Statistics
How extreme weather exacerbates air pollution
Simultaneously modeling air pollutants and weather under extreme conditions highlights the potential for serious health risks.
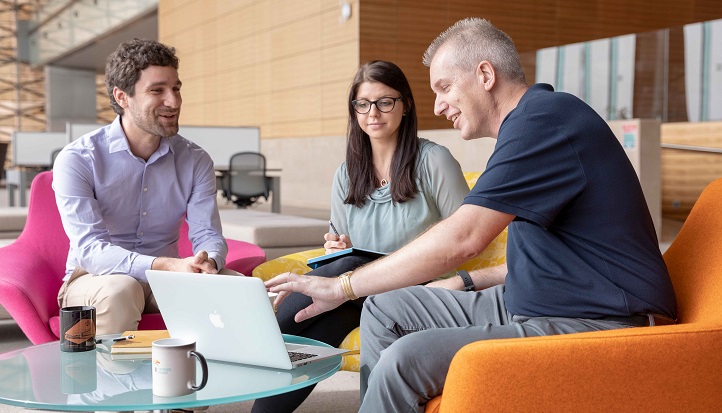
To better understand the effects of air pollution, Marc Genton, Raphaël Huser and Sabrina Vettori from KAUST have applied their expertise in modeling spatial extremes to assess the dependence between peak exposures of multiple air pollutants and extreme weather conditions across large spatial regions.
Air pollution is estimated by the World Health Organization (WHO) Global Ambient Air Quality Database (update 2018) to be responsible for more than 4 million premature deaths each year due to lung cancer, acute respiratory disease and even heart disease and stroke. The threat is quickly becoming a global crisis with over 90 percent of the world’s population living in places where air pollution exposure regularly exceeds limits set by the WHO.
Understanding and predicting the effects of air pollution, however, is complicated by the many varied pollutants, including ozone, particulate matter, nitrogen dioxide and sulfur dioxide, and their cumulative effects and interactions with meteorological conditions, such as temperature and humidity.
“There is growing interest in the health effects of interactions between high concentrations of ozone and high temperatures,” says Vettori. “Especially given the projected increasing temperature trends as a result of climate change.”
The probability of multiple variables, such as pollutant concentrations or temperature, peaking simultaneously can be estimated statistically by modeling the interdependence among variables. However, classical statistical methods, which are often based on the Gaussian distribution, generally underestimate the probabilities associated with extremes. Estimation of models for extremes is also difficult due to the rarity of such events in the statistical record.
“The idea behind our work was to develop intuitive statistical methods to describe the dependencies between multiple extreme phenomena collected at different spatial locations,” explains Vettori.
The team propose a new class of multivariate max-stable processes, essentially a hierarchical tree structure that is nested according to the complexity of the dependence relationships between the various spatial variables. The result is a statistical model for estimating the probability that multiple variables will reach high levels simultaneously across a wide spatial region.
The study showed that ozone levels are expected to exceed safe thresholds within the next 20 years due to increasing temperatures.
“This method provides a comprehensive estimate of air quality that takes into account the extremes of multiple pollutants over a large spatial region, which could be used to gauge public health risks associated with exposure,” says Vettori. “We believe it could have direct applications in multivariate data analysis for air pollution monitoring, where it could help guide policy making.”
References
-
Vettori, S., Huser, R. & Genton, M. Bayesian modeling of air pollution extremes using nested multivariate max-stable processes. arxiv.org: 1804.04588 (2018).| article
You might also like
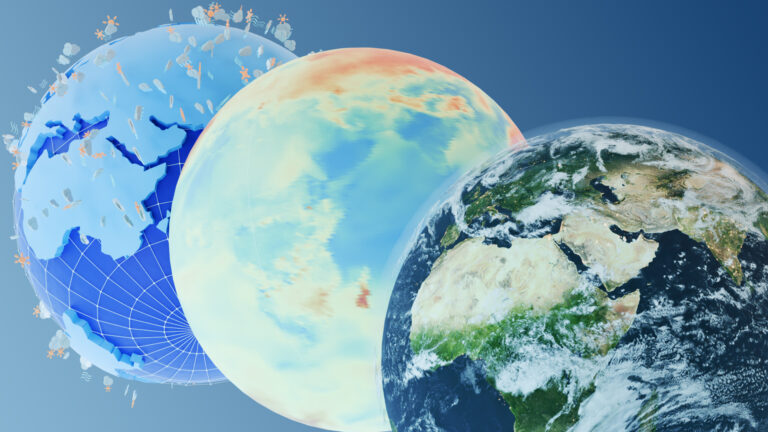
Statistics
A high-resolution boost for global climate modeling
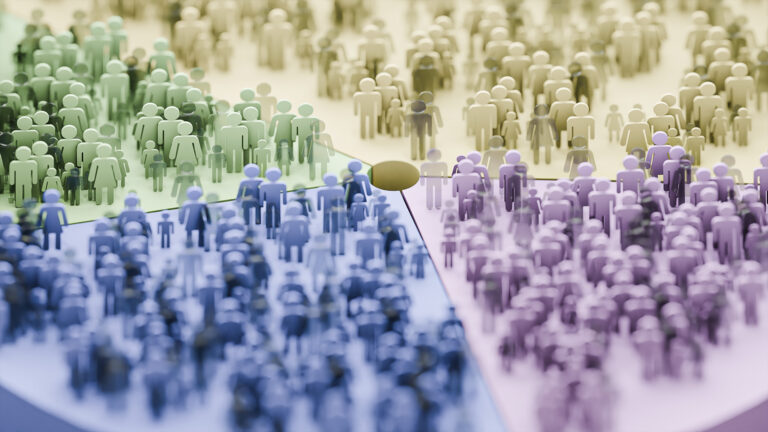
Applied Mathematics and Computational Sciences
Finer forecasting to improve public health planning
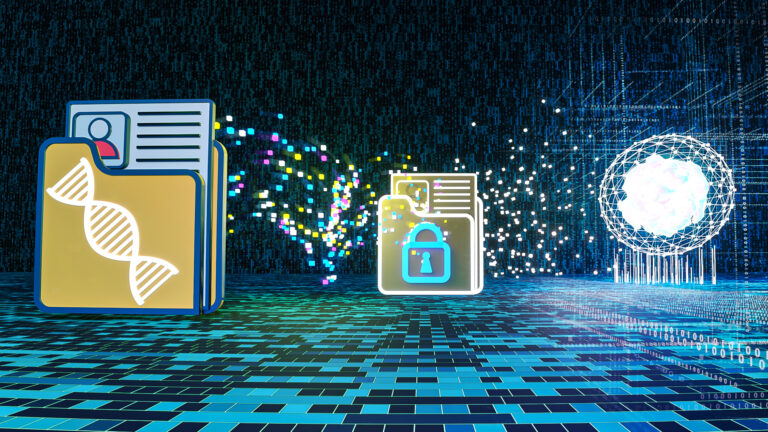
Bioengineering
Shuffling the deck for privacy
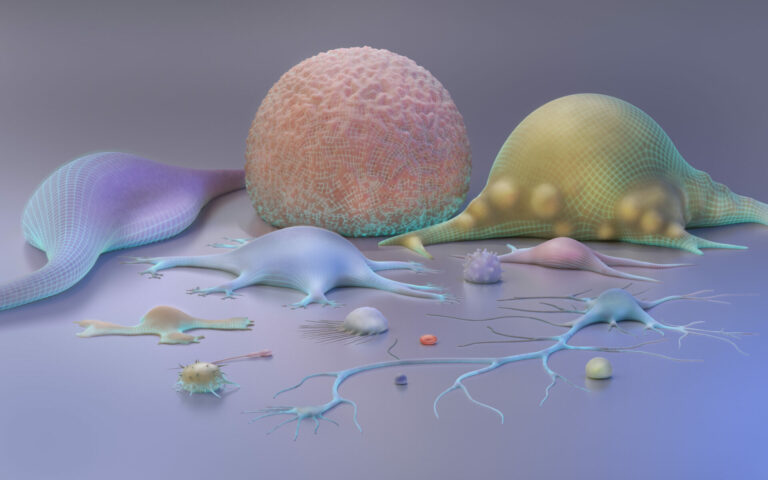
Bioengineering
AI for cells helps illuminate their identity
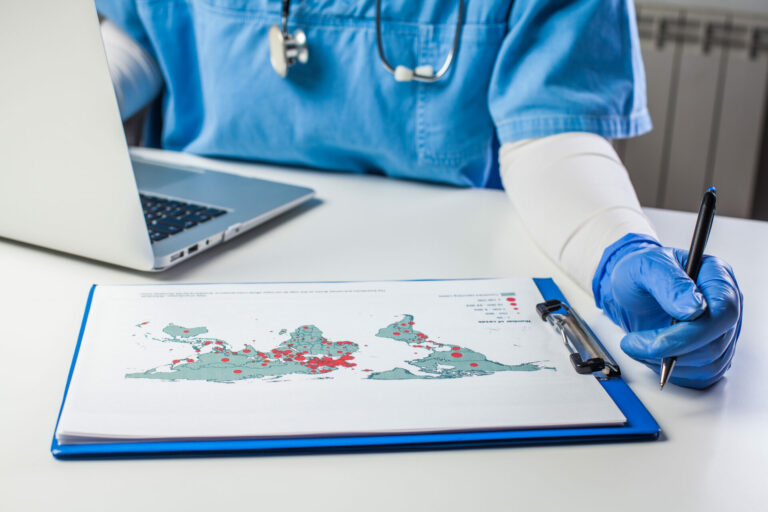
Applied Mathematics and Computational Sciences
Global look at sex differences in young people's mortality
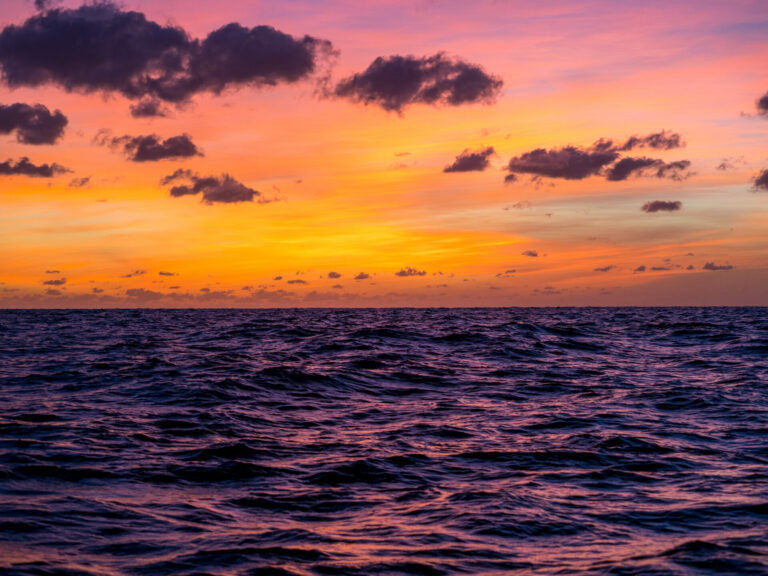
Applied Mathematics and Computational Sciences
Going likelihood-free with neural networks
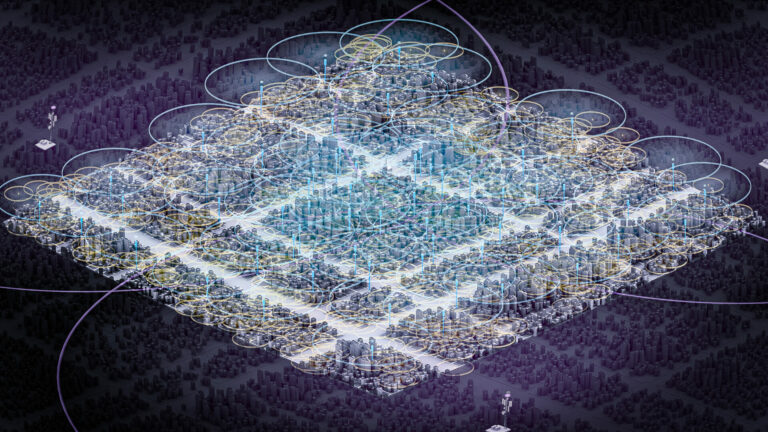
Applied Mathematics and Computational Sciences
A simple solution for frequency sharing
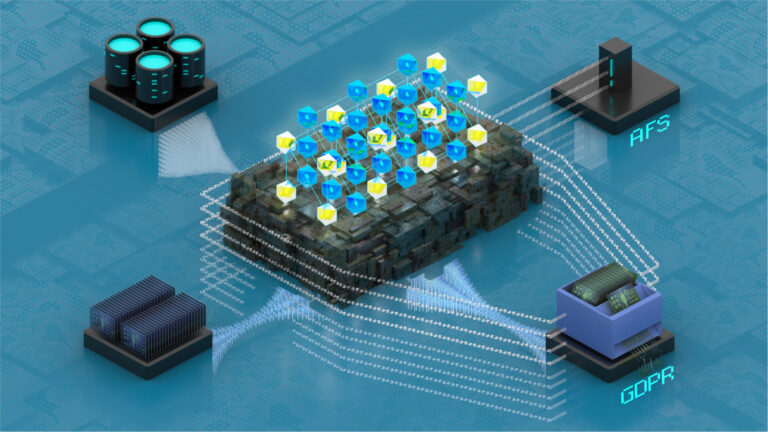
Bioengineering