Statistics
Starlight, star bright…as explained by math
The evolving periodicity of the brightness of certain types of stars can now be described mathematically.
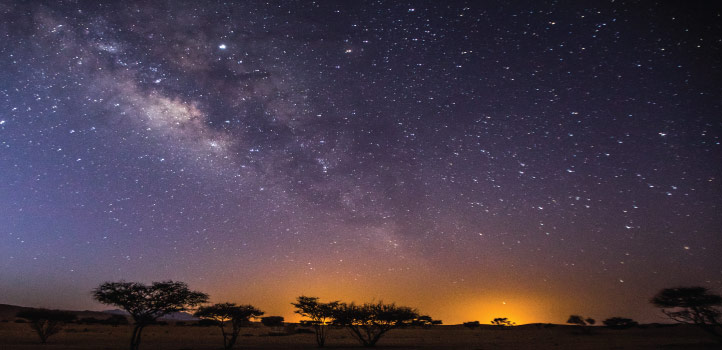
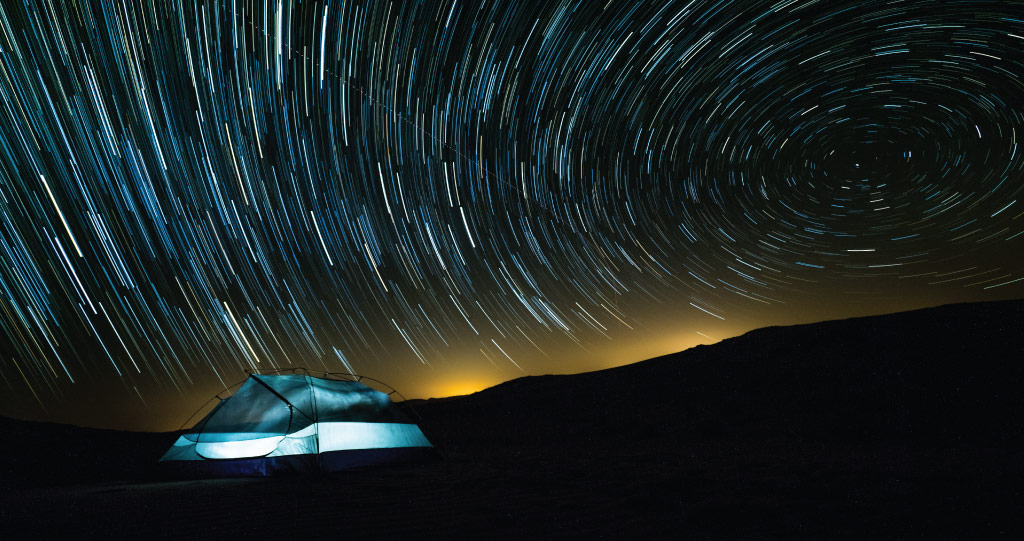
The team applied their method to model the light emitted from the variable star R Hydrae, which exhibited a slowing of its period from 420 to 380 days between 1900 and 1950.
© 2021 Morgan Bennett Smith
“We call such a process an evolving period and amplitude cyclostationary, or EPACS, process,” says Das. “Since EPACS processes are more flexible than cyclostationary processes, they can be used to model a wide variety of real-life scenarios.” Das and Genton modeled the nonstationary period and amplitude by defining them as functions that vary over time. In doing this, they expanded the definition of a cyclostationary process to better describe the relationship among variables, such as the brightness and periodic cycle for a variable star. They then used an iterative approach to refine key parameters in order to fit the model to the observed process. “We applied our method to model the light emitted from the variable star R Hydrae, which exhibited a slowing of its period from 420 to 380 days between 1900 and 1950,” says Das. “Our approach showed that R Hydrae has an evolving period and amplitude correlation structure that was not captured in previous work.” Importantly, because this approach links EPACS processes back to classical cyclostationary theory, then fitting an EPACS process makes it possible to use existing methods for cyclostationary processes. “Our method can also be applied to similar phenomena other than variable stars, such as climatology and environmetrics, and particularly for solar irradiance, which could be useful for predicting energy harvesting in Saudi Arabia,” Das says. To learn more about the model designed by KAUST Ph.D. student Soumya, check out this video: My PhD in 90 seconds #KAUST_WEP_2021 #SCICOMM_VIDEO_COMPETITION
References
- Das, S. & Genton, M.G. Cyclostationary processes with evolving periods and amplitudes. IEEE Transactions on Signal Processing 69, 1579-1590 (2021).| article
You might also like
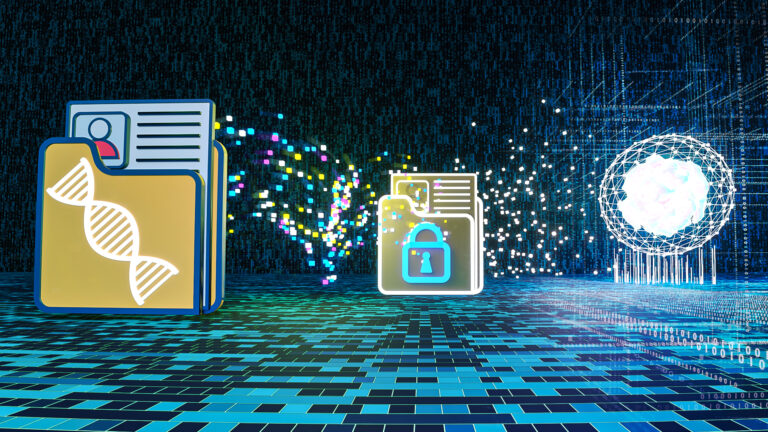
Bioengineering
Shuffling the deck for privacy
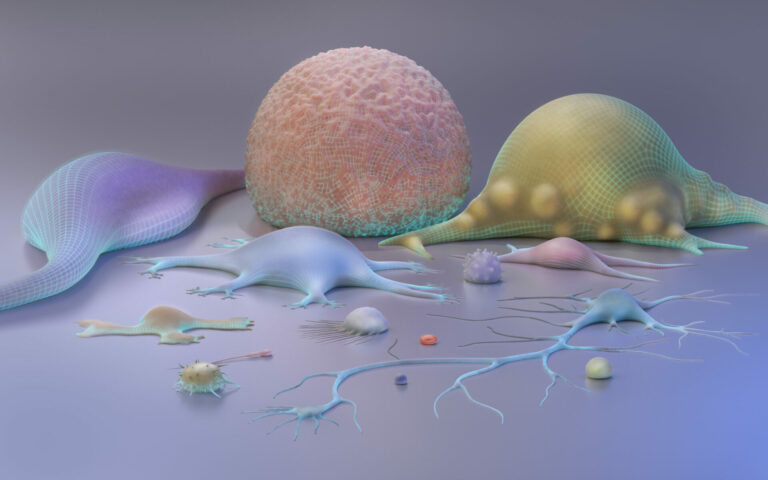
Bioengineering
AI for cells helps illuminate their identity
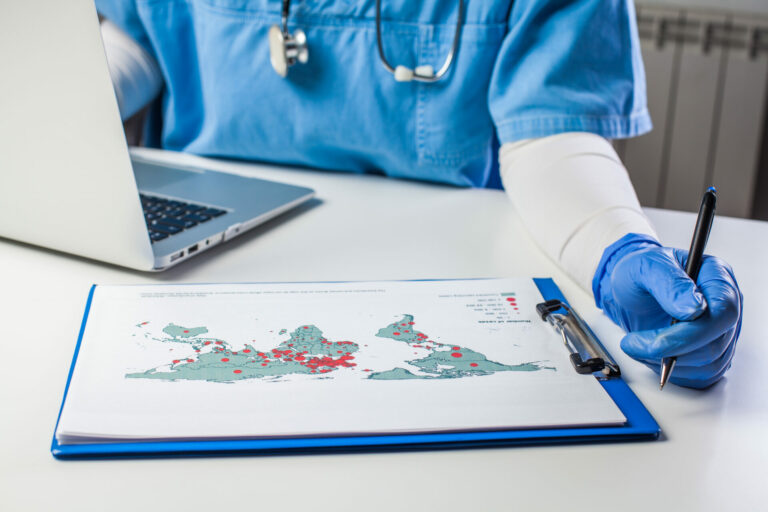
Applied Mathematics and Computational Sciences
Global look at sex differences in young people's mortality
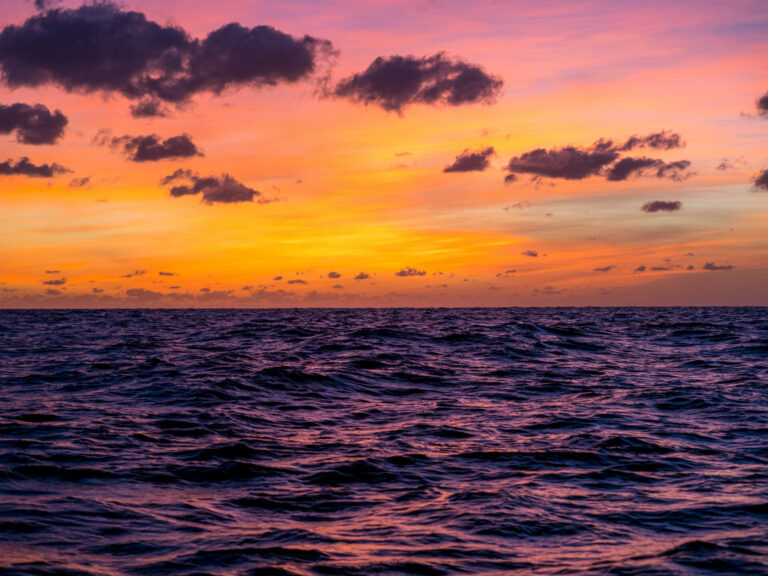
Applied Mathematics and Computational Sciences
Going likelihood-free with neural networks
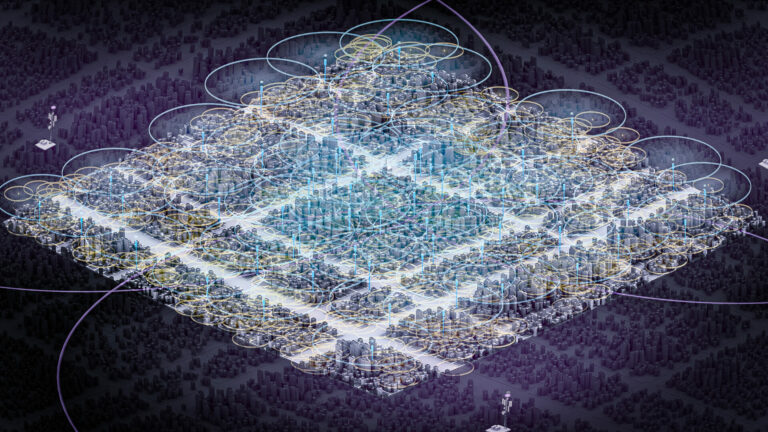
Applied Mathematics and Computational Sciences
A simple solution for frequency sharing
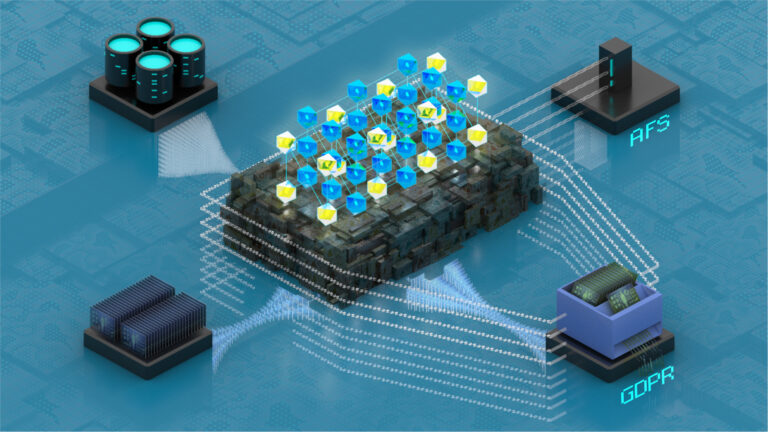
Bioengineering
Safeguarding the right to be forgotten
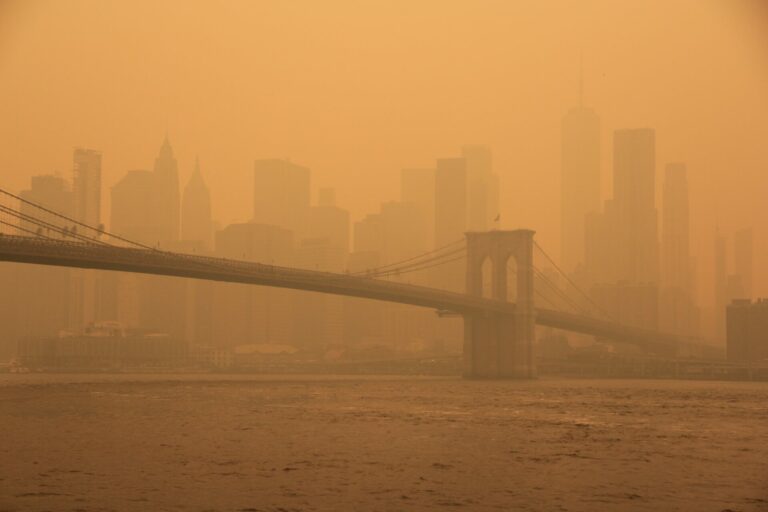
Applied Mathematics and Computational Sciences
Neural networks give deeper insights
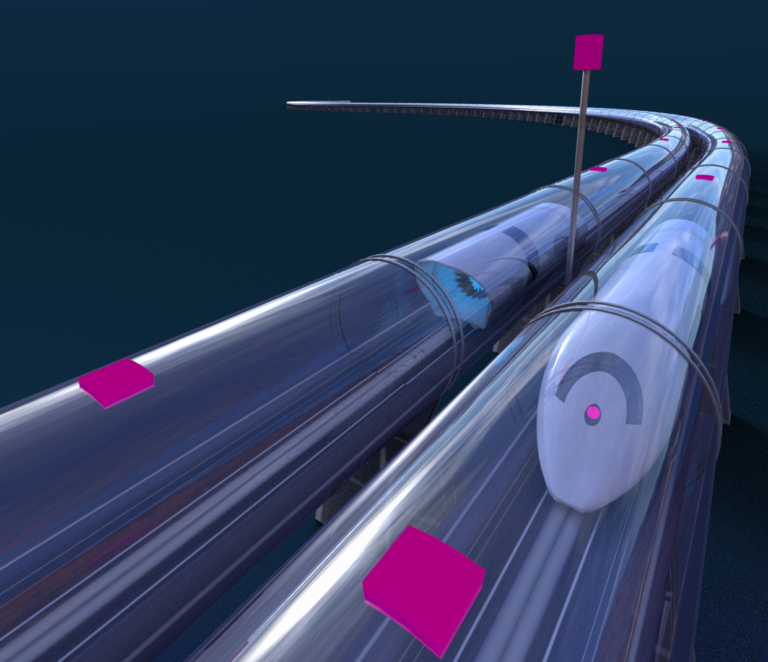
Applied Mathematics and Computational Sciences