Applied Mathematics and Computational Sciences
Giving power to demand-driven electricity
A new approach to analyzing household electricity: smart-meter data could improve the performance and efficiency of national power grids.


Accurately predicting power demand is critical to the reliable and sustainable operation of electricity grids.
© Alamy stock photo / Simon Belcher
Power generators face the constant challenge of matching the amount of power produced at any given time with the demand from consumers. Excess generation is wasteful and expensive, while undergeneration can cause brownouts. For this reason, accurately predicting power demand hours or even days in advance is critical for the reliable and sustainable operation of the electricity grid.
KAUST Distinguished Professor of Applied Mathematics and Computational Science Marc Genton and his colleagues have developed an effective way to use the enormous amount of power-usage data reported by household energy meters to more accurately forecast power demand1.
In many countries, households and businesses are fitted with smart power meters that report power consumption in short intervals to the electricity network. For consumers, smart meters make it possible to monitor electricity usage in real time, and often the data they collect can help to identify usage habits and issues. For generators, smart-meter data represent a potential gold mine of information with which to better predict demand and operate power-generation facilities more efficiently. However, the sheer volume and complexity of data collected by household smart meters on a city or regional scale makes using the data challenging.
“Smart-meter data can help generators understand individual electricity consumption behavior,” explained Genton. “However, the heterogeneity, high level of noise and volume of data require the development of new forecasting algorithms. Our flexible machine-learning algorithm is designed to handle the specific properties of smart-meter data.”
One of the main difficulties in effectively analyzing household power-consumption data is the noise caused by rapid changes in energy usage as appliances are switched on and off. In addition, the enormous variety in usage patterns among households and the volume of data that need to be analyzed presents tremendous obstacles for traditional analytical approaches.
Genton and his former postdoc Souhaib Ben Taieb and KAUST’s Raphaël Huser collaborated with Rob J. Hyndman from Monash University in Australia to develop a machine-learning algorithm that computes one-day-ahead probabilistic forecasts for each meter using recent time-series data and many predictor variables, including date and weather parameters. In their demonstration study, the team used the distributed computing environment at KAUST to analyze half-hourly smart-meter data recorded by more than 3,000 households over 1.5 years.
“Our algorithm has many advantages, including flexibility, automatic variable selection and interpretability,” said Genton, “and we showed that it can provide more accurate probabilistic forecasts compared to existing state-of-the-art forecasting methods.”
References
-
Ben Taieb, S., Huser, R., Hyndman, R.J. & Genton, M.G. Forecasting uncertainty in electricity smart meter data by boosting additive quantile regression. IEEE Transactions on Smart Grid 7, 2448–2455 (2016).| article
You might also like
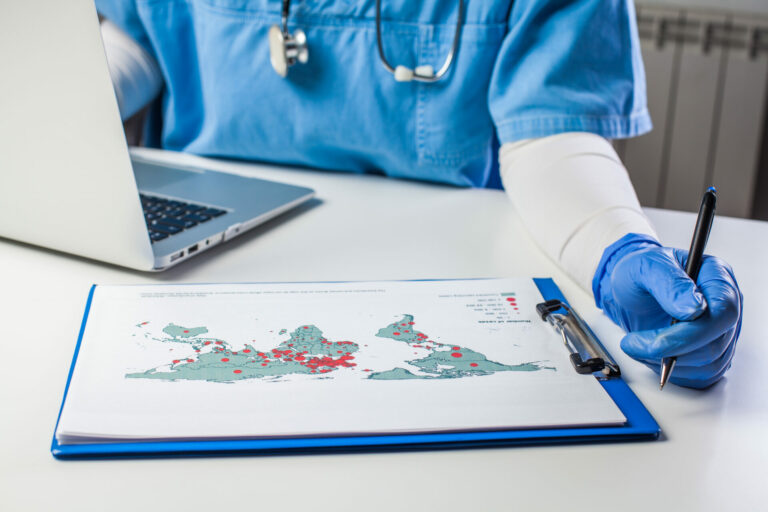
Applied Mathematics and Computational Sciences
Global look at sex differences in young people's mortality
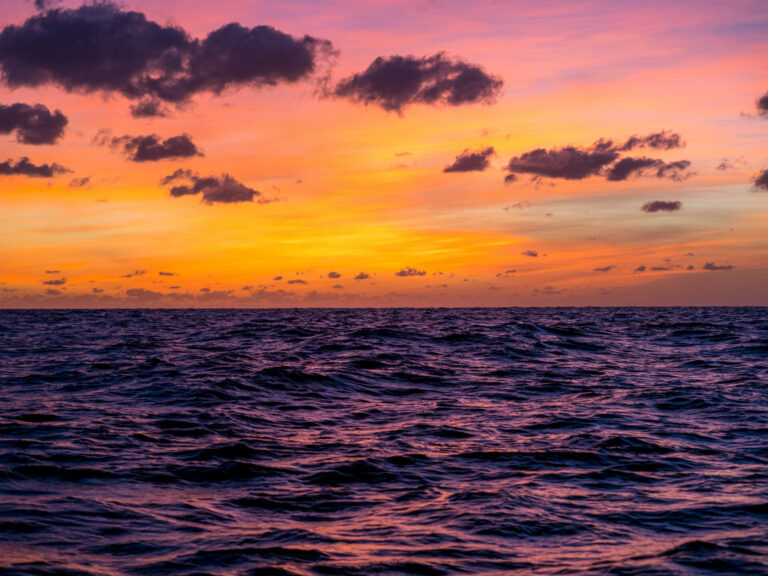
Applied Mathematics and Computational Sciences
Going likelihood-free with neural networks
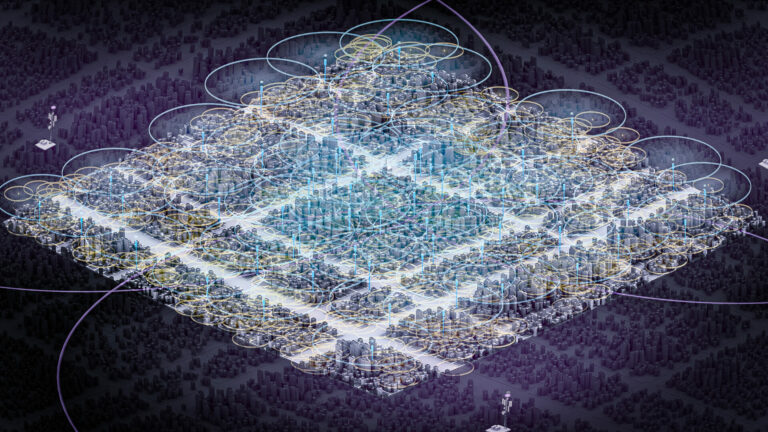
Applied Mathematics and Computational Sciences
A simple solution for frequency sharing
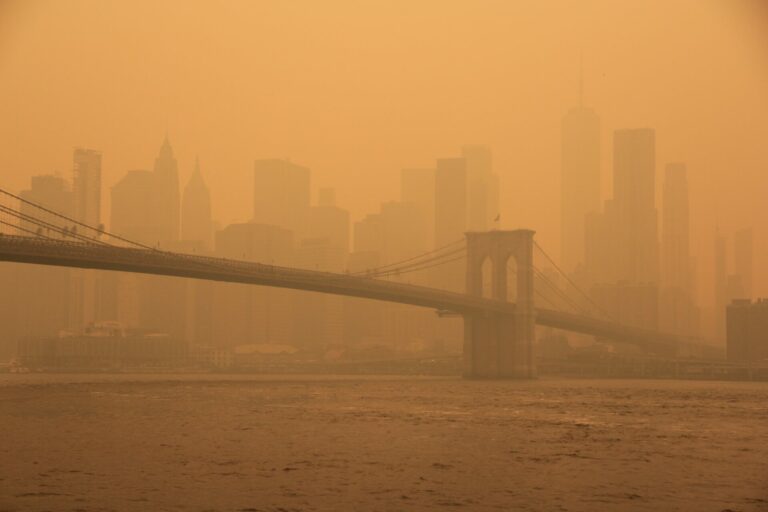
Applied Mathematics and Computational Sciences
Neural networks give deeper insights
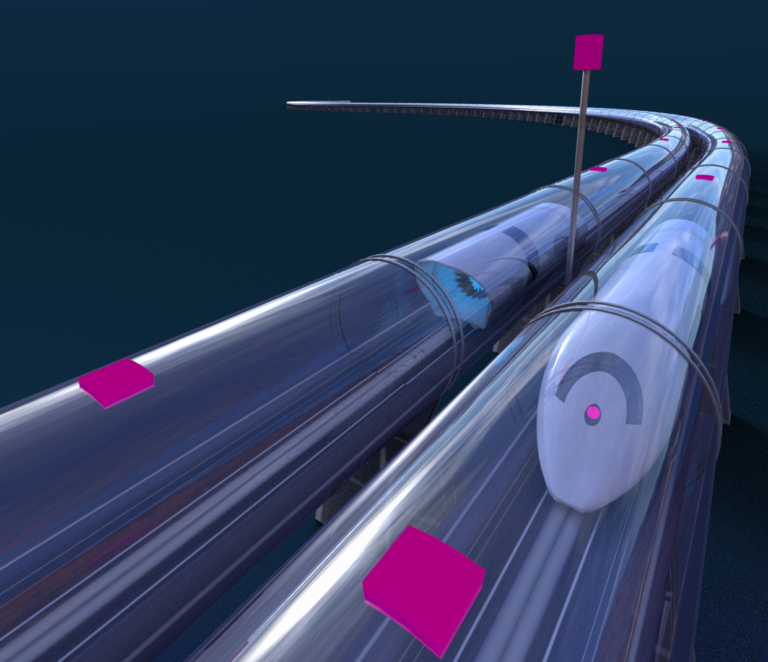
Applied Mathematics and Computational Sciences
Staying online in the high-speed tube of the future
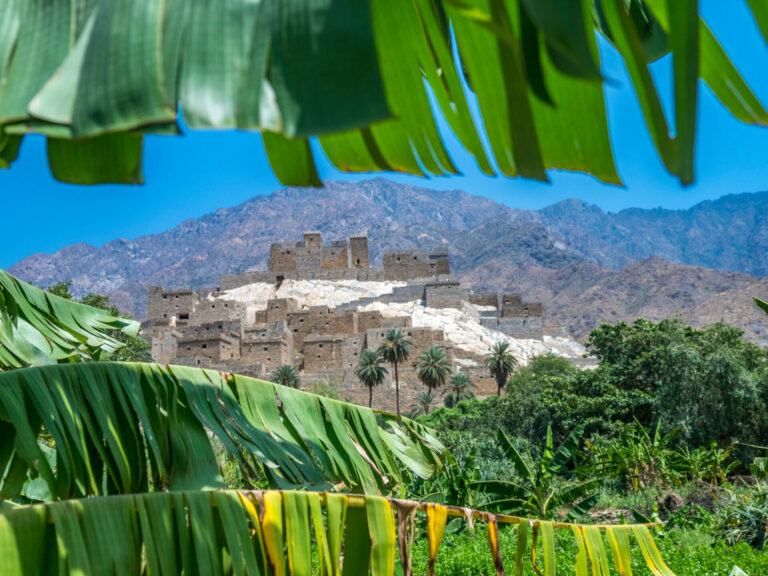
Applied Mathematics and Computational Sciences
Measuring the impact of desert greening
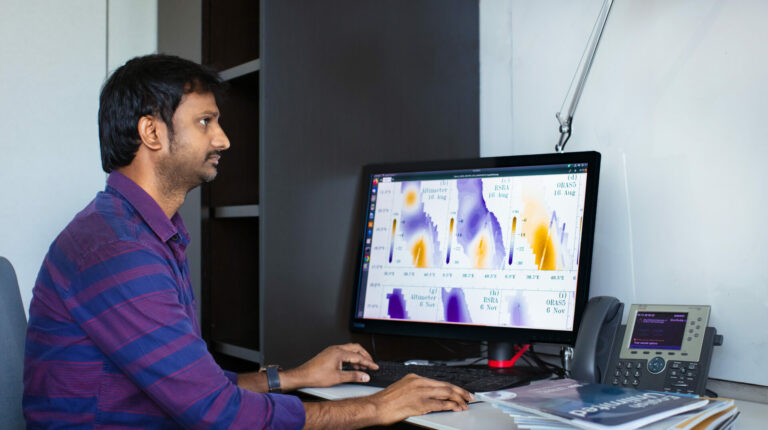
Applied Mathematics and Computational Sciences
Data-driven regional ocean models essential for planning
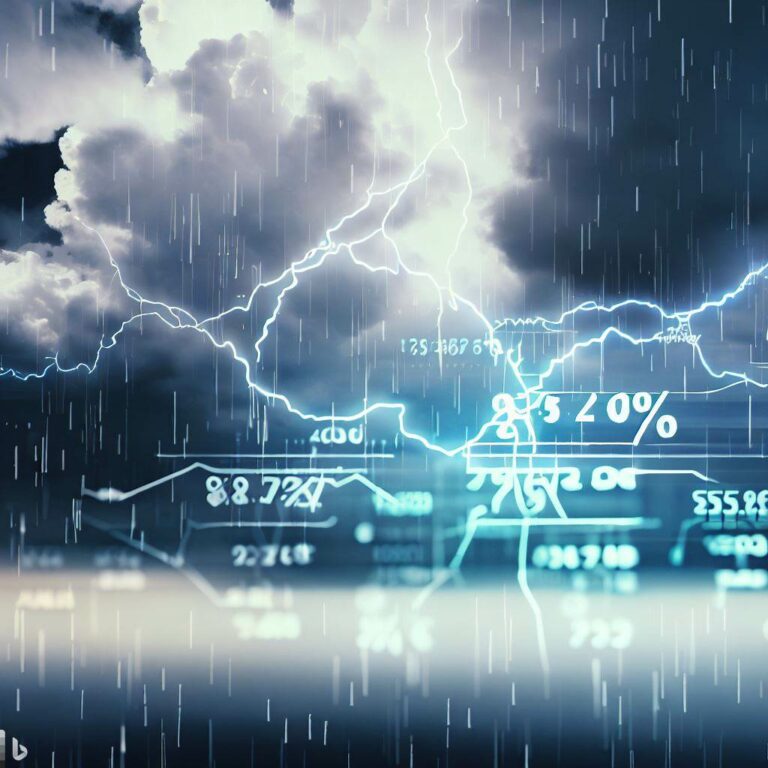
Applied Mathematics and Computational Sciences