Statistics
Predicting future hotspots in the Red Sea
Predicting areas of the Red Sea most susceptible to overheating by the end of the century could help conservation efforts to protect its precious coral reefs.
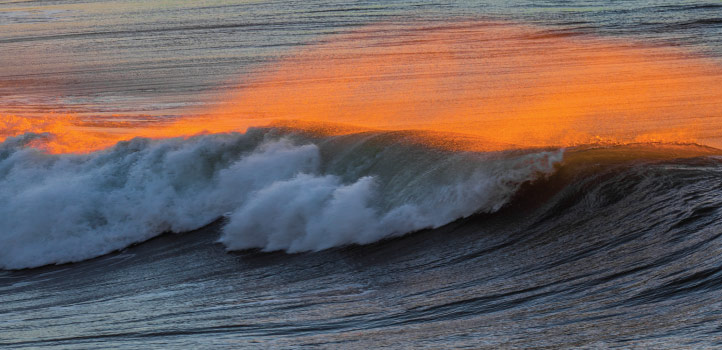
Using high-resolution sea surface temperature (SST) data and advanced statistical methods, KAUST researchers have been able to show that certain areas of the Red Sea are at risk of forming extreme “hotspots” under climate change, which could have disastrous consequences for the sea’s unique coral reefs.
The research also solves some of the challenging problems of deriving meaning from high-resolution observations in complex environmental systems, with broad application for long-term predictions of other climate-dependent extreme conditions.
“The modeling of spatial extremes mostly focuses on low-dimensional situations involving observations at fewer than, say, 100 locations,” says postdoc Arnab Hazra. “We are analyzing satellite-derived SST data at over 16,000 pixels across the Red Sea for the period 1985 to 2015. This is a very large dataset for which existing spatial extremes methods are practically infeasible.”
Adding to the level of difficulty, the SST data also vary seasonally and the correlation or dependence between spatial locations is not fixed. Hazra, with lab director Raphaël Huser, tackled each of these challenges, in turn, using a suite of advanced statistical approaches.
“Because the data is very high-dimensional, even fitting a simple Gaussian process — the simplest approach available — is not computationally feasible,” says Hazra. “We overcome this by using a hierarchical low-rank modeling approach.”
A low-rank model is useful for reducing the dimensionality of a dataset by generating a condensed mathematical representation for every row of data in the dataset. This can reduce the computational complexity by many orders of magnitude, while maintaining the capacity to pick up extremes in the dataset.
“We applied a similar low-rank approach to the spatially and seasonally varying features of the dataset, combined the low-rank models using matrix projections and used empirical orthogonal functions to capture the data’s nonstationarity,” says Hazra.
By fitting their model to observed SSTs throughout the Red Sea and incorporating climate model projections for different scenarios of greenhouse gas concentration pathways, Hazra and Huser identified spatially and seasonally varying patterns of climate change. They showed that some regions, particularly where there are large coral reefs, have the potential to experience very high SSTs by the end of the century.
“We observe that such hotspot regions could cover the large coral reefs of the Dahlak Islands of Eritrea and the Farasan Islands of Saudi Arabia, which is concerning because high SSTs are known to heavily impact coral reefs,” Hazra says.
To learn more about the work of Prof. Raphaël Huser’s group on extreme statistics, visit their website: https://cemse.kaust.edu.sa/extstat
References
- Hazra, A. & Huser, R. Estimating high-resolution red sea surface temperature hotspots, using a low-rank semiparametric spatial model. Annals of Applied Statistics arXiv preprint arXiv:1912.05657 (2021).| article
You might also like
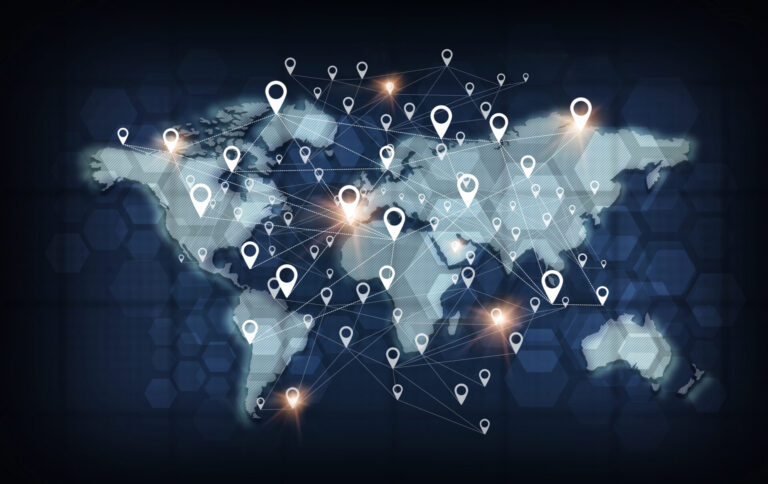
Statistics
Joining the dots for better health surveillance
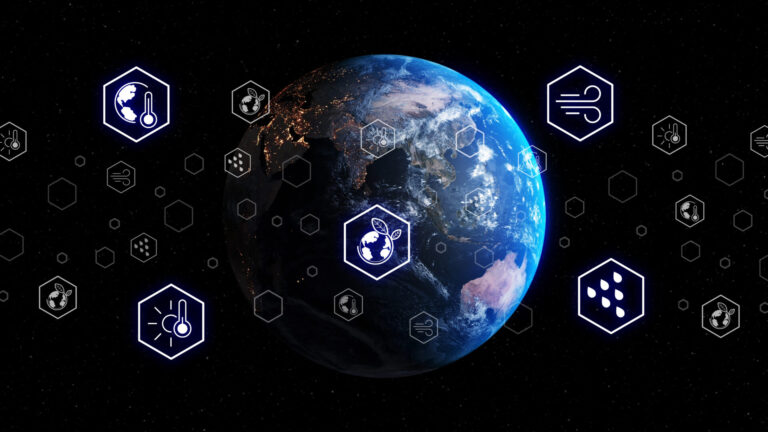
Statistics
Easing the generation and storage of climate data
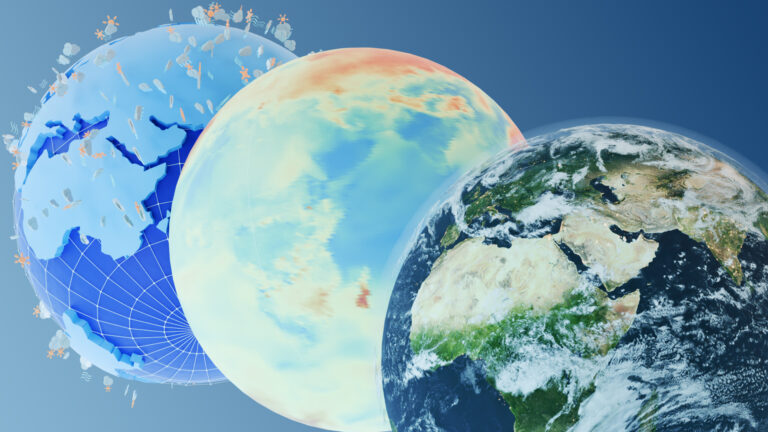
Statistics
A high-resolution boost for global climate modeling
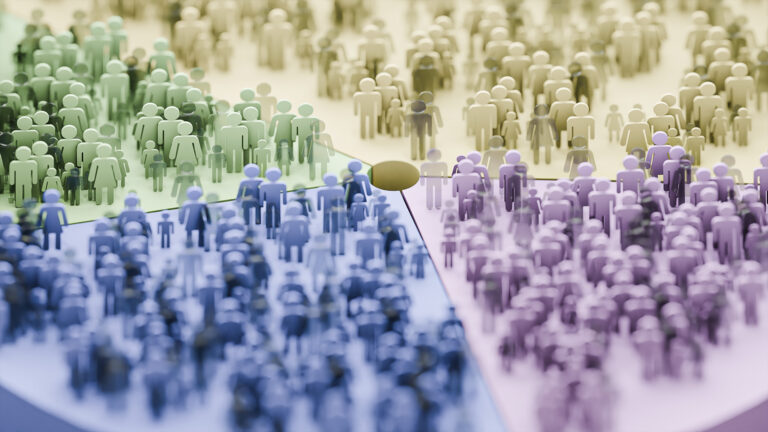
Applied Mathematics and Computational Sciences
Finer forecasting to improve public health planning
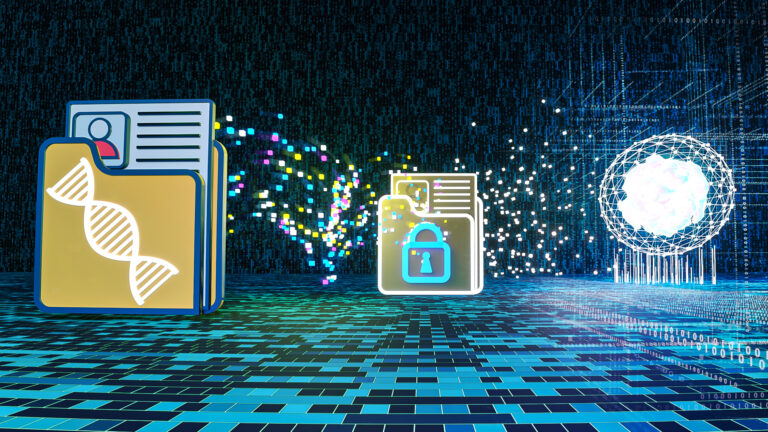
Bioengineering
Shuffling the deck for privacy
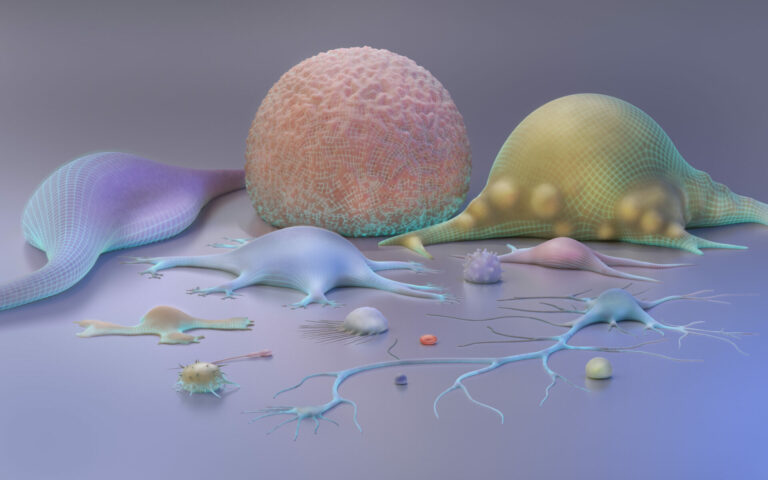
Bioengineering
AI for cells helps illuminate their identity
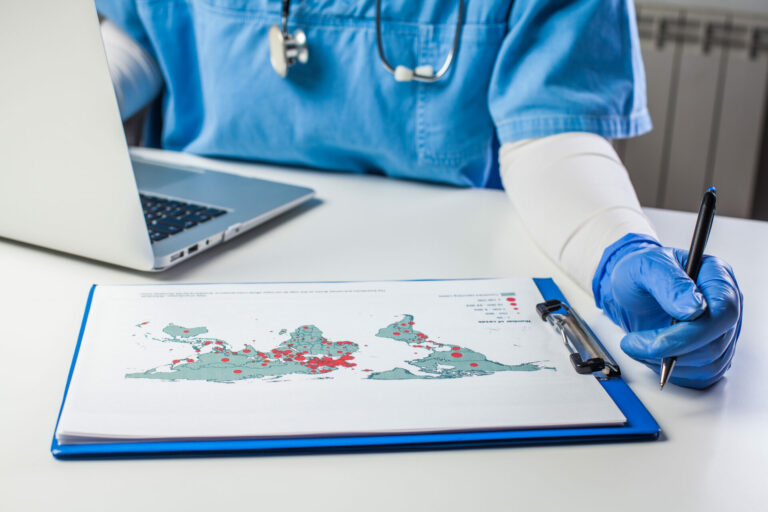
Applied Mathematics and Computational Sciences
Global look at sex differences in young people's mortality
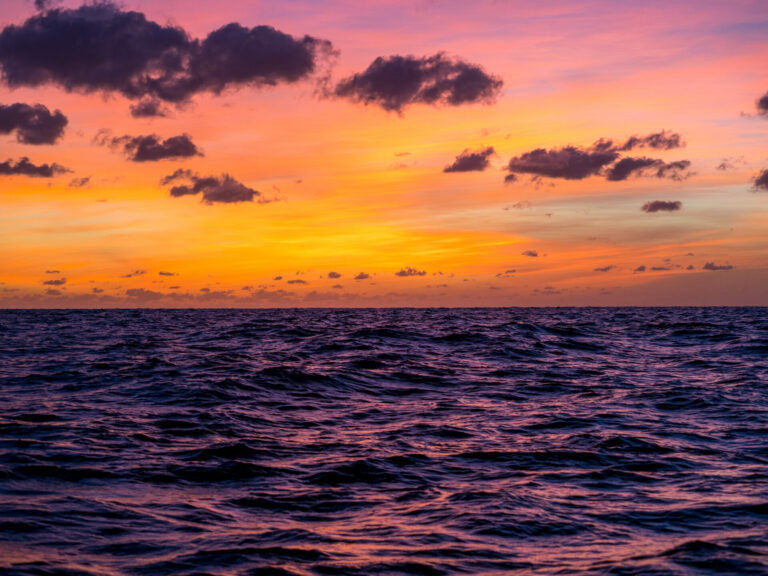
Applied Mathematics and Computational Sciences