Computer Science
Finding the face in the crowd
An algorithm that maximizes the difference between data categories achieves high accuracy in classifying faces.

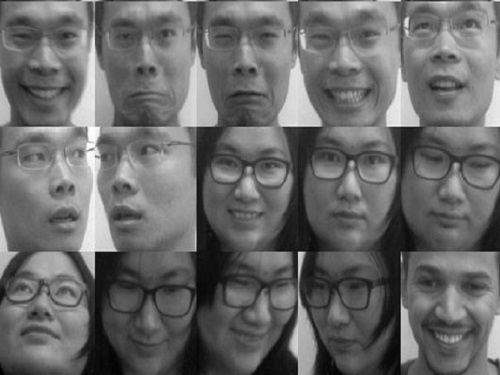
The algorithm, trained to simplify a matrix of faces, can assign a new image to the right person.
© 2015 KAUST
Imagine being asked to pick out a particular face among a sea of people. Researchers from KAUST have come up with a method to accurately sift complex biological data.
Xin Gao and his team have developed an algorithm that achieves high accuracy in difficult classification problems1.
Biological data are often presented with dizzying complexity. They can be made up of many samples, with thousands of features per sample, and need to be converted into a simpler form for analysis.
Popular statistical methods for complexity reduction, such as principal component analysis, assign both positive and negative values to the simplified data. Thus, explains Gao, they cannot fit to the non-negative nature of some practically useful data, such as image and gene expression data.
Instead Gao, with postdoctoral fellow Jingyan Wang from KAUST’s Computer, Electrical and Mathematical Science and Engineering Division, improved upon a method that does not assign negative numbers, the so-called non negative matrix factorization (NMF). A complex dataset is expressed as a matrix — each row is a feature and each column a sample — and is then broken down into simpler matrices with fewer features for representation of the data. NMF is first ‘trained’ on known data and then used to represent test data.
Gao and Wang utilized the fact that each sample in a training set can be assigned to a particular class. They then increased the distance between any two pairs belonging to different classes to develop Max-Min NMF. “Instead of dealing with all the inter-class pairs equally, we pick the closest inter-class pair and maximize the distance, so that all other inter-class pairs will also be separated simultaneously,” says Gao.
They applied Max-Min NMF to face classification using images of 11 people bearing different facial expressions. Each image was treated as a sample with 1024 features. First they trained Max-Min NMF to derive a low dimensional matrix that represented the faces, they then showed that they could assign any grey scale image to the correct person. “A practical example”, says Gao, “is the face recognition system of U.S. Customs and Border Protection.”
In future work, through a collaboration with researchers from the Université Claude Bernard Lyon in France, Gao wants to take face recognition to an even more challenging level by extending it to distinguish images of twins’ faces. Not only will they be able to pick a stranger from a crowd, they will be able to tell him from his twin brother.
References
- Wang, J.-Y. & Gao, X. Max-min distance nonnegative matrix factorization. Neural Networks 61, 75-84 (2015).| article
You might also like
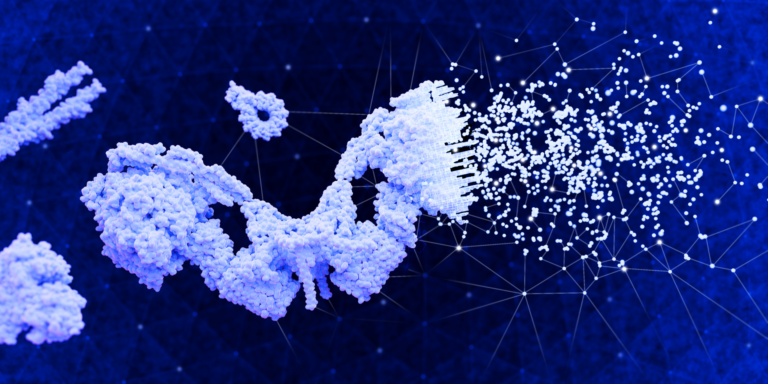
Bioengineering
AI tool predicts function of unknown proteins
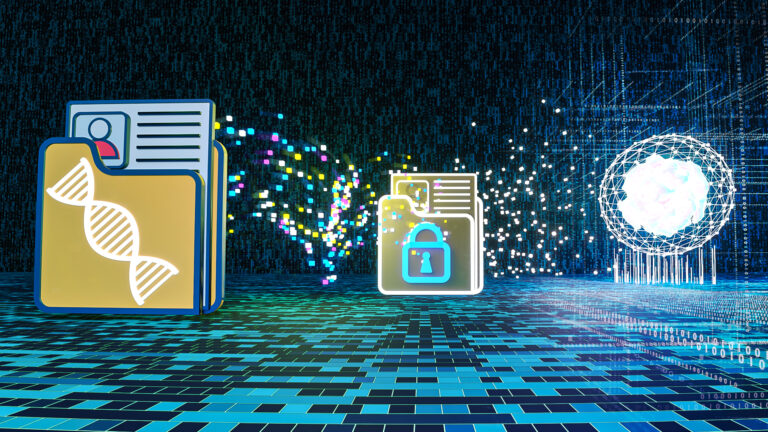
Bioengineering
Shuffling the deck for privacy
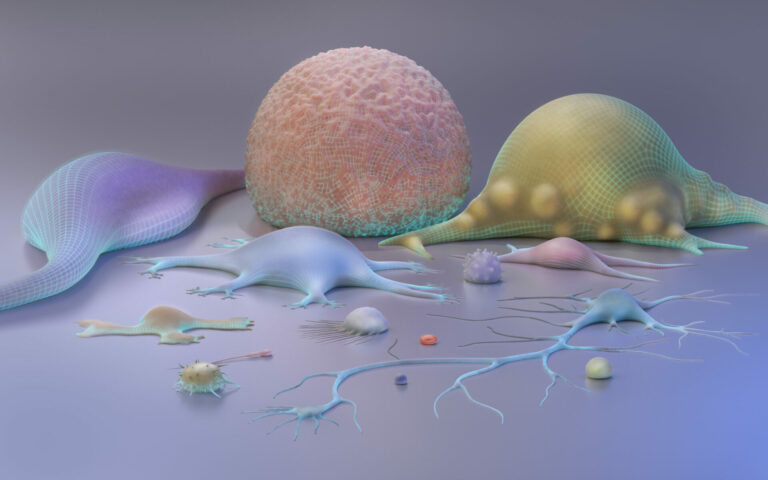
Bioengineering
AI for cells helps illuminate their identity
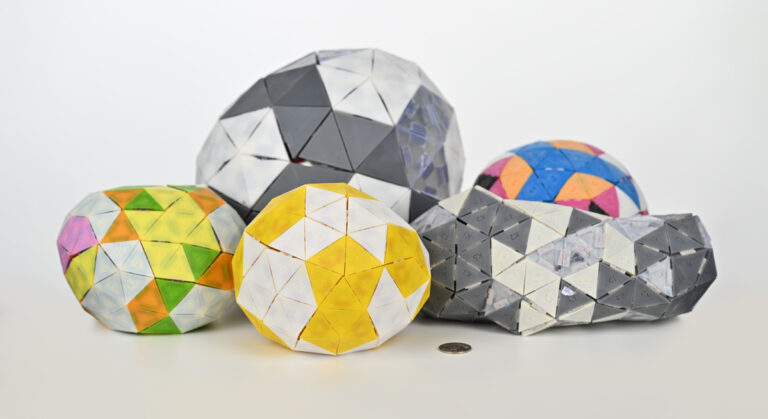
Computer Science
Dr. KID turns biological models into fun puzzles
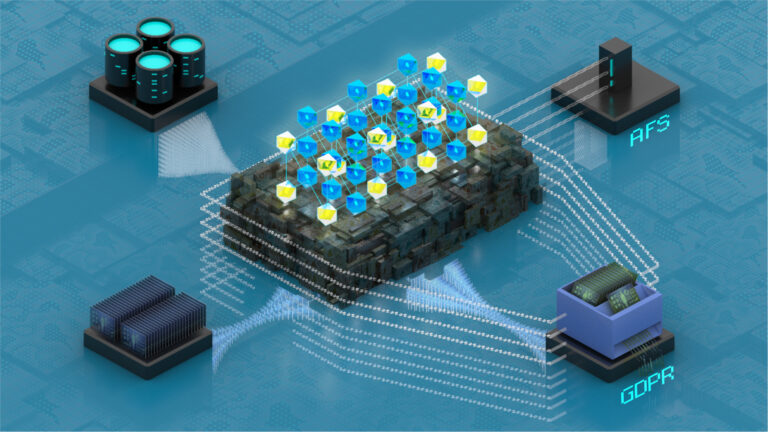
Bioengineering
Safeguarding the right to be forgotten
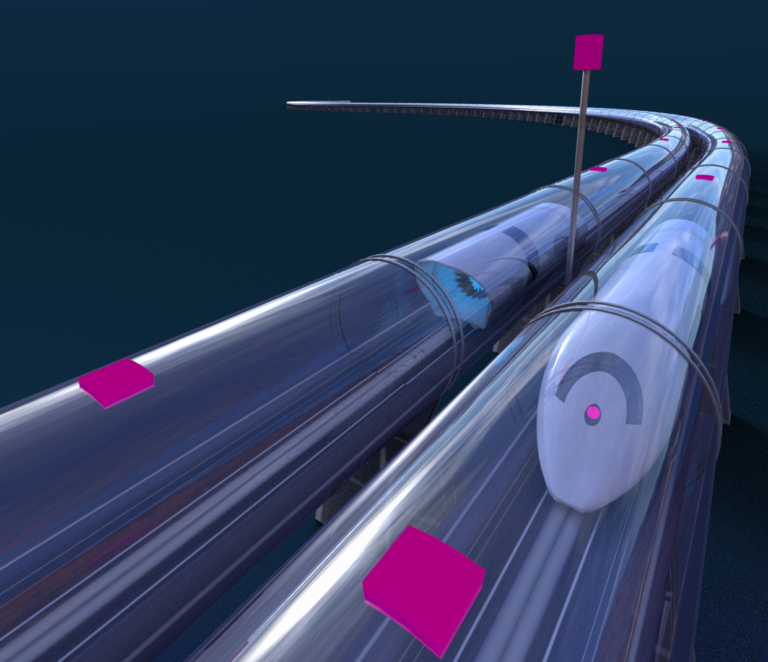
Applied Mathematics and Computational Sciences
Staying online in the high-speed tube of the future
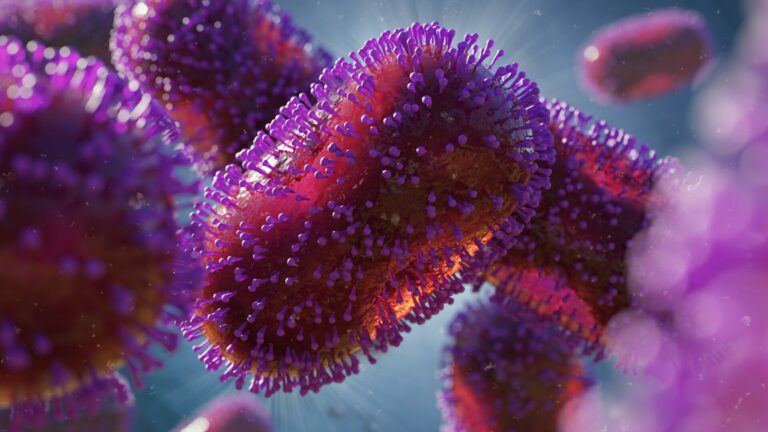
Bioengineering
Lab-grown skin helps unlock secrets of mpox virus infection
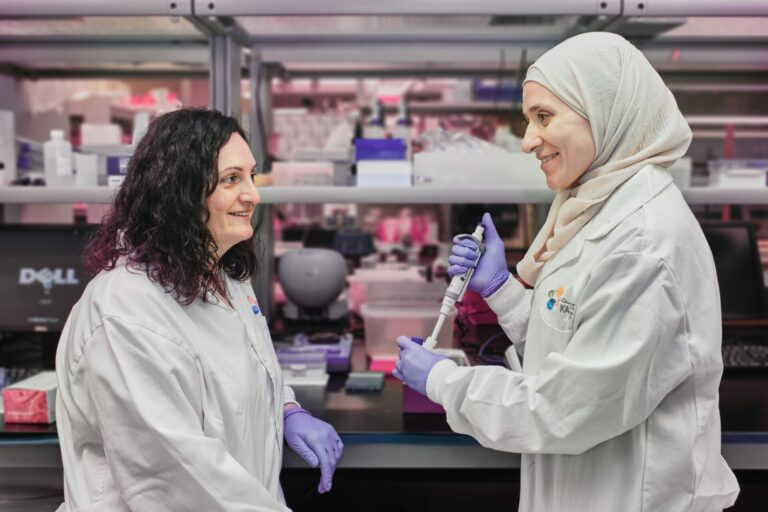
Bioengineering