Statistics
Letting the data speak for itself
A new statistical approach for environmental measurements lets the data determine how to model extreme events.

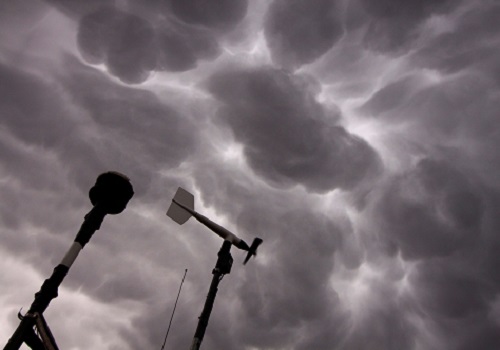
Modeling of wind speed across a region is based on a handful of monitoring stations.
© Alamy Ryan McGinnis
Modeling environmental data, such as regional wind speed or temperature, is a complicated business. To model data statistically requires significant assumptions about its behavior over time and space—yet arriving at those assumptions requires an understanding of the data that can generally only be obtained by modeling. It’s a catch-22 that presents a major obstacle to progress in large-scale environmental and climate modeling, particularly for extreme events.
KAUST researcher Raphaël Huser, in collaboration with colleagues from France and Switzerland, has now developed a modeling framework that lets the data define its own behavior around extreme events without the need for restrictive predetermined assumptions.
“Environmental extremes, such as extreme wind gusts, floods, or heat waves, are often spatially dependent,” explains Huser. “That is, two neighboring measurement stations may, and often do, experience extreme events simultaneously. But does this dependence stabilize or weaken as the event becomes more extreme? Classical statistical models require the nature of this extremal dependence to be defined before modeling, but because extreme events are scarce, it can be very difficult if not impossible to correctly guess the dependence class in advance.”
Classical statistical models that account for extreme events are known as asymptotic models. The choice of asymptotic extremal dependence type determines how the model extrapolates to events even more extreme that those present in the data. It comes bundled with other implied assumptions that are not always realistic environmentally, with the result that such models may incorrectly assess the likelihood of future extreme events.
“We developed a suite of flexible geostatistical ‘subasymptotic’ models using a general Gaussian basis that captures both types of asymptotic dependence,” says Huser. “Our models are more flexible and easier to use, especially for higher-dimensional data collected at many monitoring stations.”
Through simulations of measured wind speeds, Huser’s team showed that their Gaussian-scale mixtures model can accurately estimate the extremal dependence type. It also outperforms other typical models over a range of performance metrics with a good fit to the data and more realistic spatial prediction of extreme wind speeds at unobserved locations.
“The most important result of our work is that we no longer need to fix the asymptotic dependence class in advance but can let the data speak for itself,” says Huser. “This model is applicable to a wide range of environmental data and will help improve our modeling and prediction of extreme events.”
References
-
Huser, R., Opitz, T. & Thibaud, E. Bridging asymptotic independence and dependence in spatial extremes using Gaussian scale mixtures. Spatial Statistics 21A, 166-186 (2017).| article
You might also like
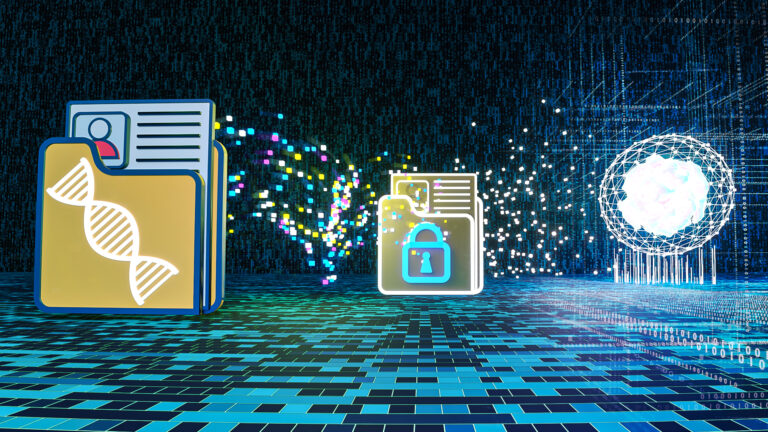
Bioengineering
Shuffling the deck for privacy
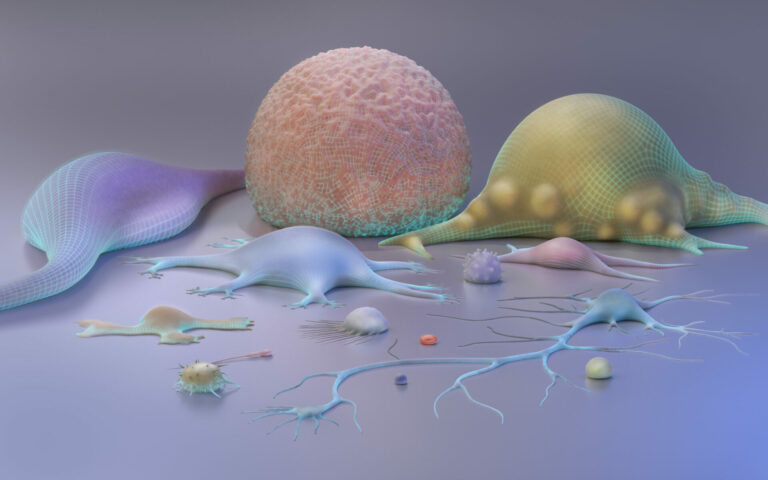
Bioengineering
AI for cells helps illuminate their identity
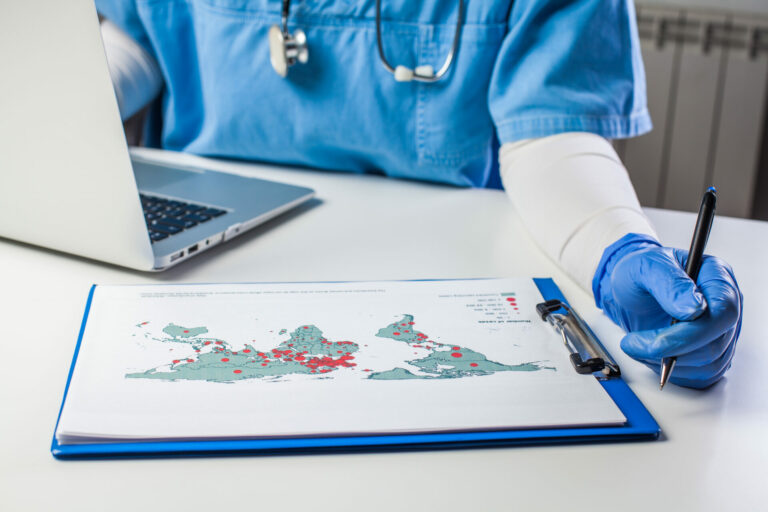
Applied Mathematics and Computational Sciences
Global look at sex differences in young people's mortality
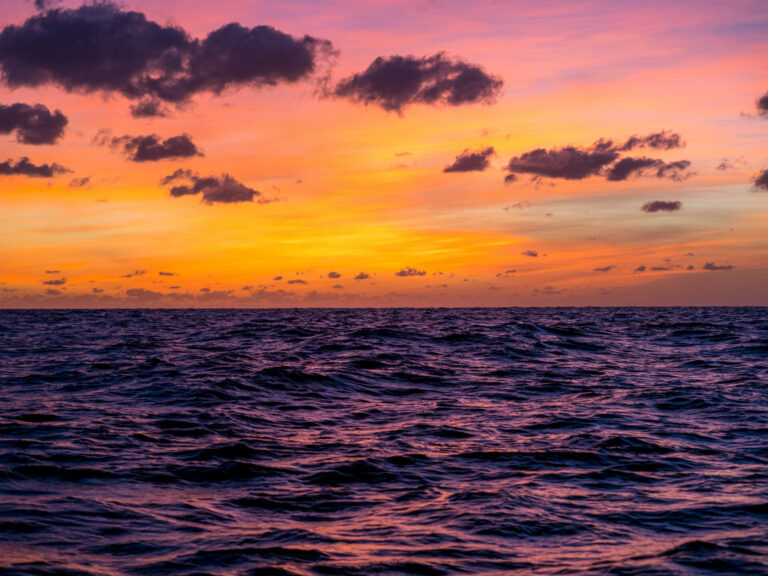
Applied Mathematics and Computational Sciences
Going likelihood-free with neural networks
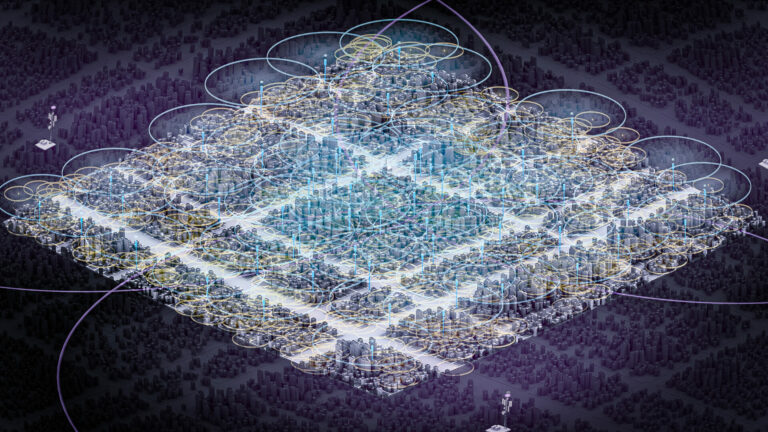
Applied Mathematics and Computational Sciences
A simple solution for frequency sharing
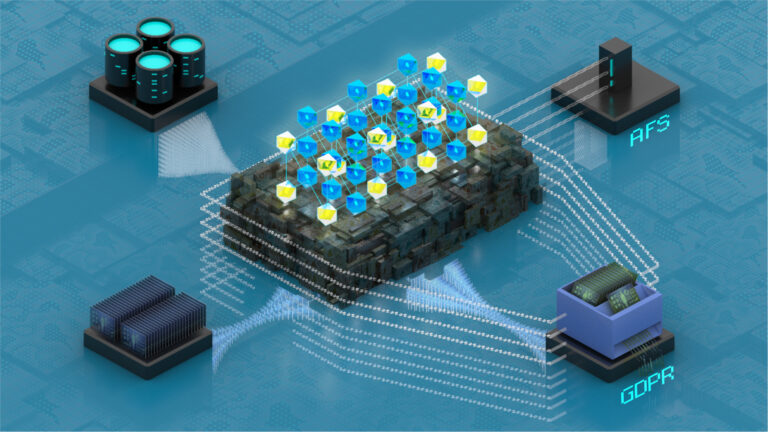
Bioengineering
Safeguarding the right to be forgotten
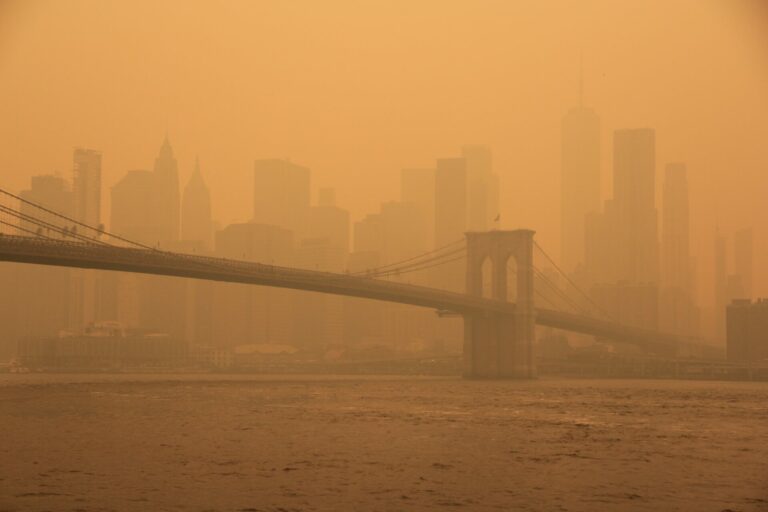
Applied Mathematics and Computational Sciences
Neural networks give deeper insights
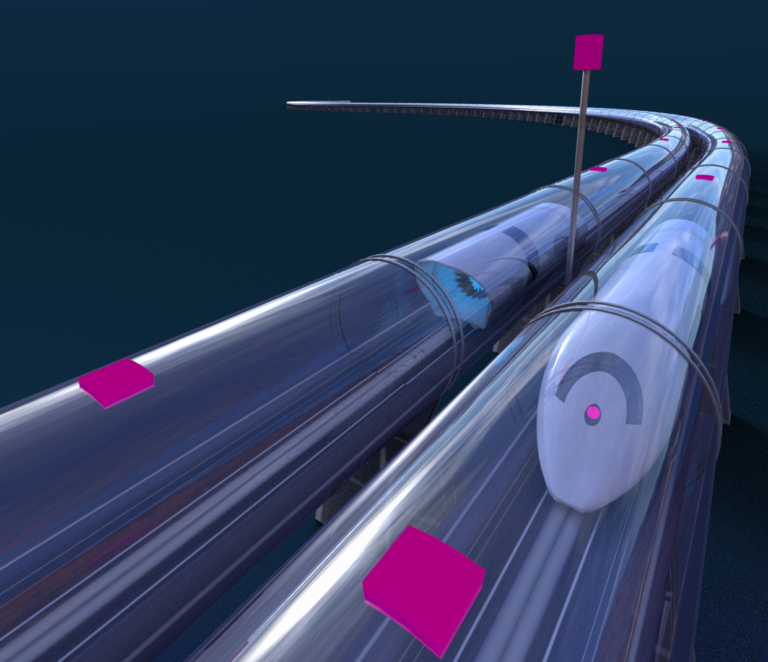
Applied Mathematics and Computational Sciences