Statistics
Reining in computational complexity
A more efficient approach to modeling spatial data involving thousands of variables keeps computation time in check.
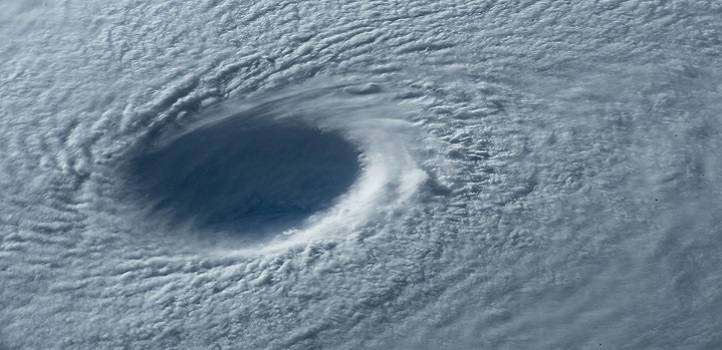
With the rapid expansion of worldwide climate monitoring networks, more data is being collected at higher resolution and at more locations than ever before. While such a wealth of data promises unprecedented insight into climate behavior and could vastly improve our ability to predict the frequency and severity of future events, it also presents an enormous computational challenge. Computing power can be repeatedly multiplied to tackle such problems; however, as the number of observation sites and variables expands into the thousands and tens of thousands, this approach will soon fall behind the exponential pace of the data explosion.
To address this looming dilemma, Jian Cao, a doctoral student in Marc Genton’s research group at KAUST, has now developed an approach that reduces the computational complexity of such problems by a factor of 50 or more.
“Our goal was to develop a more effective method for computing multivariate normal probabilities, which appear frequently in statistical models used to analyze extreme events,” says Cao.
One of the key motivations behind Cao’s work was the limited number of variables, or dimensions, that can be handled by commonly used statistical platforms.
“Most current statistical software can only handle hundreds of dimensions,” explains Cao. “The R package, for example, is the most prevalent statistical software used for computing multivariate normal probabilities, and it can only accept 1,000 dimensions.”
Cao applied a combination of modern statistical techniques to break down the high-dimensional problem into many low-dimensional ones. Taking a hierarchical approach, he first exploited the amenable analytical properties of the statistical covariance functions, which describe the similarity in observations at different locations, to form simpler block approximations. The blocks are then reordered for computational efficiency and a trade-off parameter is applied to set the level of accuracy versus approximation.
The result is a flexible scheme that avoids iterative computations and lowers the dimensionality of the problem to something that can be accommodated by standard software packages.
“In R, for example, with its maximum 1,000 dimensions, such calculations usually take around 20 seconds,” says Cao. “With our method, the problem can be approximated in just 0.3 seconds.”
Cao explains that this study, his first since joining KAUST, is an initial step in the development of a more comprehensive and powerful statistical platform.
“This method cannot yet provide an estimation of the approximation error, which would be a major hurdle for practitioners, but, inspired by our recent experiments, we are addressing this issue,” he says.
References
- Cao, J., Genton, M.G., Keyes, D.E., & Turkiyyah, G.M. Hierarchical-block conditioning approximations for high-dimensional multivariate normal probabilities. Statistics and Computing (2018).| article
You might also like
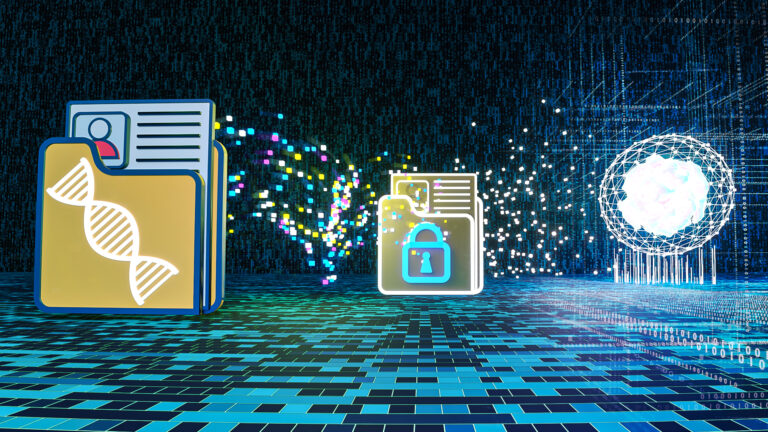
Bioengineering
Shuffling the deck for privacy
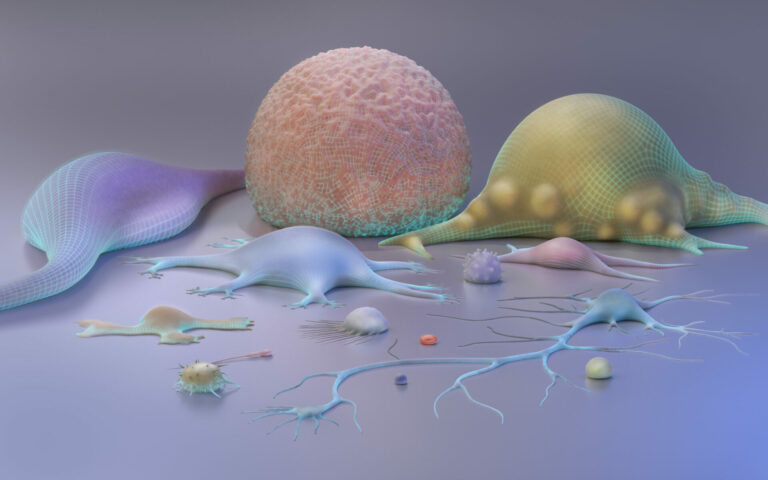
Bioengineering
AI for cells helps illuminate their identity
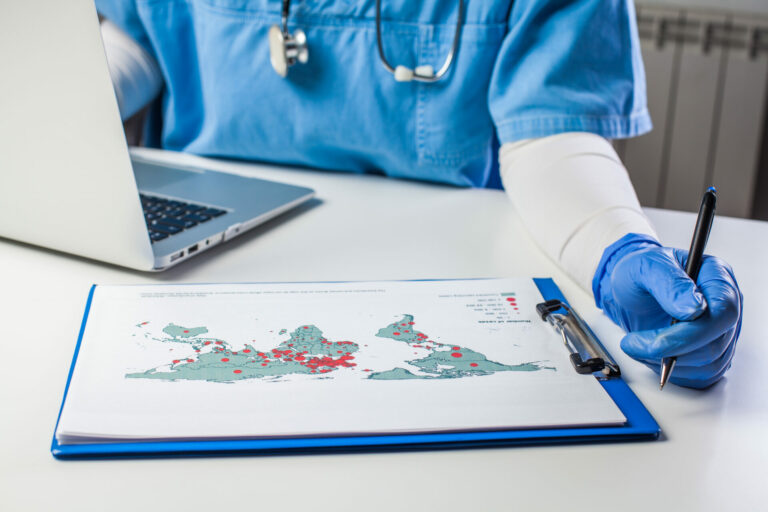
Applied Mathematics and Computational Sciences
Global look at sex differences in young people's mortality
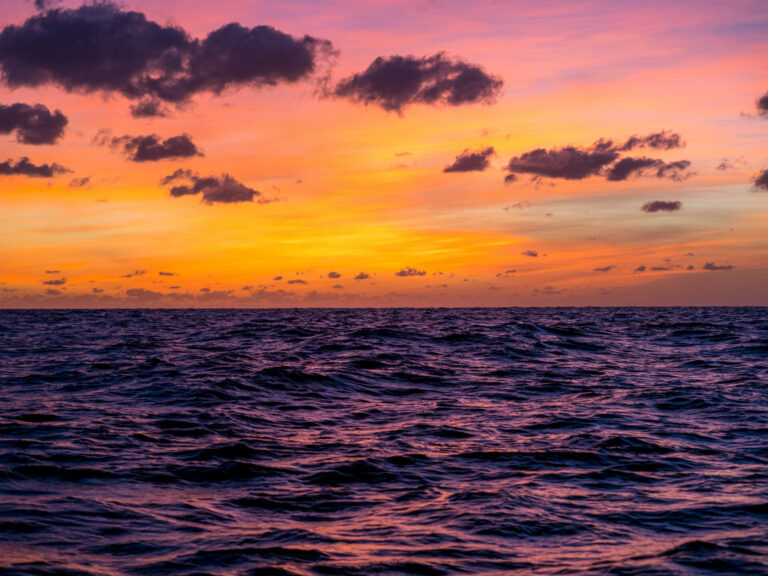
Applied Mathematics and Computational Sciences
Going likelihood-free with neural networks
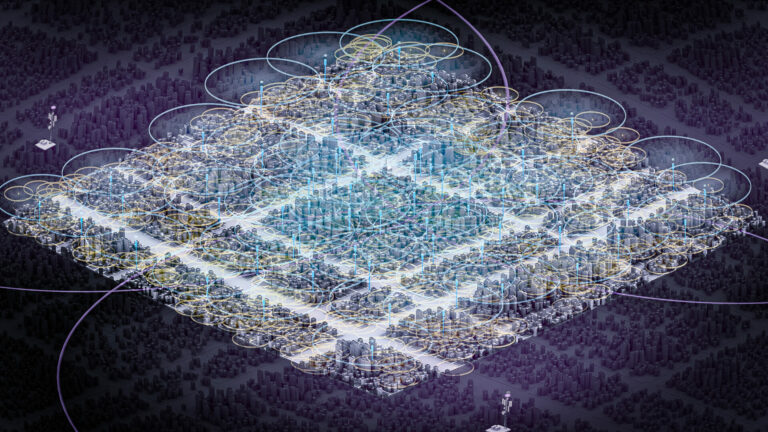
Applied Mathematics and Computational Sciences
A simple solution for frequency sharing
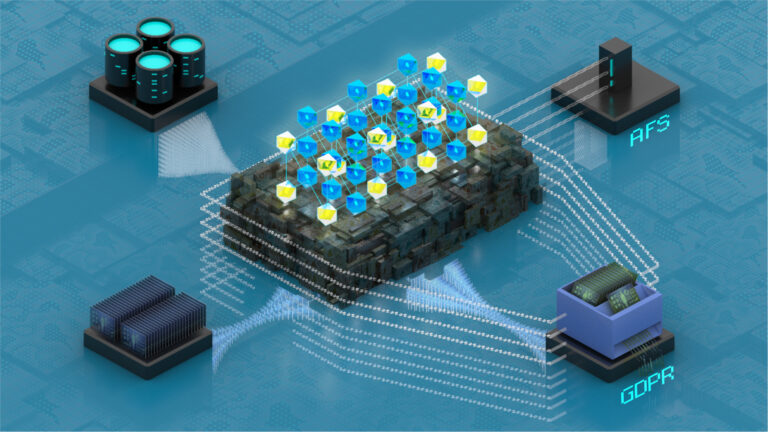
Bioengineering
Safeguarding the right to be forgotten
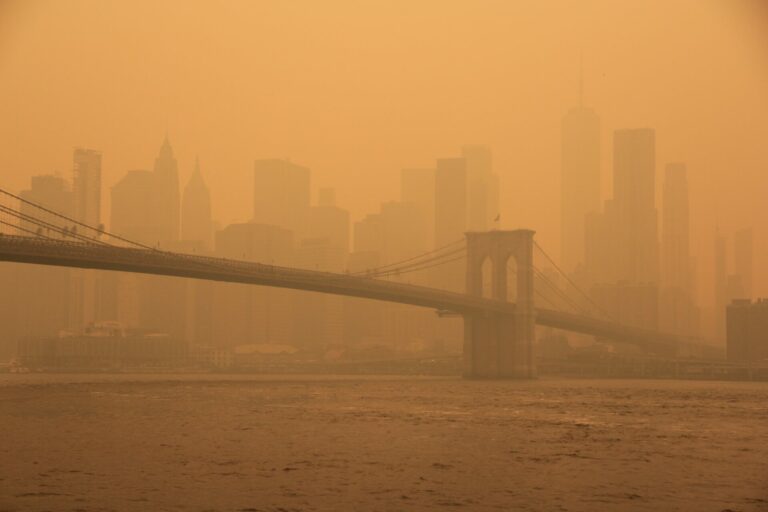
Applied Mathematics and Computational Sciences
Neural networks give deeper insights
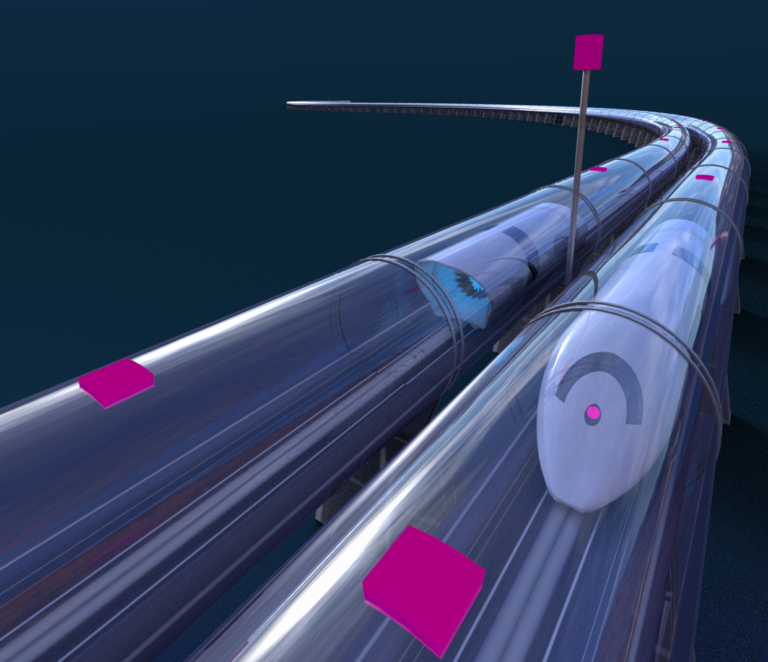
Applied Mathematics and Computational Sciences