Computer Science
Exploring protein form to find function
Existing systems for predicting protein structure are outperformed by a newly developed method.
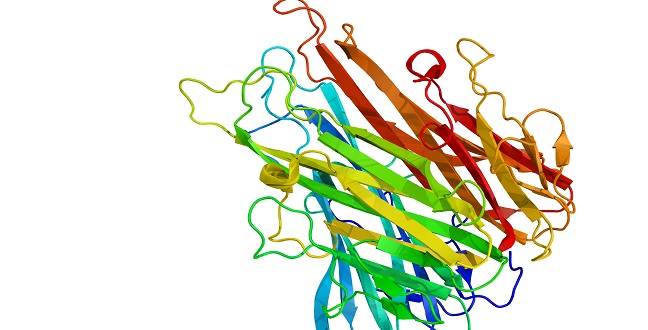
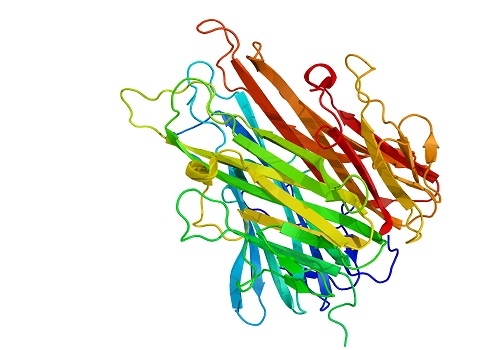
A new method developed by KAUST researchers improves the prediction of 3-D protein structures.
© 2016 Alamy DGG1YK
The prediction of protein structures has become easier with a method developed by KAUST researchers that outperforms state-of-the-art techniques. Resulting insight into proteins could help to identify drug targets and develop therapeutics.
Proteins have multiple structure levels, the most basic of which is a string of building blocks called amino acids that are linked in a protein-specific sequence. The complete amino acid chains fold into 3-D structures that are essential for protein function. The 3-D structure of a protein tells us about proteins’ roles in cells, but determining this structure is remarkably difficult.
“Searching for protein 3-D structure from scratch is difficult due to the huge search space,” explained Xin Gao from the KAUST Computational Bioscience Research Center. “The most promising way to predict protein structures is by homology modeling, which is based on the observation that homologous proteins have similar structures.”
Homology modeling looks for similarities between the query protein and thousands of proteins with known 3-D structures. Current systems can look for similarities in amino acid sequence through a process called sequence alignment or assess how well the amino acid sequence maps to known 3-D structures in a process called threading. However, they do not account for the entire protein sequence space (all known sequences) and the entire structure space (all known 3-D structures). Gao and colleagues developed a cross-modal method called CMsearch that integrates such information.
“CMsearch systematically and simultaneously incorporates sequence and structure features and sequence space and structure space information,” Gao said. “Its ability to consider more information in a systematic way means that CMsearch can successfully detect some remote homologs with relatively weak sequence alignment or threading scores that existing methods cannot detect.”
The researchers tested their system on a database of over 8,000 proteins. They selected some proteins to be query proteins for which only the basic sequences were known. They then asked CMsearch to predict the 3-D structures of these proteins by comparing the query protein sequences to proteins in the rest of the database.
CMsearch identified homologs for the query proteins more accurately than other currently used prediction software, demonstrating the benefit of analyzing sequence and structure space information in parallel.
The improvement that CMsearch yields in structural predictions provides a basis for greater functional insights, with the potential for considerable biomedical benefits.
“The function of a protein is determined by its 3-D structure,” said Gao. “Therefore, determining protein structures can help us to understand how proteins work and lead to therapeutic solutions for various diseases.”
References
-
Cui, X., Lu, Z., Wang, S., Wang, J. J.-Y. & Gao, X. CMsearch: simultaneous exploration of protein sequence space and structure space improves not only protein homology detection but also protein structure prediction. Bioinformatics 32,i332-i340.|article
You might also like
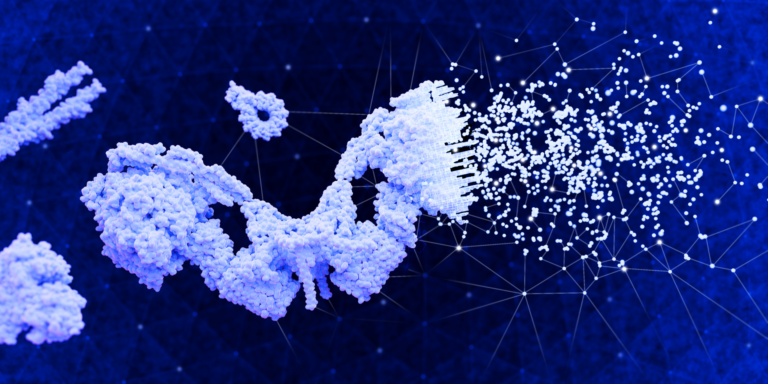
Bioengineering
AI tool predicts function of unknown proteins
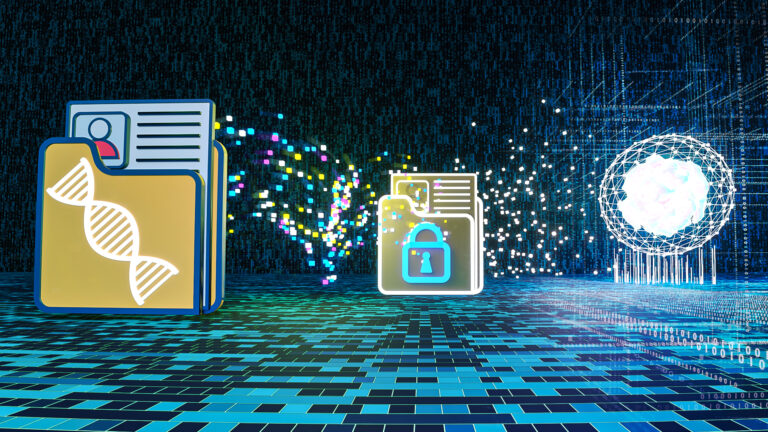
Bioengineering
Shuffling the deck for privacy
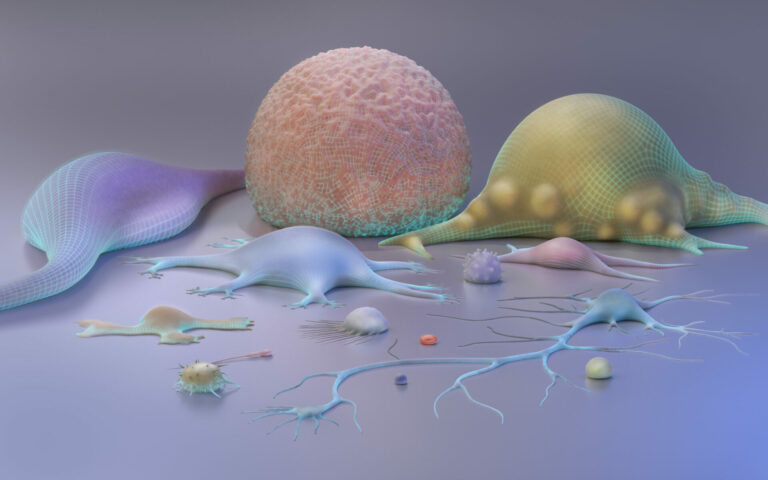
Bioengineering
AI for cells helps illuminate their identity
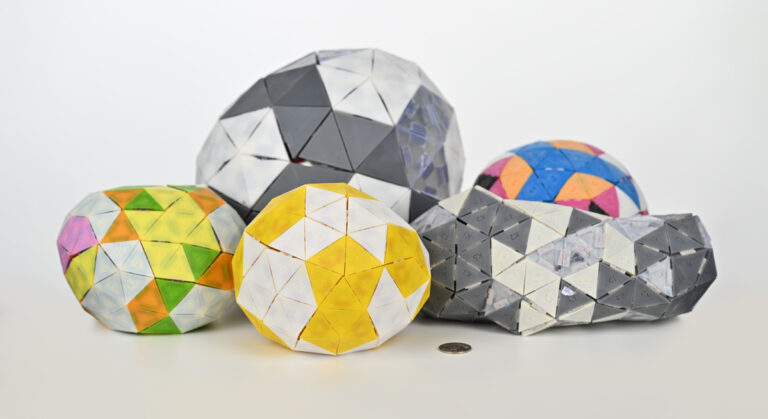
Computer Science
Dr. KID turns biological models into fun puzzles
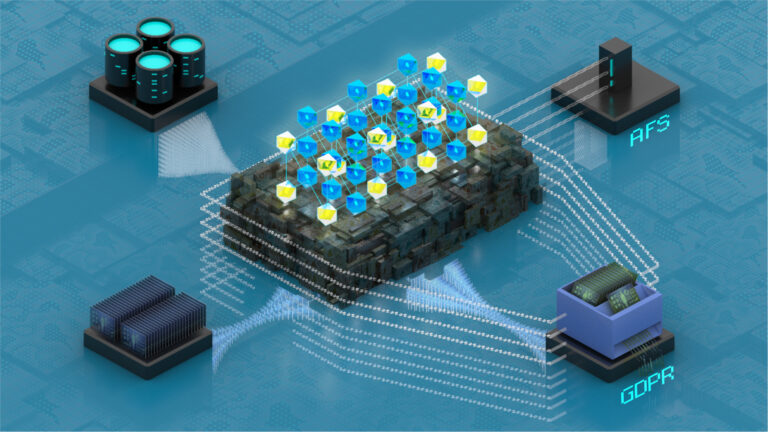
Bioengineering
Safeguarding the right to be forgotten
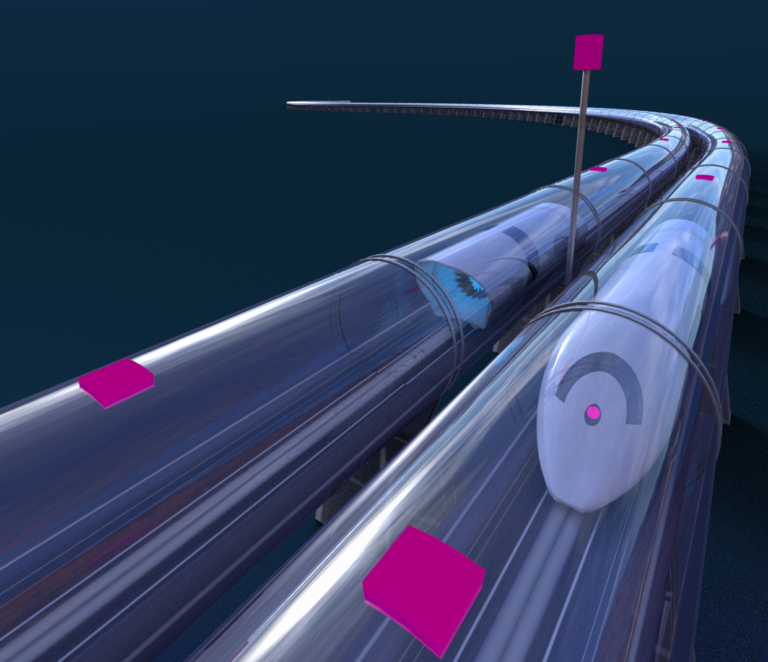
Applied Mathematics and Computational Sciences
Staying online in the high-speed tube of the future
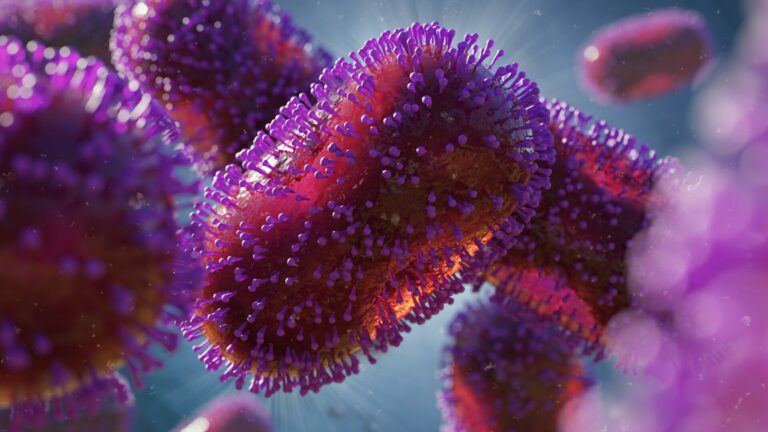
Bioengineering
Lab-grown skin helps unlock secrets of mpox virus infection
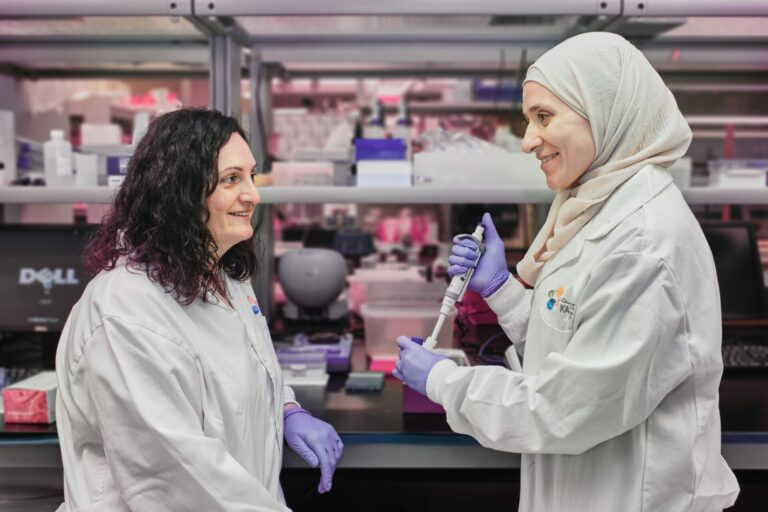
Bioengineering