Statistics
Competition sheds light on approximation methods for large spatial datasets
Comparing different approximation methods for analyzing large spatial datasets allows for a better understanding of when these methods become insufficient.
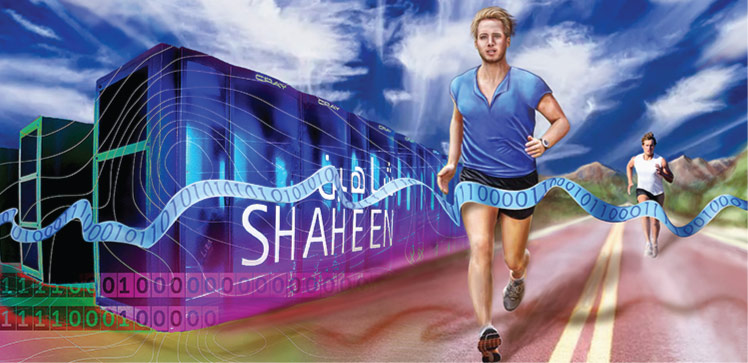
Organizing a global competition between approximation methods used for analyzing and modeling large spatial datasets enabled KAUST researchers to compare the performance of these different methods.
Spatial datasets can contain many different types of data, from topographical, geometric or geographic information, such as environmental or financial data, comprising measurements taken across many locations. The development of advanced observation techniques has led to increasingly larger datasets with high dimensionality, making statistical inference in spatial statistics computationally challenging and very costly.
Various approximation methods can be used to model and analyze these large real-world spatial datasets, where exact computation is no longer feasible and inference is typically validated empirically or via prediction accuracy with the fitted model. However, there have been few studies that compare the statistical efficiency of these approximation methods, and these have been limited to small- and medium-sized datasets for only a few methods.
This motivated Marc Genton, Huang Huang and colleagues from KAUST to organize a competition between different approximation methods to assess their model inference performance.
The competition “was designed to achieve a comprehensive comparison between as many different methods as possible and also involved more recently developed methodologies,” said Huang. “It was also designed to overcome weaknesses in previous studies by incorporating several key features.”
These features included synthetic spatial datasets generated by the ExaGeoStat software, which comprised datasets ranging from 100,000 to one million data points. “With these much larger synthetic datasets, where we know the true processes at scale, we could better compare the statistical efficiency of different approximation methods,” Genton explains.
In addition, the data-generating models represented a wide range of statistical properties for both Gaussian and nonGaussian cases and included both estimation and prediction that were assessed by multiple criteria.
Launched in November 2020, the competition motivated 29 research teams from the global spatial statistics community to register their interest, with 21 teams submitting their results by the close of the competition in February 2021. “By reviewing entries to the competition, we were able to better understand when each approximation method became inadequate,” said Huang, which provided “a unified framework for understanding the performance of existing approximation methods.”
“We now plan to extend the comparison to more complex datasets from multivariate or spatio-temporal random processes.” he adds.
References
- Huang, H., Abdulah, S., Sun, Y., Ltaief, H., Keyes, D.E. & Genton, M.G. Competition on spatial statistics for large datasets (with discussion). Journal of Agricultural, Biological, and Environmental Statistics 26, 580-595 (2021).| article
You might also like
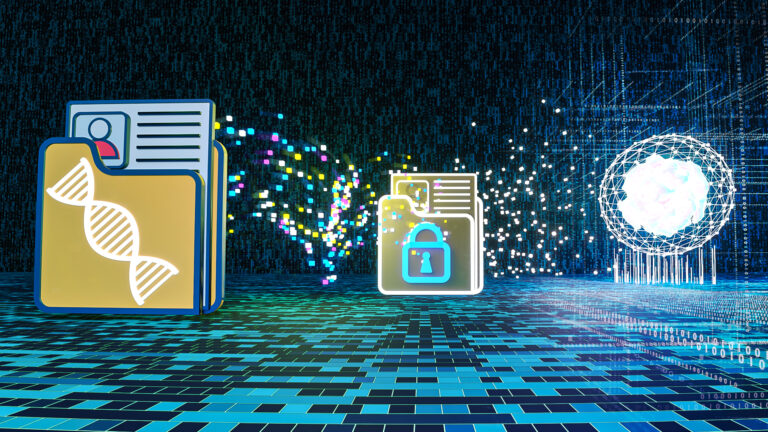
Bioengineering
Shuffling the deck for privacy
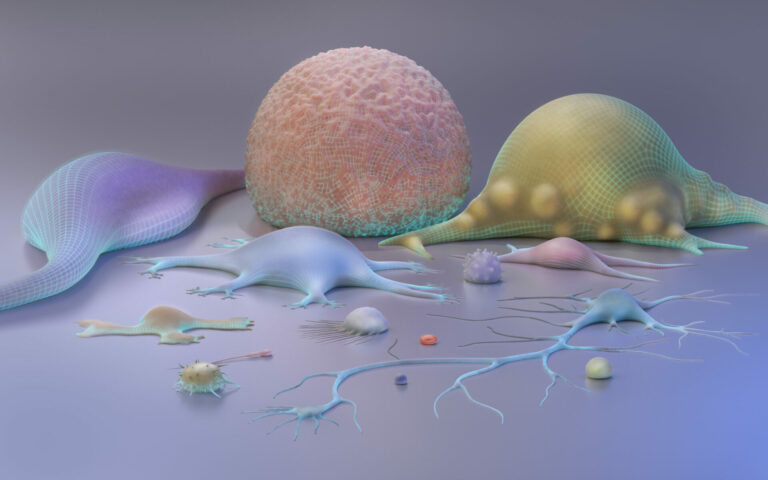
Bioengineering
AI for cells helps illuminate their identity
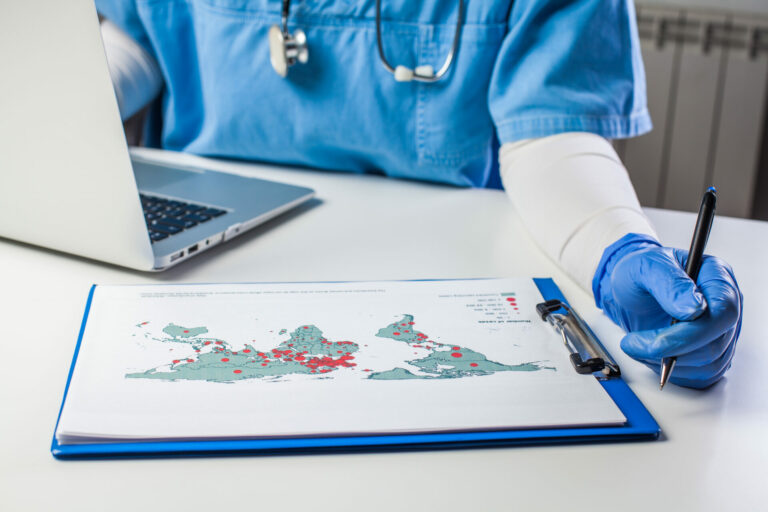
Applied Mathematics and Computational Sciences
Global look at sex differences in young people's mortality
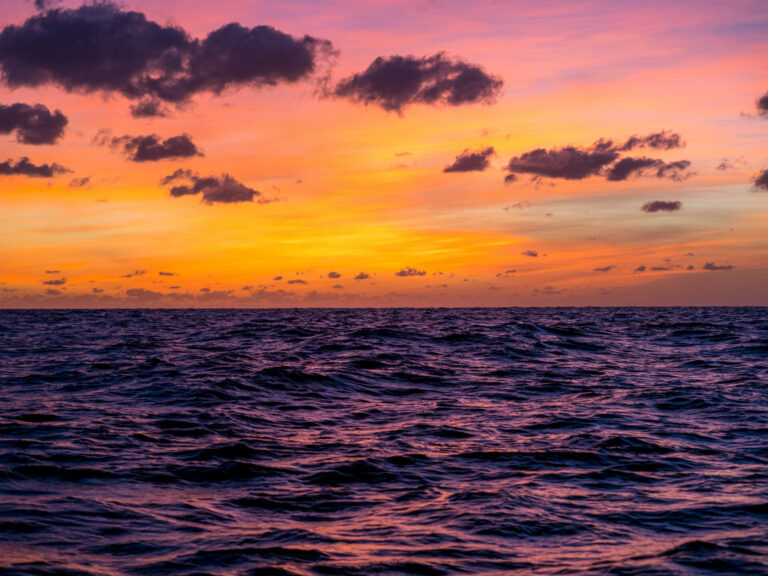
Applied Mathematics and Computational Sciences
Going likelihood-free with neural networks
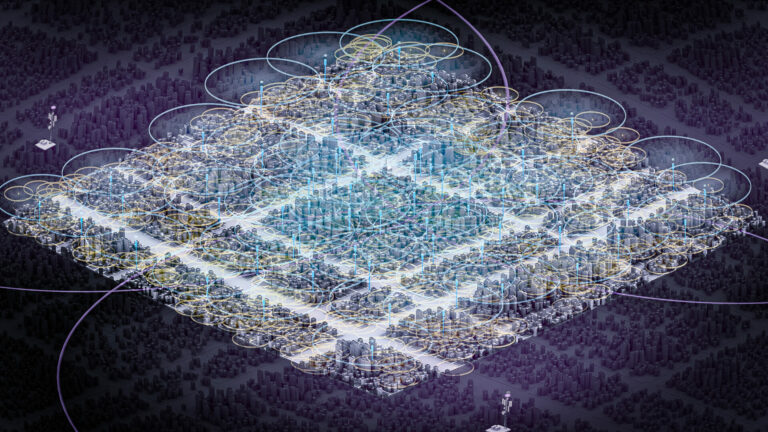
Applied Mathematics and Computational Sciences
A simple solution for frequency sharing
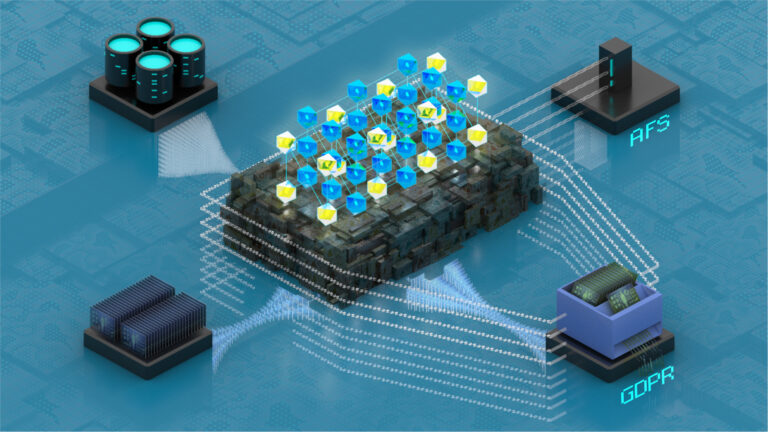
Bioengineering
Safeguarding the right to be forgotten
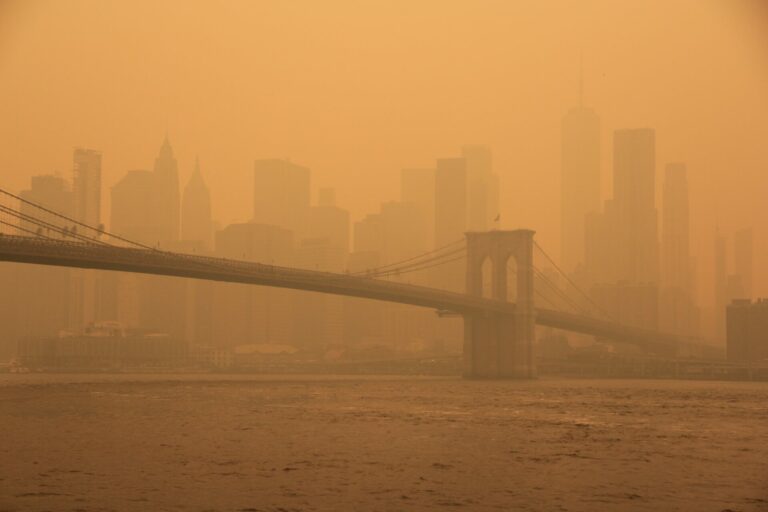
Applied Mathematics and Computational Sciences
Neural networks give deeper insights
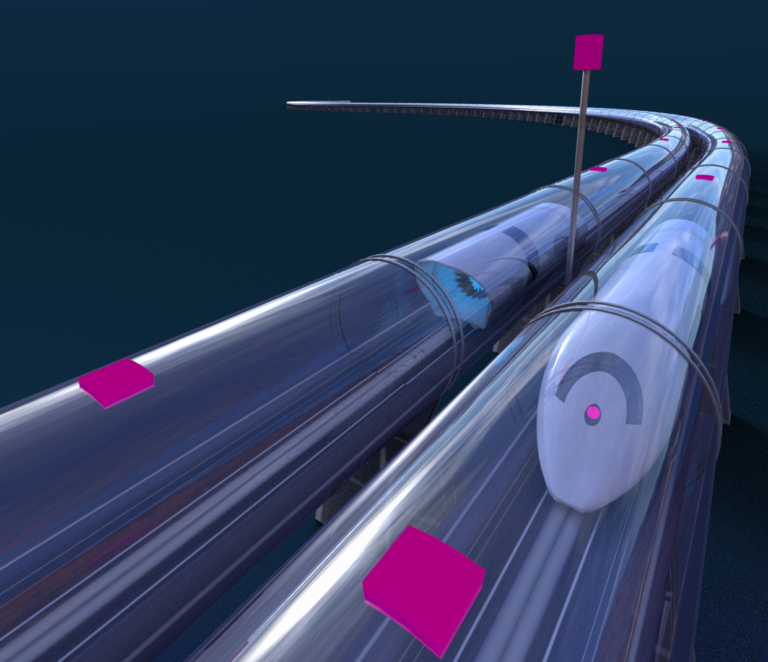
Applied Mathematics and Computational Sciences