Statistics
Dataset size counts for better predictions
A statistical technique for modeling large datasets improves interpretation of climate and environmental data.
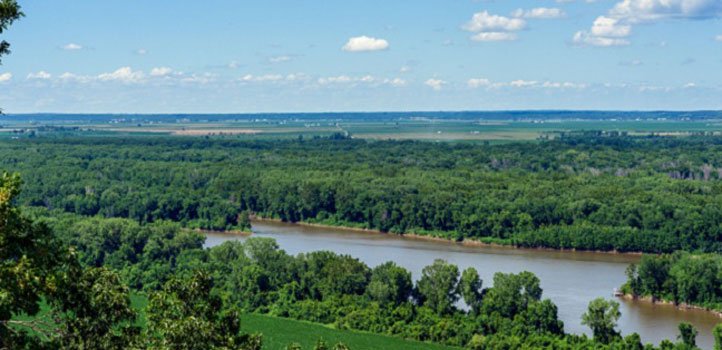
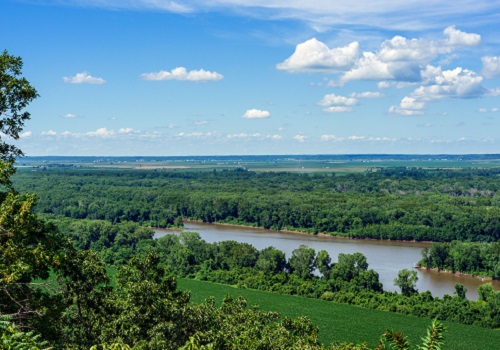
The model was applied to a spatial dataset of the Mississippi River basin in the United States to improve understanding of hydrological processes and climate variability.
© Ian Dagnall / Alamy Stock Photo
A new statistical tool for modeling large climate and environmental datasets that has broad applications—from weather forecasting to flood warning and irrigation management—has been developed by researchers at KAUST.
Climate and environmental datasets are often very large and contain measurements taken across many locations and over long periods. Their large sample sizes and high dimensionality introduce significant statistical and computational challenges. Gaussian process models used in spatial statistics, for example, face considerable difficulty due to the prohibitive computational burden and rely on subsamples or analyze spatial data region by region.
Ying Sun and her PhD student Huang Huang developed a new method that uses a hierarchical low-rank approximation scheme to resolve the computational burden, providing an efficient tool for fitting Gaussian process models to datasets that contain large quantities of climate and environmental measurements.
“One advantage of our method is that we apply the low-rank approximation hierarchically when fitting the Gaussian process model, which makes analyzing large spatial datasets possible without excessive computation,” explains Huang. “The challenge, however, is to retain estimation accuracy by using a computationally efficient approximation.”
Traditional low-rank methods are usually computationally fast, but often inaccurate. The researchers, therefore, made the low-rank approximation hierarchical, ensuring that the covariance matrix used to fully characterize dependence in the spatial data is not low rank: this makes it is as fast as traditional methods while significantly improving the accuracy.
To evaluate their model’s performance, they undertook numerical analysis and simulations and found the model performs much better than the most commonly used methods. This ensures that credible inferences can be made from real-world datasets.
The model was applied to a spatial dataset of two million soil-moisture measurements from the Mississippi River basin in the United States. They were able to fit a Gaussian process model to understand the spatial variability and predict values at unsampled locations. This led to a better understanding of hydrological processes, including runoff generation and drought development, and climate variability for the region.
“Our research provides a powerful tool for the statistical inference of large spatial data, says Sun. “And when exact computations are not possible, environmental scientists could use our methodology to handle large datasets instead of only analyzing subsamples. This makes it a practical and attractive technique for very large climate and environmental datasets.”
References
-
Huang, H. & Sun, Y. Hierarchical low rank approximation of likelihoods for large spatial datasets. arXiv:1605.08898 (2017).| article
You might also like
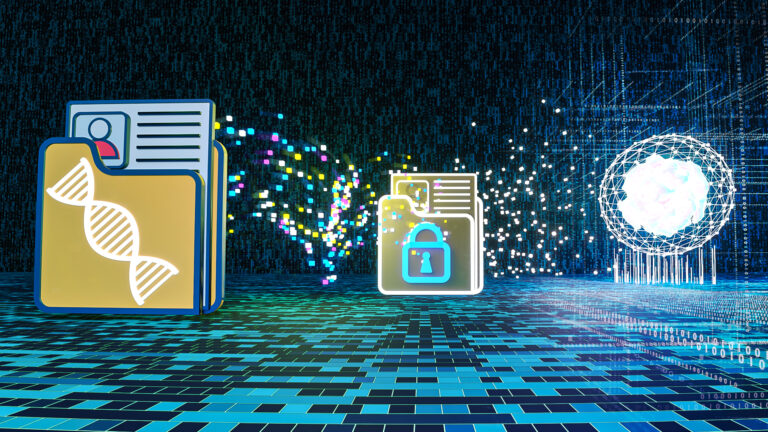
Bioengineering
Shuffling the deck for privacy
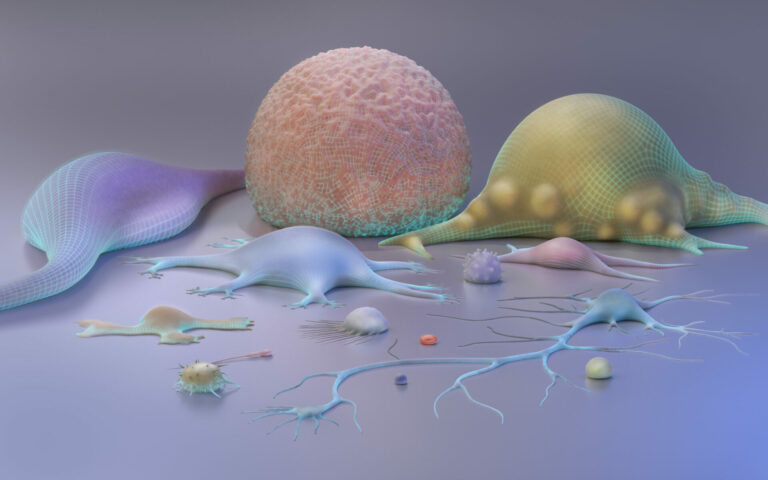
Bioengineering
AI for cells helps illuminate their identity
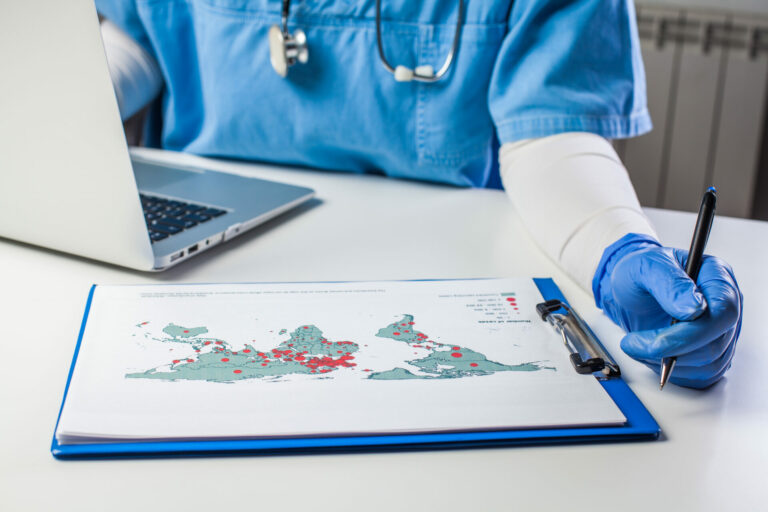
Applied Mathematics and Computational Sciences
Global look at sex differences in young people's mortality
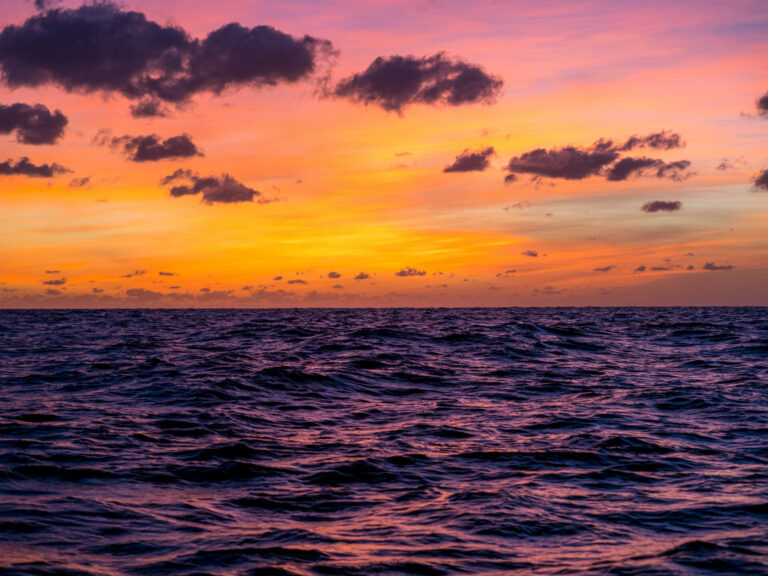
Applied Mathematics and Computational Sciences
Going likelihood-free with neural networks
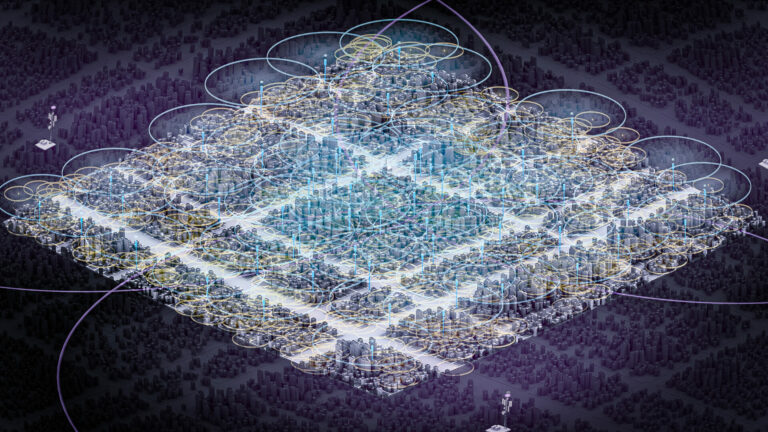
Applied Mathematics and Computational Sciences
A simple solution for frequency sharing
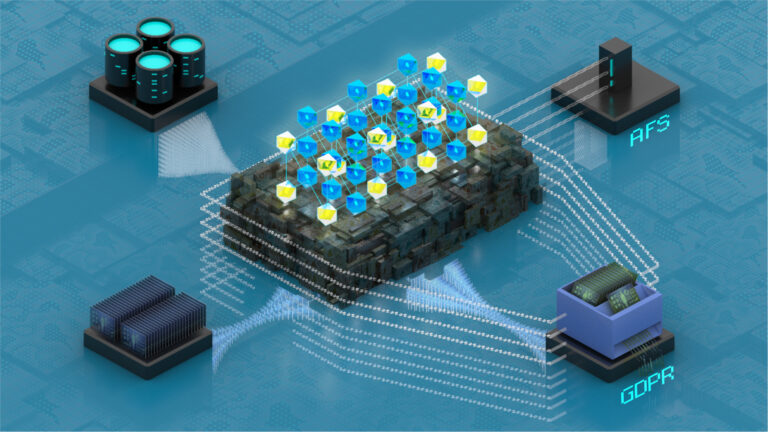
Bioengineering
Safeguarding the right to be forgotten
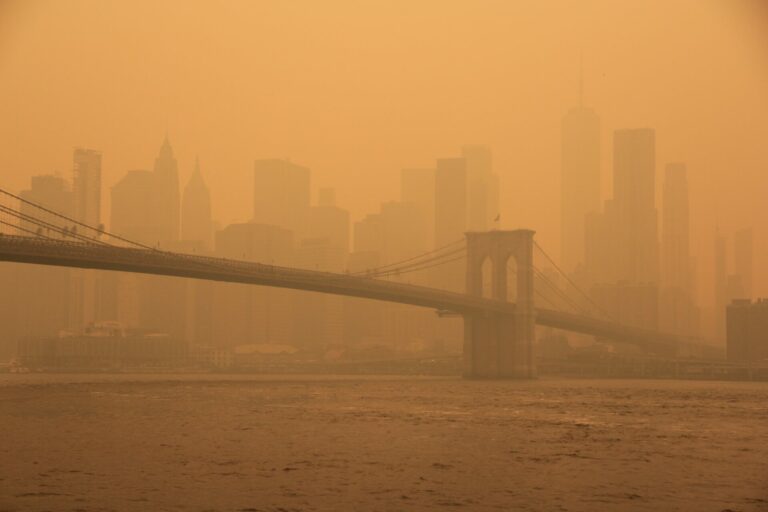
Applied Mathematics and Computational Sciences
Neural networks give deeper insights
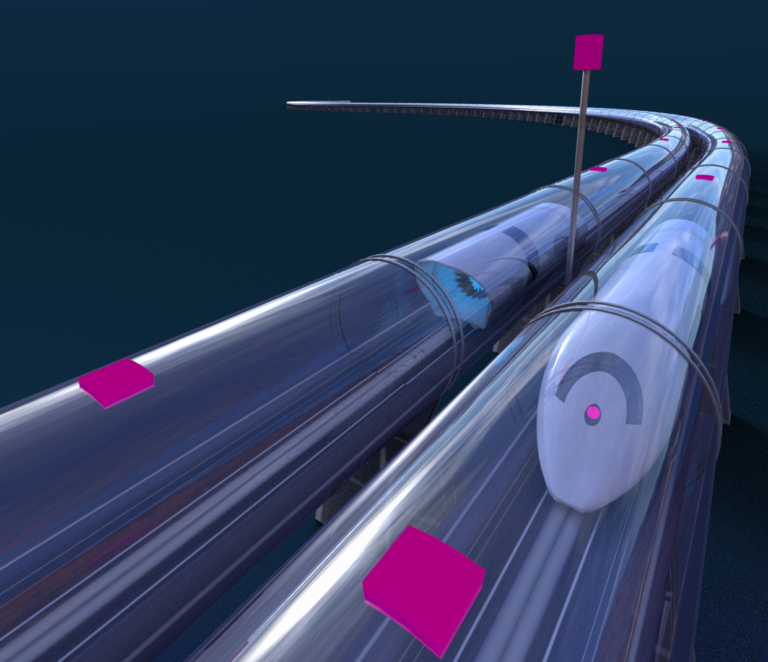
Applied Mathematics and Computational Sciences