Statistics
A clearer view of rainfall patterns
Improved random modeling allows scientists to generate realistic patterns of high-frequency rainfall.
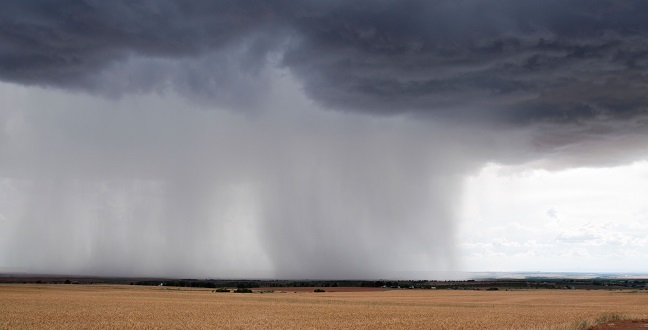
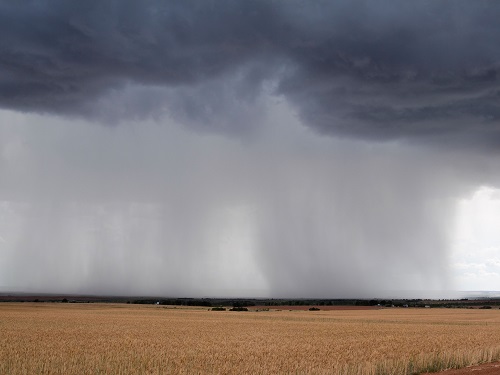
A statistical model developed by KAUST researchers enables the generation of realistic rainfall patterns that can help inform agricultural and land-use management.
© 2016 Alamy BP4W8Y
A thorough understanding of rainfall patterns is vital for the hydrological and agricultural models that are used to project flooding, stream flow and crop growth around the world. A study at KAUST has generated an accurate method for modeling precipitation events based on data from rain gauges in the United States1.
Rainfall data are collected on different space-time scales depending on the instruments used. For example, satellites gather information on precipitation down to a resolution of around one square kilometer over days, while an individual rain gauge collects data from around one square meter as often as once every minute. The intermittent and random nature of rainfall events means that modeling a precipitation field is challenging, and the best models for simulating where and when rain might fall require a probabilistic element.
Ying Sun from the University’s Computer, Electrical and Mathematical Science and Engineering Division and Michael Stein at the University of Chicago (U.S.) have developed a novel precipitation occurrence model that generates realistic patterns of rainfall events at multiple locations every 15 minutes.
The researchers used high-quality data gathered at rain gauges in Virginia, Maryland and North Carolina to develop their “stochastic” model. Stochastic modeling allows for random fluctuations in multiple simulations and provides a collection of possible outcomes that can help scientists understand rainfall variability across space and time.
“Our model, a truncated non-Gaussian distribution, allows for areas to have zero rainfall and gives us a higher chance of simulating extreme values than in previous models,” said Sun. Sun’s model also uses a “random field” that connects all the sites and describes the spatial and temporal patterns, such as the tendency for one site to experience rainfall a few hours after another. “For example, if five sites out of 10 have rain, the random field model decides which five sites have rain and how long it rains for at each site,” she said.
“I used data detailing rain events at 15 minute intervals over three years to estimate the unknown parameters in my model, and I then generated multiple simulations from the estimated model,” explained Sun. “Because it includes more detail on the spatio-temporal patterns of rainfall events, our model produces more realistic rainfall occurrence patterns than other commonly used models.”
The next challenge for Sun is to generate simulations of rainfall amounts for multiple sites so that model users can study the impact of both light and heavy rain.
References
-
Sun, Y. & Stein, M.L. A stochastic space-time model for intermittent precipitation occurrences. The Annals of Applied Statistics 9, (2015).| article
You might also like
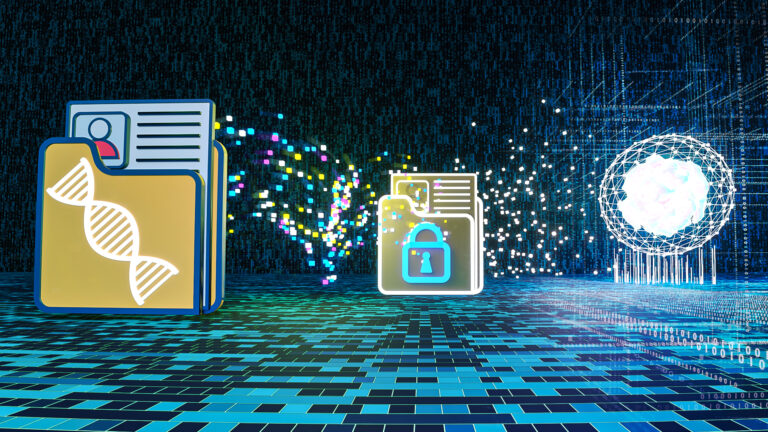
Bioengineering
Shuffling the deck for privacy
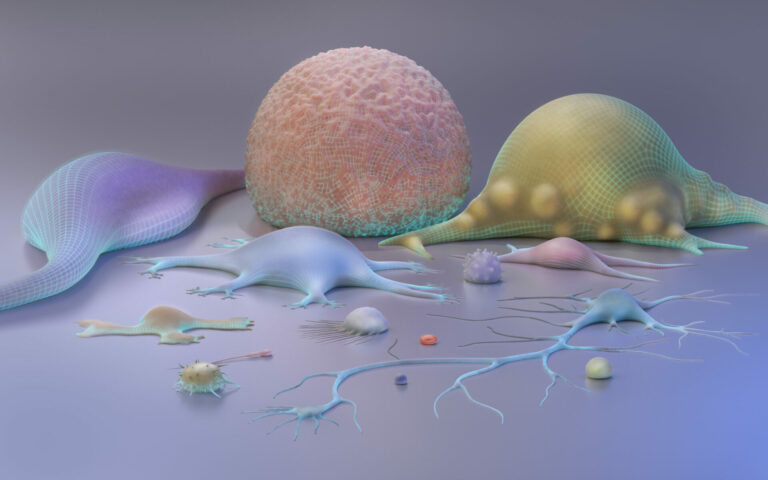
Bioengineering
AI for cells helps illuminate their identity
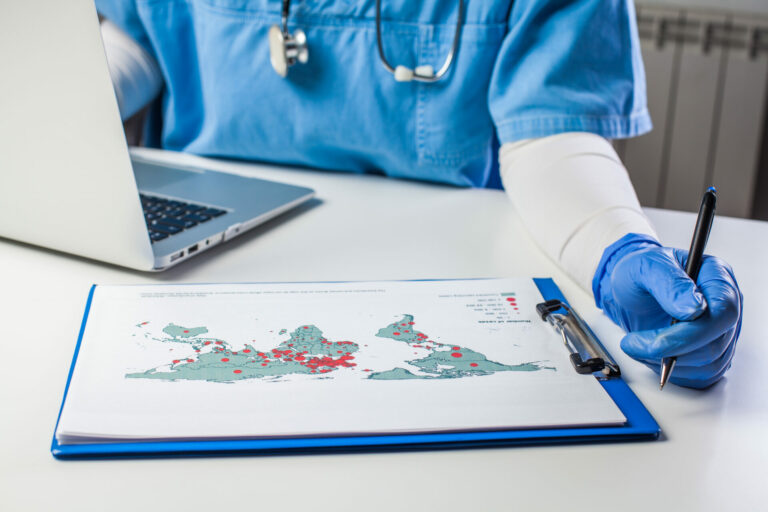
Applied Mathematics and Computational Sciences
Global look at sex differences in young people's mortality
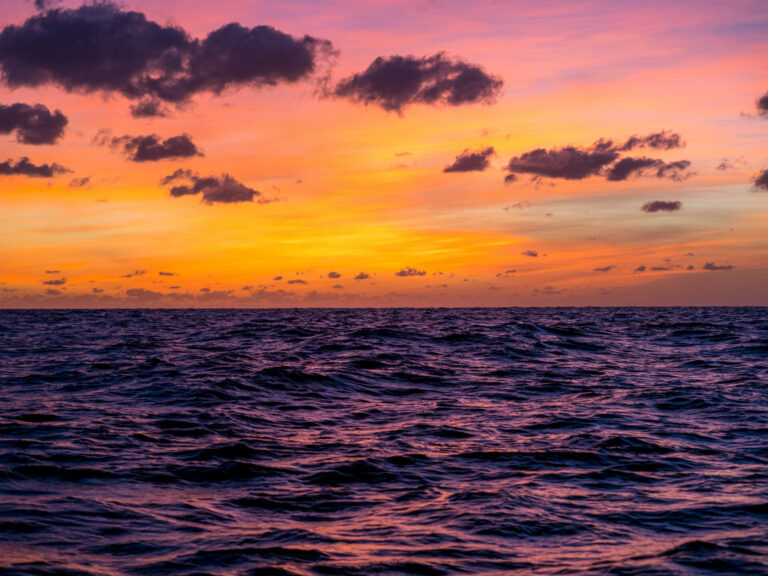
Applied Mathematics and Computational Sciences
Going likelihood-free with neural networks
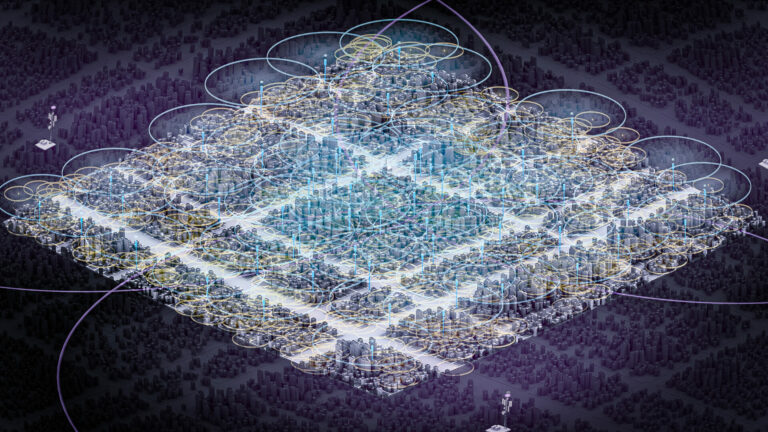
Applied Mathematics and Computational Sciences
A simple solution for frequency sharing
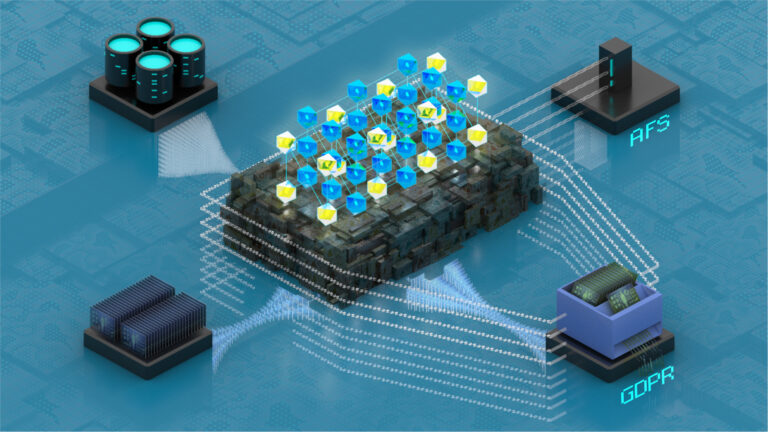
Bioengineering
Safeguarding the right to be forgotten
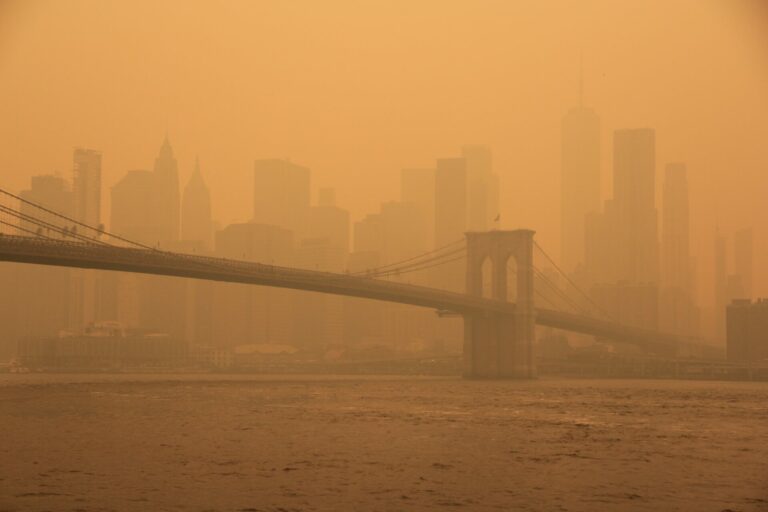
Applied Mathematics and Computational Sciences
Neural networks give deeper insights
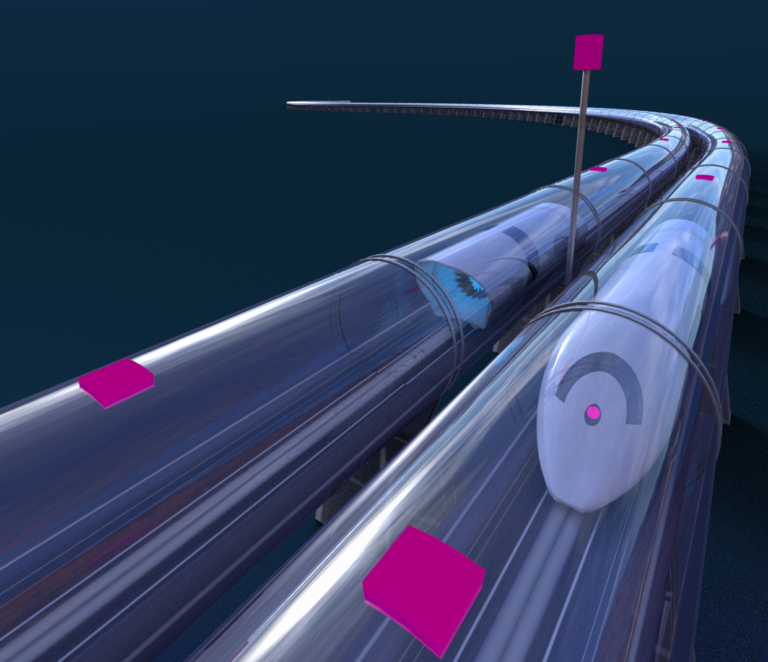
Applied Mathematics and Computational Sciences