Computer Science
Tweaking tools to track tweets over time
Computer model learns to identify Twitter users’ evolving interests by analyzing their Tweets.
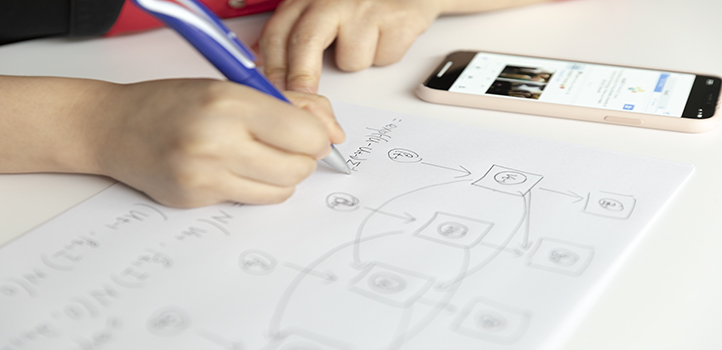
Your social media posts reveal a lot about you. KAUST researchers have developed a dynamic computational model that can analyze tweets to identify Twitter users’ interests and track changes over time.
KAUST researchers have developed a dynamic computational model that can analyze Twitter users’ stream of tweets to identify their interests and track changes over time.
© 2019 KAUST
“Understanding the evolution of users’ interests means we can group them accordingly and recommend friends, news, events and other services,” says Xiangliang Zhang who led the research at KAUST.
Creating computer models that can identify a person’s evolving interests from their social media posts is a multifaceted problem. The first challenge is to understand the meaning of the posted text, a research area known as natural language processing (NLP). “The objective of NLP is to make computers as intelligent as human beings in understanding language,” Zhang says. “It is one of the most challenging tasks of AI,” she adds.

Xiangliang’s dynamic computational models can analyze tweets to identify Twitter users’ interests.
© 2019 KAUST
Rule-based NLP models have not been very successful at interpreting the nuance of language in the diverse and creative way that humans use words, such that the meaning of words can often be highly dependent on context. One alternative approach is to apply machine learning to represent words in a semantic space—where semantically related words for example, Paris, Beijing and Riyadh—are mapped closely together.
To identify Twitter users’ interests by analyzing their tweets, the key challenge is to characterize individual users by their most important keywords. Zhang and her team has created an embedding model in which words and users are handled together. “We created a dynamic-user and word-embedding model that can jointly and dynamically learn user and word representations in the same semantic space,” Zhang says.
The researchers improved the model’s output by developing and incorporating a streaming keyword diversification component, which can identify closely related keywords and remove redundant entries from the top keyword list. The resulting model can capture a diverse range of interests for each user and adapt to their evolving interests over time.
When the team tested their model on a set of tweets, it was a significant improvement on previous approaches, Zhang says. “Our model significantly outperforms many state-of-the-art user-profiling models.” The team has already produced a new iteration of their embedding model approach, she adds, in which user-user relationships are also captured to begin to identify interests that users have in common. “The next model will be more advanced and build dynamic co-embedding vectors that capture the user-user social proximity and user-attribute relevance simultaneously,” Zhang says.
References
-
Liang, S., Zhang. X., Ren, Z., & Kanoulas, E. Dynamic embeddings for user profiling in Twitter. Proceedings of the 24th ACM SIGKDD International Conference on Knowledge Discovery & Data Mining, 1764-1773 (2018).| article
You might also like
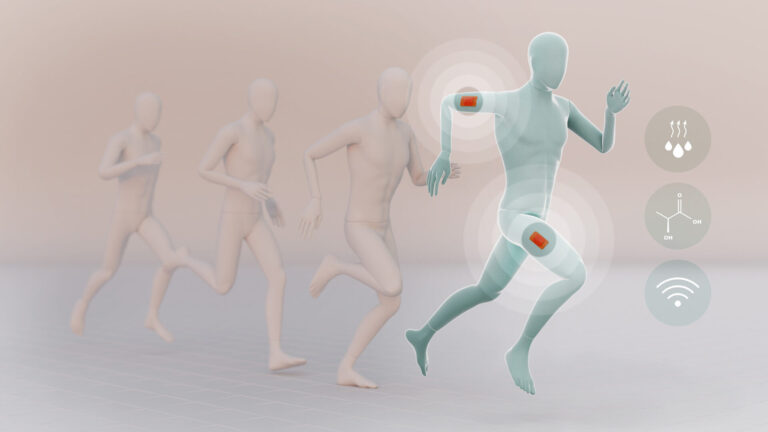
Computer Science
Sweat-sniffing sensor could make workouts smarter
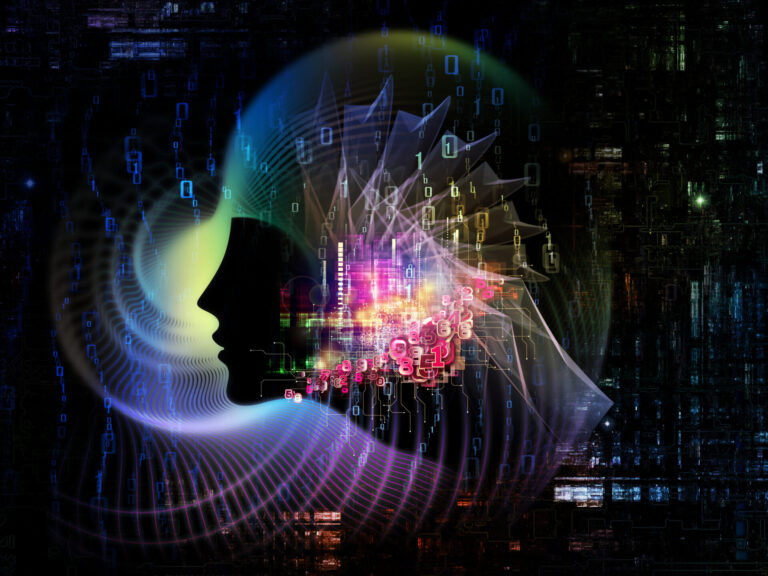
Computer Science
A blindfold approach improves machine learning privacy
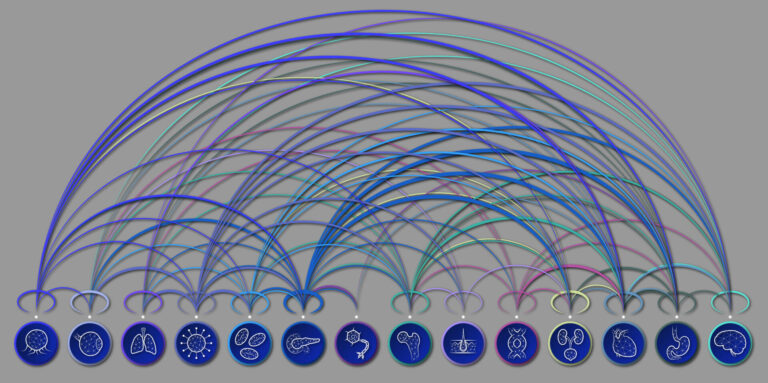
Computer Science
AI tool maps hidden links between diseases
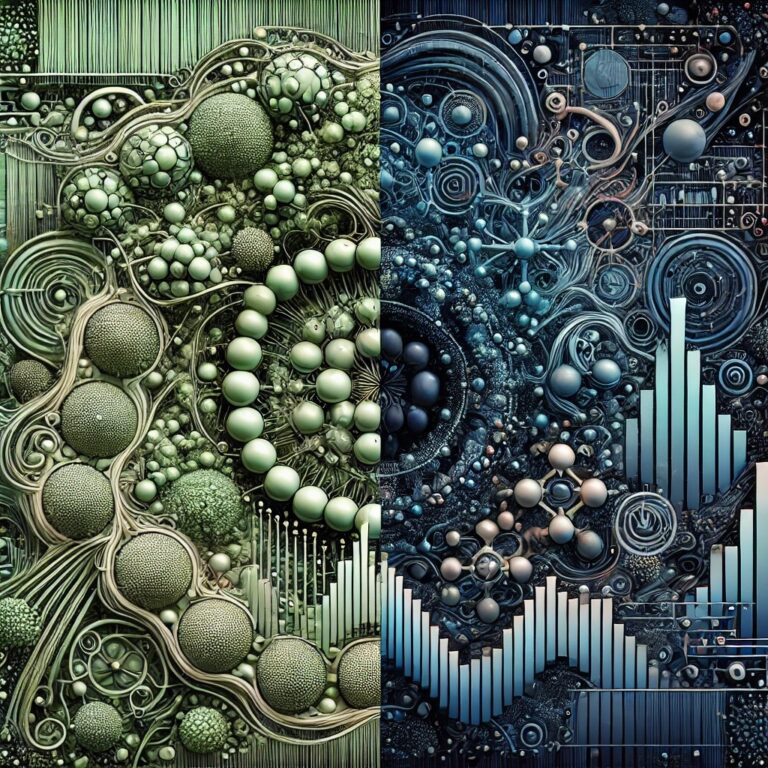
Bioscience
The theory of everything that wasn’t
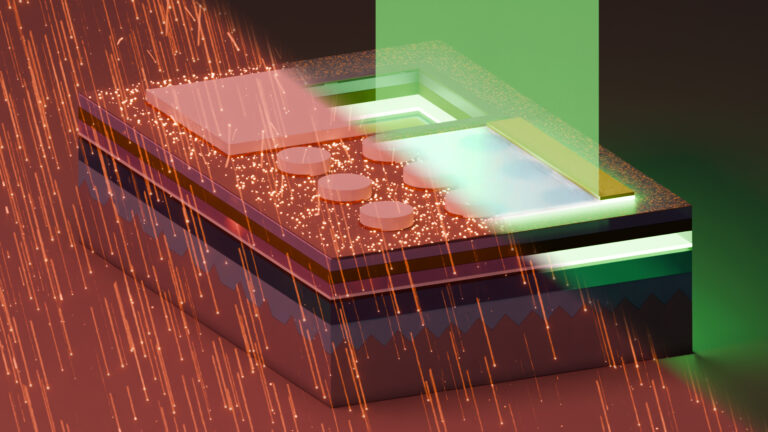
Computer Science
A new path to high-efficiency micro-LEDs
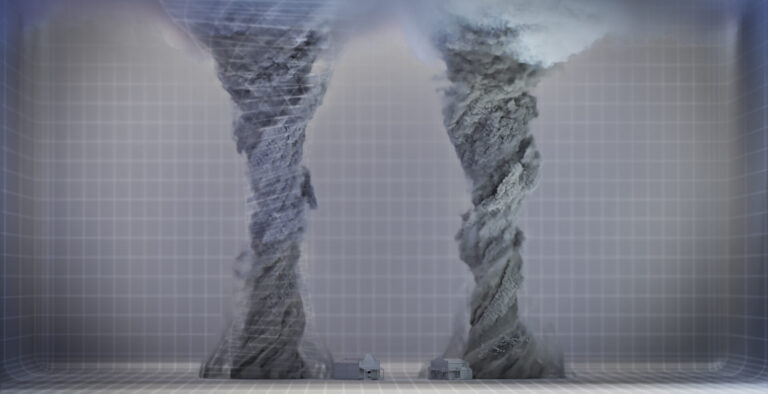
Computer Science
Cyclones meet their mathematical match
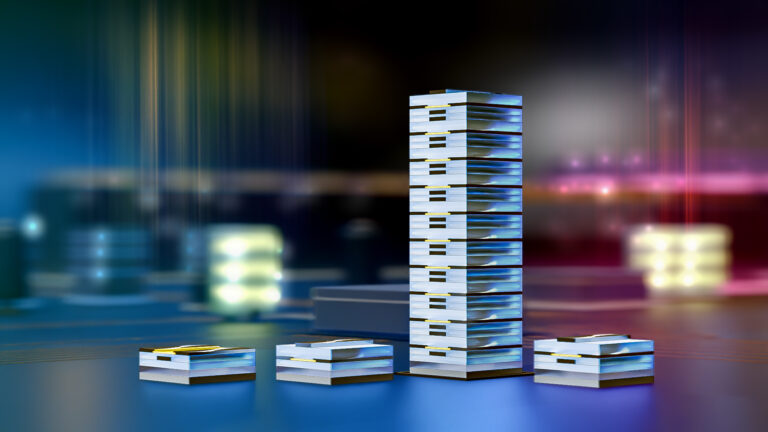
Applied Physics
Onward and upward to smaller faster devices
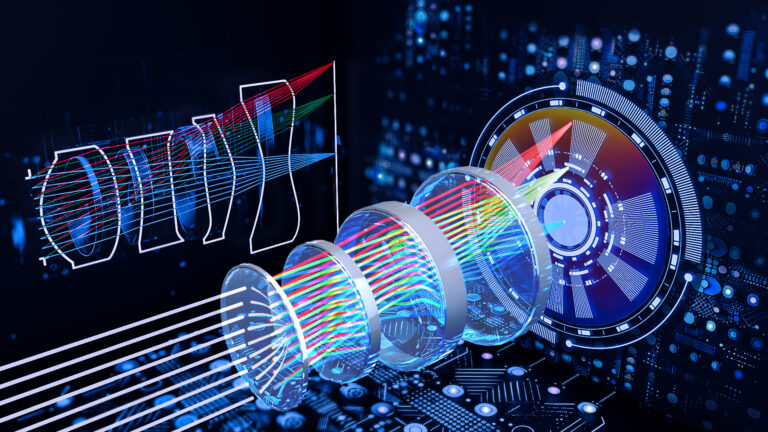
Computer Science