Statistics
Finding true protein hotspots in cancer research
Novel statistical methods help tell the difference between "false positives" and true detections of protein domain hotspots that could be linked to cancer.
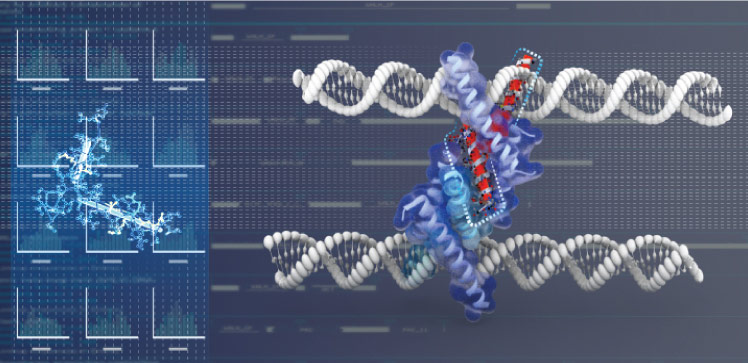
Mutations in proteins with possible links to cancer can be identified more reliably by applying a rigorous test that accounts for false positives. The new statistical approach developed by KAUST researchers has the potential to accelerate cancer research at the molecular level by minimizing false leads and misdirections.
“Investigating mutations at the molecular or protein-domain level is crucial for uncovering mutations functionally related to cancer,” says postdoc Iris Ivy Gauran. “Traditional statistical analyses of tumor samples look for mutations at the gene level. However, studies looking at variants in protein domains — the functional, structural and evolutionary units of proteins — have shown great potential for identifying functionally relevant mutations.”
Finding such acquired or “somatic” mutations involves conducting statistical tests on enormous volumes of protein domain data generated from the molecular analysis of real tumors. These statistical tests yield “hotspots” in certain protein domains where a significant number of molecular variations have been detected. However, the identification of hotspots is unreliable when there is insufficient data to yield confident results, resulting in a high rate of false hotspot detections.
Gauran, collaborating with colleagues from Seoul National University, the University of Maryland and the University of California, has developed a test procedure that accounts more robustly for the false positive rate.
“Identification of protein domain hotspots that occur with significantly higher frequency in a sample set represents a large-scale simultaneous inference problem involving hundreds of hypothesis tests at the same time,” says Gauran. “Our study developed a multiple testing procedure based on the Bayesian local false discovery rate for sparse count data. Using this method, we can select clusters of somatic mutations across entire gene families using protein domain models while controlling the false discovery rate.”
Bayesian methods allow for statistical models that utilize available knowledge about its parameters, in this case a known protein domain model. This allows, for example, a protein domain hotspot identified on the basis of inconsistent molecular variants to be recognized as a false positive and excluded.
To test their method, the research team analyzed protein domain data for prostate cancer, which is known to have an associated protein domain mutation. Their method correctly identified mutation in the DNA binding protein cd00083 as an “oncodomain” with links to cancer.
“Our method successfully eliminated redundant hotspot positions while identifying oncodomains with high putative cancer relevance, and also demonstrates the ability of Bayesian methods to solve a crucial statistical issue in the correct identification of oncodomains,” Gauran says.
References
-
Gauran, I.I.M., Park, J., Rattsev, I., Peterson, T.A., Kann, M.G. & Park, D.-H. Bayesian local false discovery rate for sparse count data with application to the discovery of hotspots in protein domains. Annals of Applied Statistics | article
You might also like
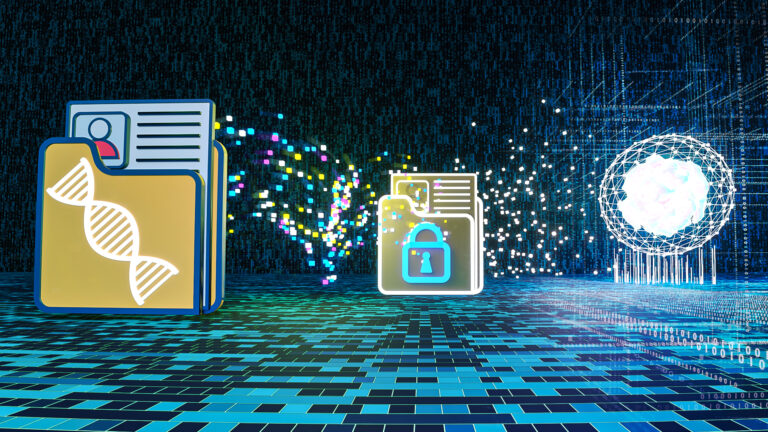
Bioengineering
Shuffling the deck for privacy
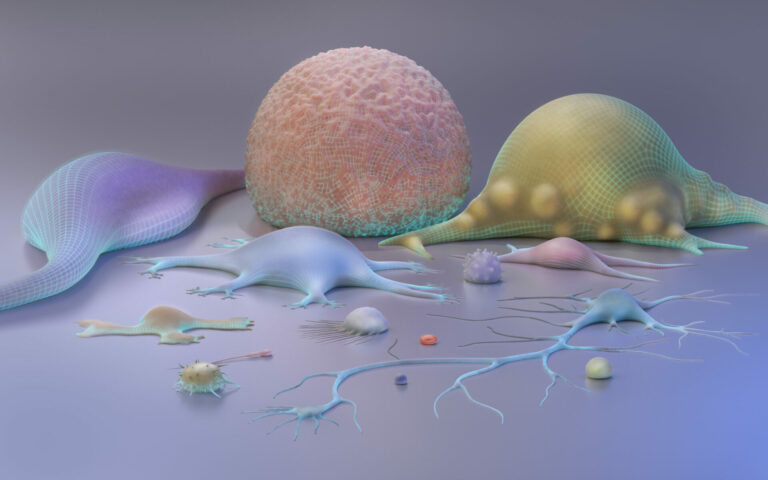
Bioengineering
AI for cells helps illuminate their identity
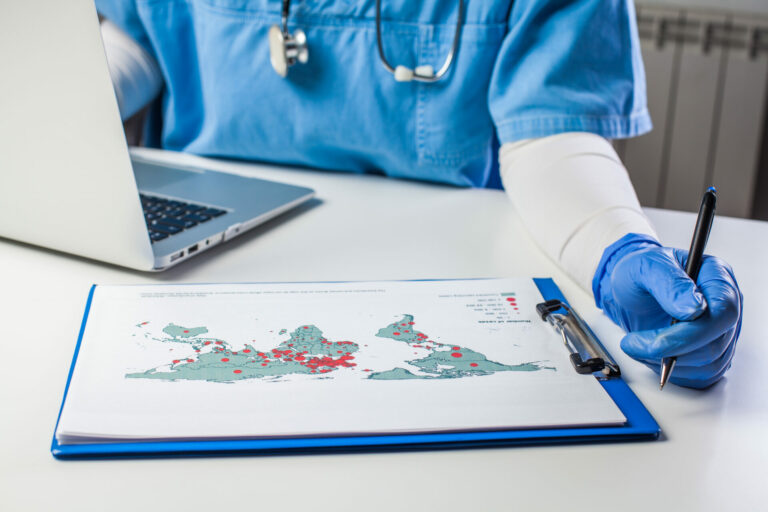
Applied Mathematics and Computational Sciences
Global look at sex differences in young people's mortality
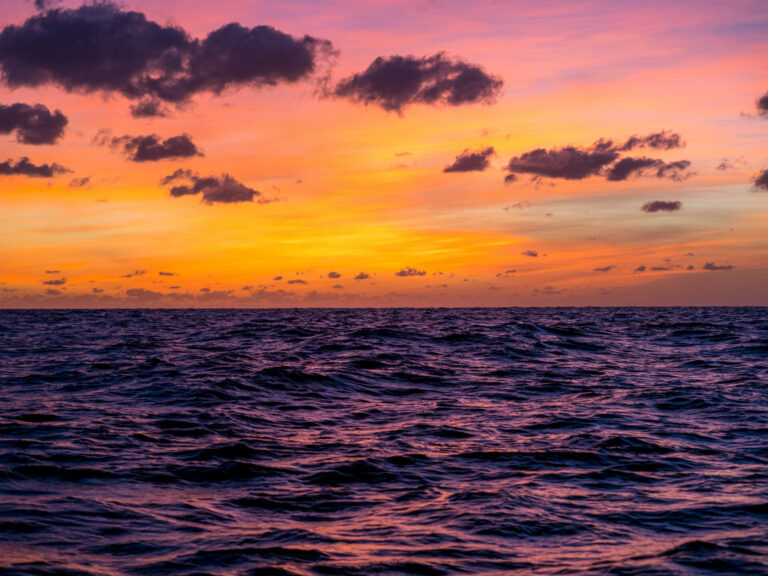
Applied Mathematics and Computational Sciences
Going likelihood-free with neural networks
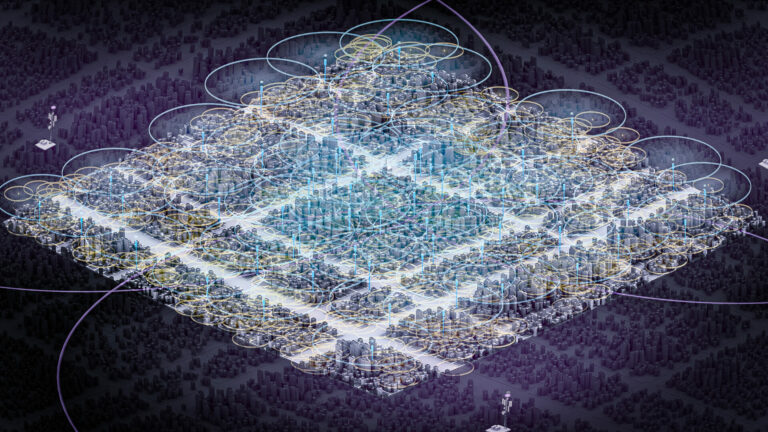
Applied Mathematics and Computational Sciences
A simple solution for frequency sharing
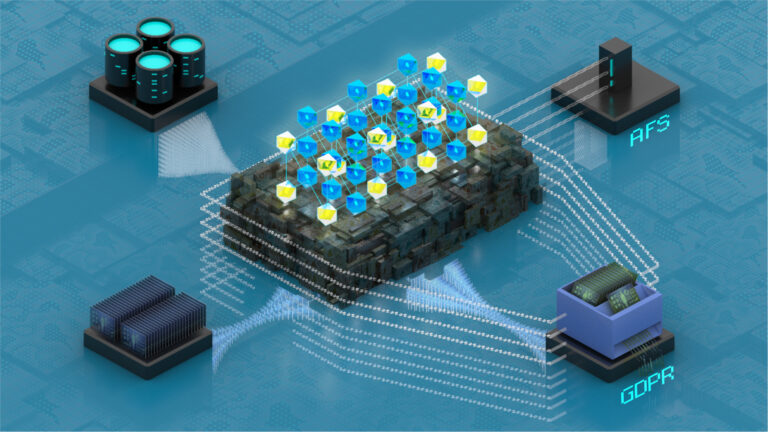
Bioengineering
Safeguarding the right to be forgotten
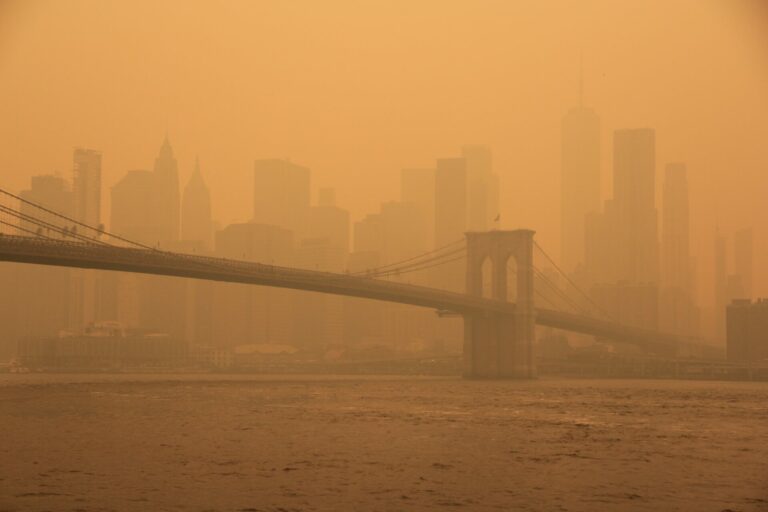
Applied Mathematics and Computational Sciences
Neural networks give deeper insights
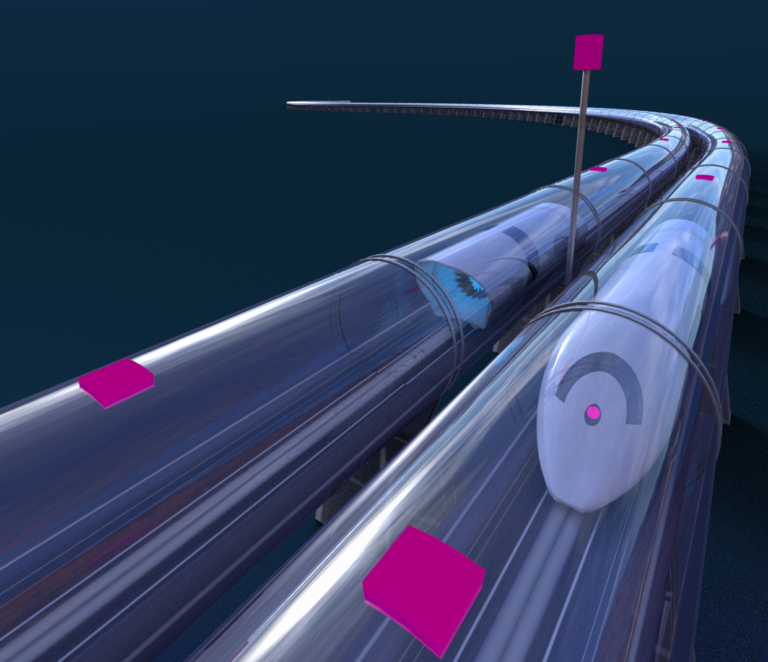
Applied Mathematics and Computational Sciences