Statistics
Can machine learning help predict disease spread?
Machine learning techniques used to analyze case data show promise for predicting the evolution of an epidemic.
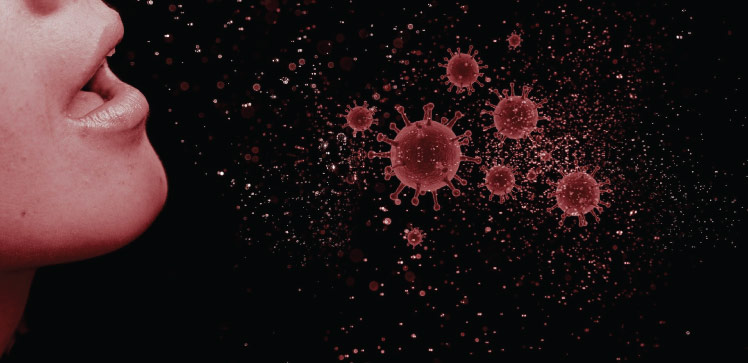
Machine learning techniques can provide an assumption-free analysis of epidemic case data with surprisingly good prediction accuracy and the ability to dynamically incorporate the latest data, a new KAUST study has shown. The proof of concept developed by Yasminah Alali, a student in KAUST’s 2021 Saudi Summer Internship (SSI) program, demonstrates a promising alternative approach to conventional parameter-driven mechanistic models that removes human bias and assumptions from analysis and shows the underlying story of the data.
Working with KAUST’s Ying Sun and Fouzi Harrou, Alali leveraged her experience working with artificial intelligence models to develop a framework to fit the characteristics and time-evolving nature of epidemic data using publicly reported COVID-19 incidence and recovery data from India and Brazil.
“My major at college was artificial intelligence, and I previously worked on a medical project using various ML algorithms,” says Alali. “Working with Professor Sun and Dr Harrou during my internship, we considered whether the Gaussian Process Regression method would be useful for predicting pandemic spread because it gives confidence intervals for the predictions, which can greatly assist decision-makers.”
Accurate forecasting of cases during a pandemic is essential to help mitigate and slow transmission. Various methods have been developed to improve the forecasting of case spread using mathematical and time-series models, but these rely on a mechanistic understanding of how the contagion spreads and the efficacy of mitigation measures such as masks and isolation. Such methods become increasingly accurate as our understanding of a particular contagion improves, but this can lead to erroneous assumptions that might unknowingly affect the accuracy of the modeling results.
As ML techniques are not able to capture the time-dependence of a data series, the team had to come up with a way to dynamically incorporate new data at different points in the learning process by “lagging” the data inputs. They also incorporated a Bayesian optimization method to allow the extracted distributions to be refined for increased accuracy. The result is an integrated dynamic ML framework that performed remarkably well using real case data.
“In this study, we employed machine learning models because of their capacity to extract relevant information from the data with flexibility and without any assumptions regarding the underlying data distribution,” explains Harrou. “GPR is very attractive for handling different kinds of data that follow different Gaussian or nonGaussian distributions, and the integration of lagged data contributes significantly to improved prediction quality.”
References
-
Alali, Y., Sun, Y. & Harrou, F. A proficient approach to forecast COVID-19 spread via optimized dynamic machine learning models. Scientific Reports 12, 2467 (2022).| article
You might also like
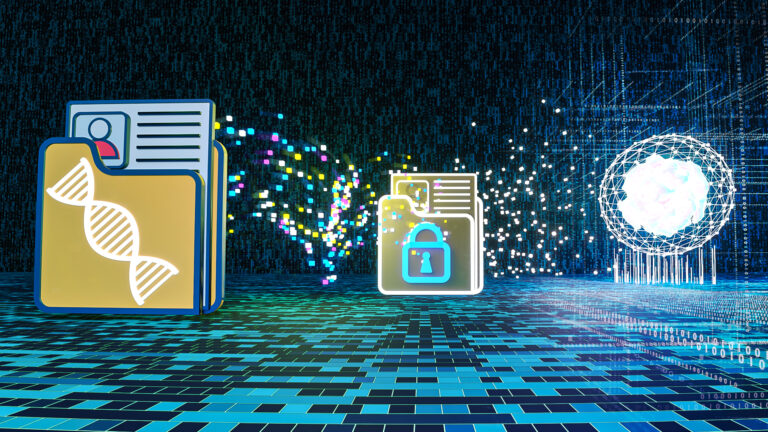
Bioengineering
Shuffling the deck for privacy
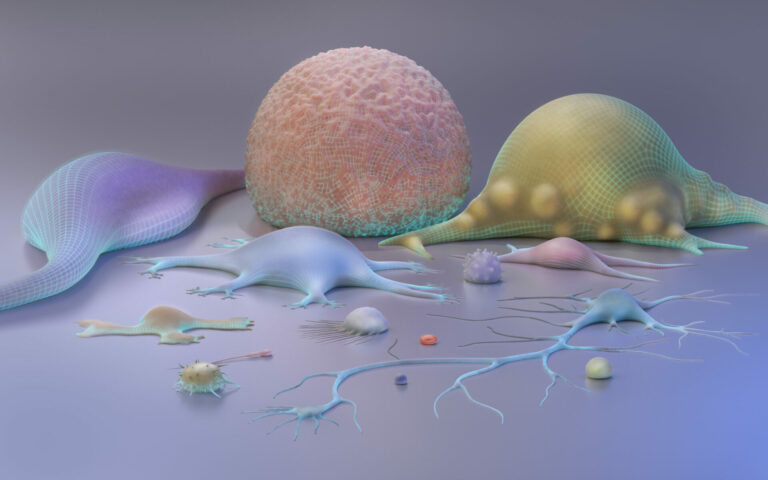
Bioengineering
AI for cells helps illuminate their identity
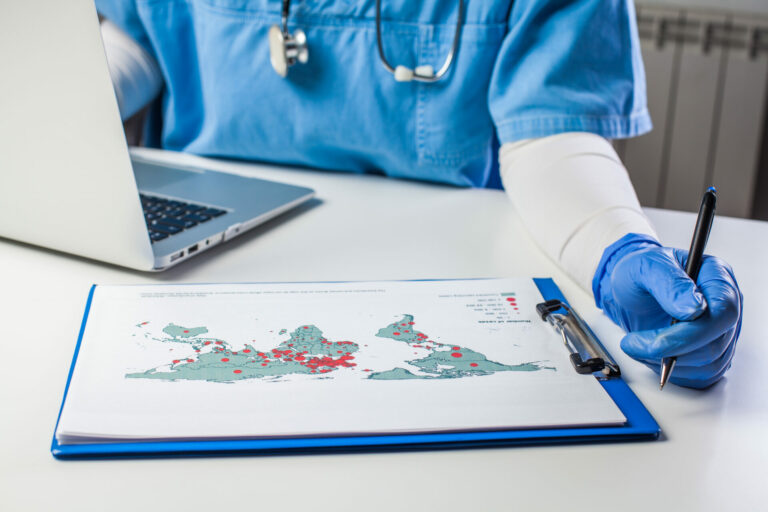
Applied Mathematics and Computational Sciences
Global look at sex differences in young people's mortality
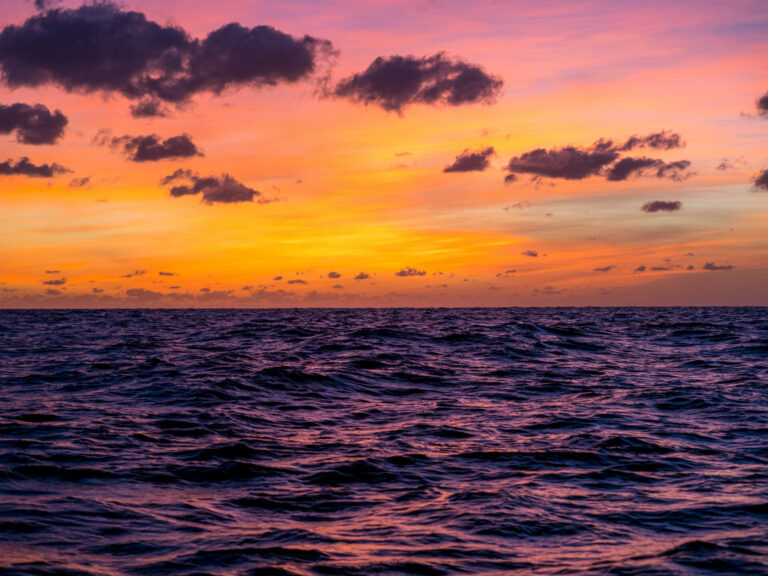
Applied Mathematics and Computational Sciences
Going likelihood-free with neural networks
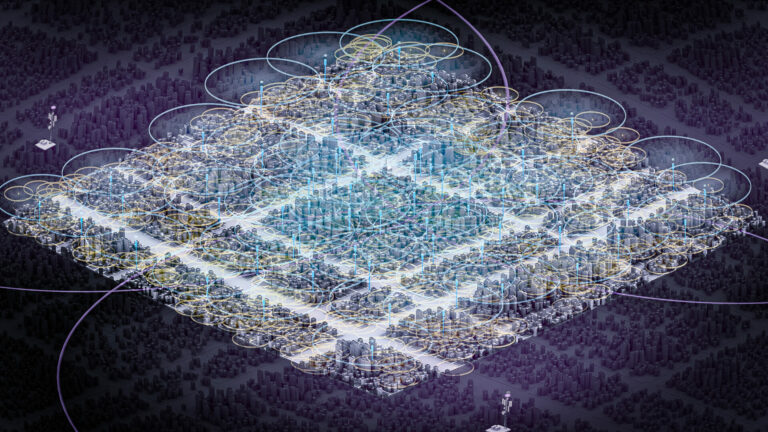
Applied Mathematics and Computational Sciences
A simple solution for frequency sharing
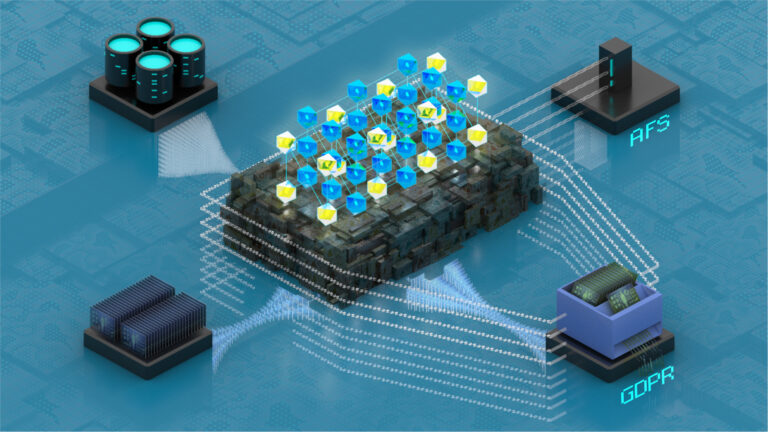
Bioengineering
Safeguarding the right to be forgotten
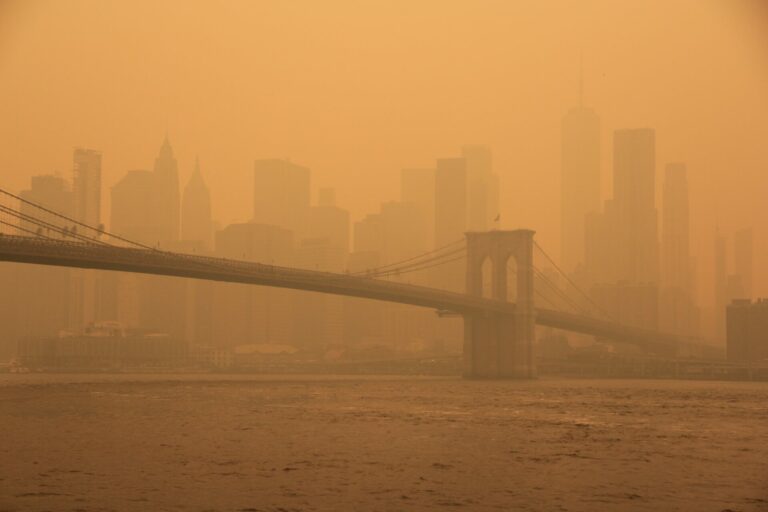
Applied Mathematics and Computational Sciences
Neural networks give deeper insights
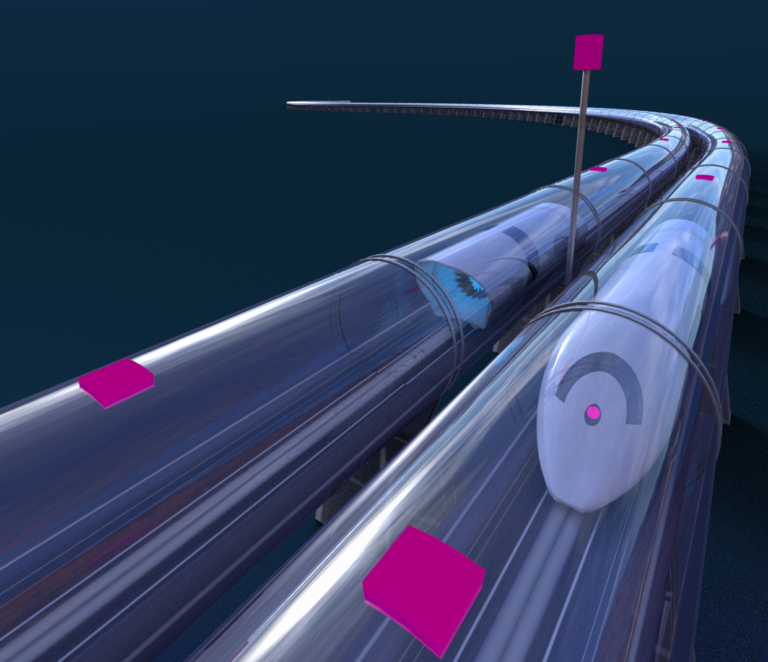
Applied Mathematics and Computational Sciences