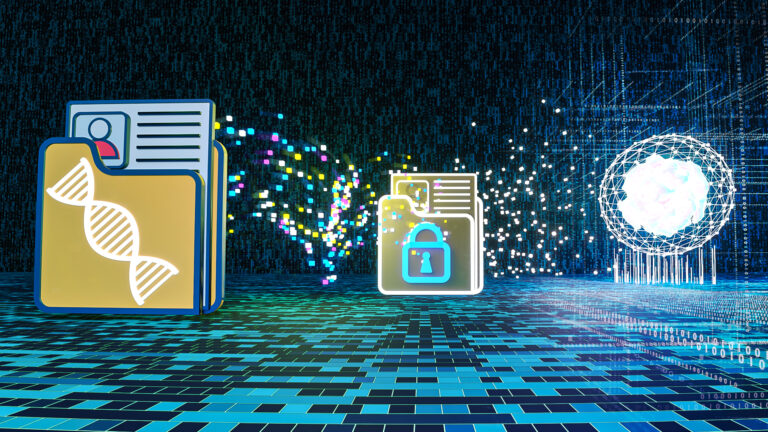
Bioengineering
Shuffling the deck for privacy
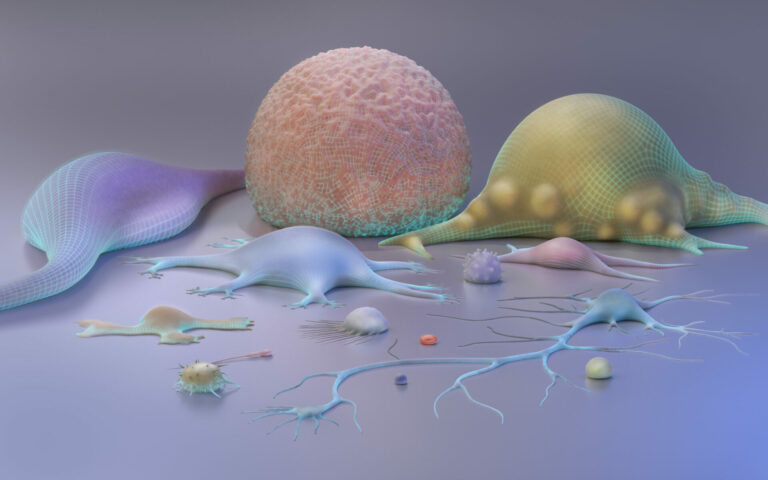
Bioengineering
AI for cells helps illuminate their identity
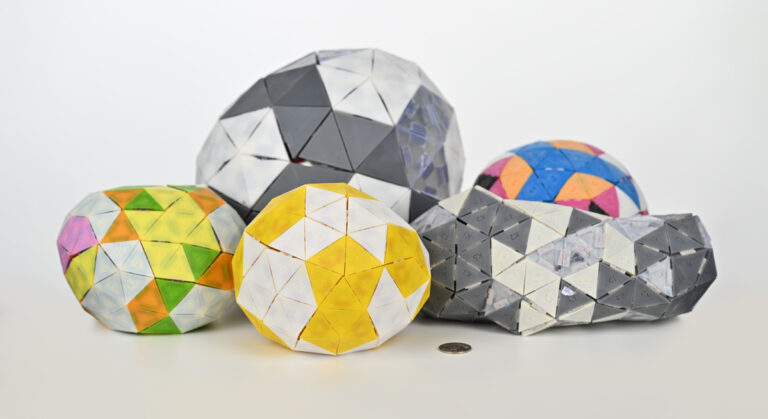
Computer Science
Dr. KID turns biological models into fun puzzles
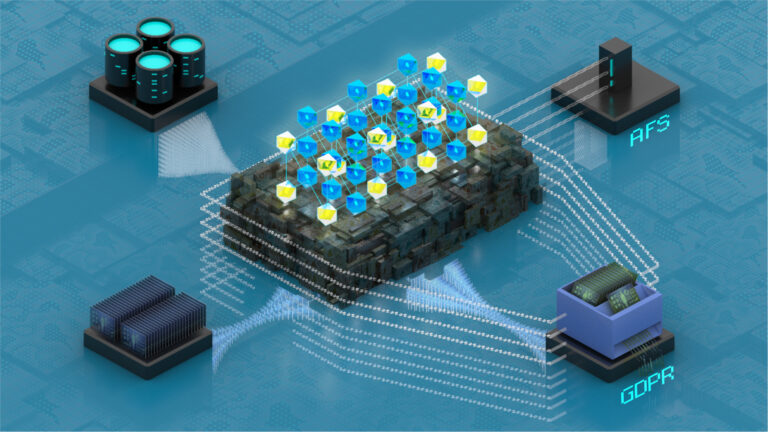
Bioengineering
Safeguarding the right to be forgotten
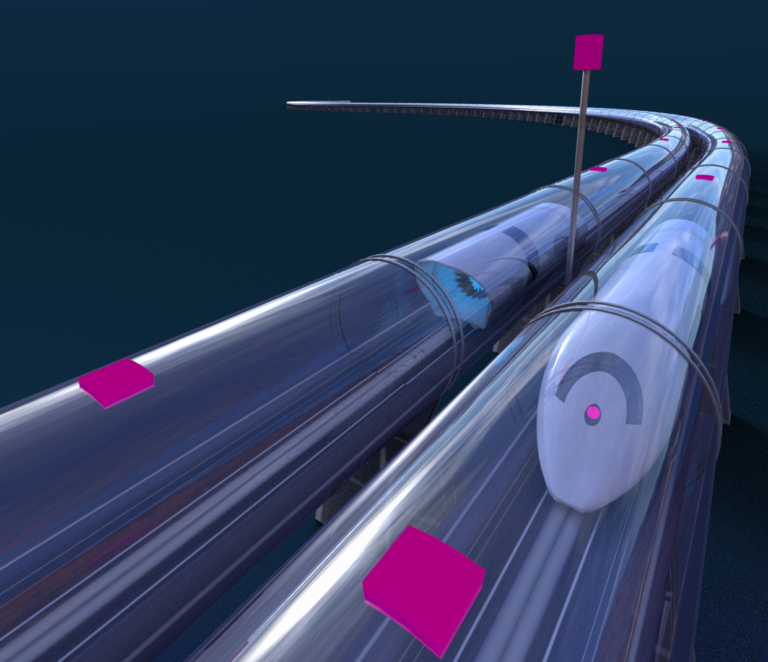
Applied Mathematics and Computational Sciences
Staying online in the high-speed tube of the future
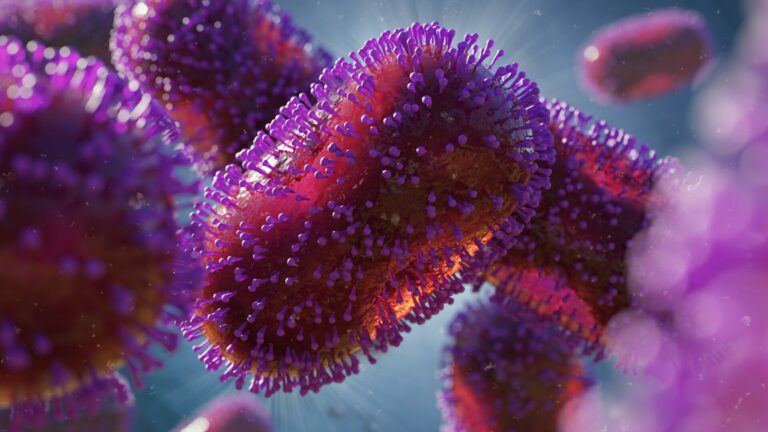
Bioengineering
Lab-grown skin helps unlock secrets of mpox virus infection
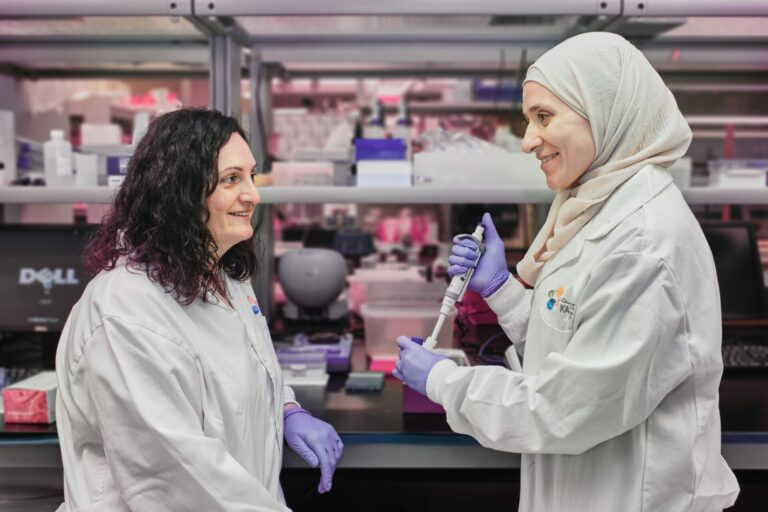
Bioengineering