Statistics
Modeling where the wind blows
A new statistical technique could be used to more accurately locate global wind resources.
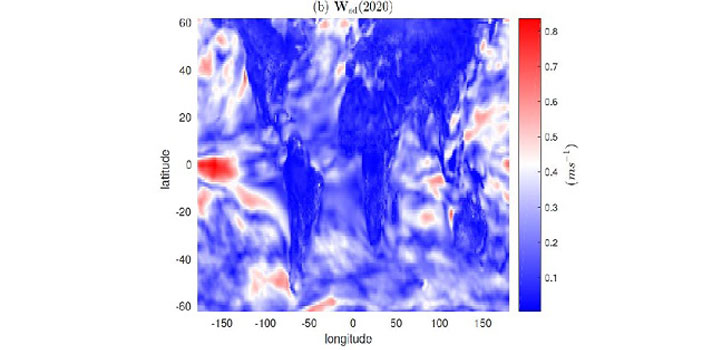
By incorporating geographical information into models for wind energy, researchers from KAUST have developed an innovative statistical tool that reduces the computational burden of locating global wind resources.
Renewable energy generated from wind has the potential to ease the demand for resources, as well as reduce greenhouse gas emissions. However, locating global wind resources is very challenging because of the difficulty in storing the large amounts of data generated by climate model simulations.
This led postodoc Jaehong Jeong and his supervisor Marc Genton from KAUST, working with colleagues from the University of Notre Dame in the United States, to develop a statistical model that includes geographical and physical information for mountains, such as longitude and altitude, and can better account for their impacts on wind speed.
“Our model incorporates more large-scale geographical information to explain the behavior of wind across longitudes,” explains Jaehong. “In particular, the model incorporates information on the physical characteristics of mountains, which have been shown to affect the spatial smoothness of wind fields, and leads to better model selection metrics for modeling wind data, reducing the data storage and computational requirements of large datasets.”
Wind fields have been shown to exhibit varying spatio-temporal smoothness across longitudes due to land, ocean and mountainous features, and differences in altitude can produce thermal effects as well as the acceleration of wind flows over hills and funneling effects in narrow valleys.
The researchers therefore developed a flexible model in which ocean, land and high mountains with altitude information were included as covariates to account for the statistical behavior of wind speed. And by using a stochastic generator, they were able to incorporate large amounts of wind data, allowing them to better model the spatio-temporal variability of wind resources.
Once the mean and covariance parameters were estimated from a sample set, the stochastic generator was able to generate surrogate runs whose large-scale features were almost identical to the original runs, and a more detailed assessment of wind uncertainty under different initial conditions was achieved, without the need for storing a prohibitive amount of information.
“Our work could provide wind energy assessments in developing countries, where no regional studies are available, and could be a stepping stone for more complex and realistic problems regarding wind resources, such as daily or monthly global wind ensemble and multivariate wind-field modeling,” says Genton.
References
-
Jeong, J., Castruccio, S., Crippa, P. & Genton, M.G. Reducing storage of global wind ensembles with stochastic generators. Annals of Applied Statistics arXiv:1702.01995 (2017).| article
You might also like
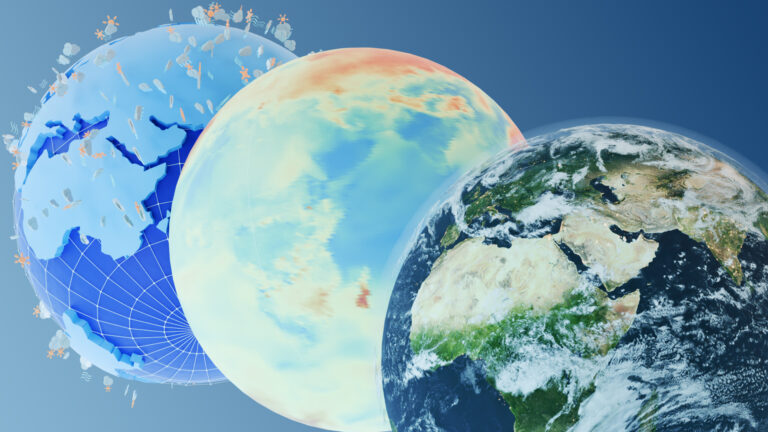
Statistics
A high-resolution boost for global climate modeling
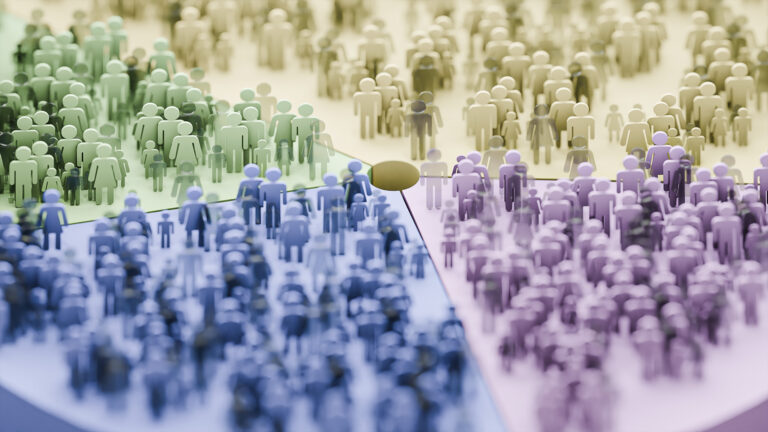
Applied Mathematics and Computational Sciences
Finer forecasting to improve public health planning
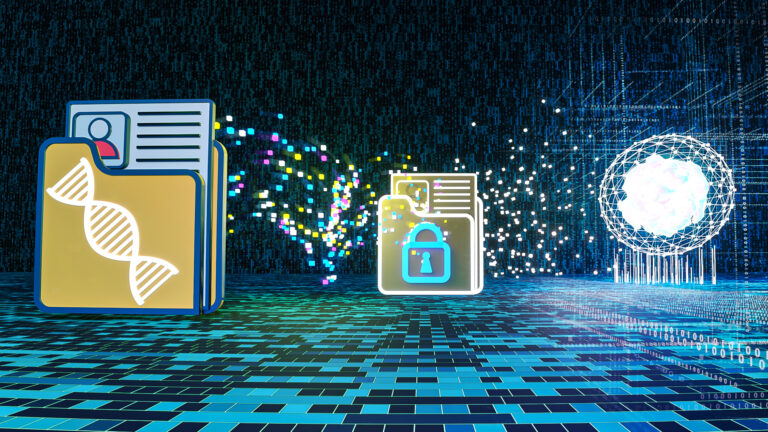
Bioengineering
Shuffling the deck for privacy
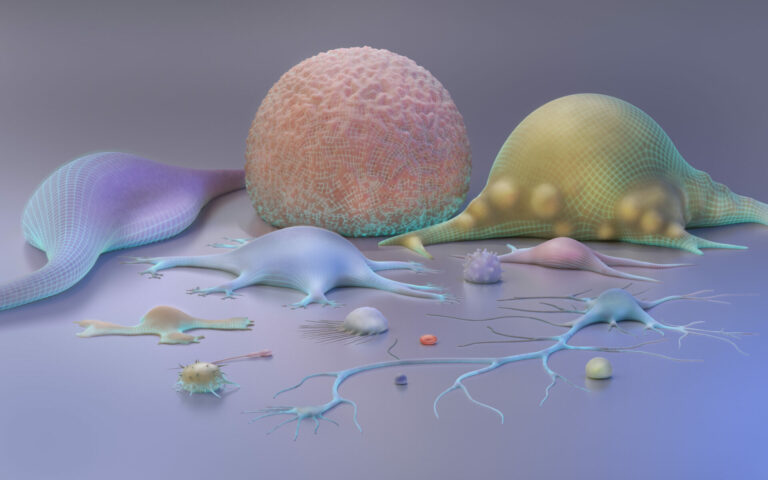
Bioengineering
AI for cells helps illuminate their identity
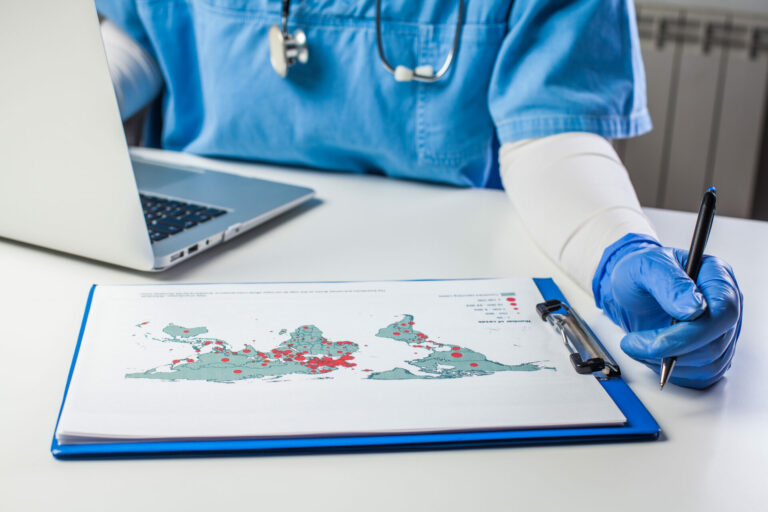
Applied Mathematics and Computational Sciences
Global look at sex differences in young people's mortality
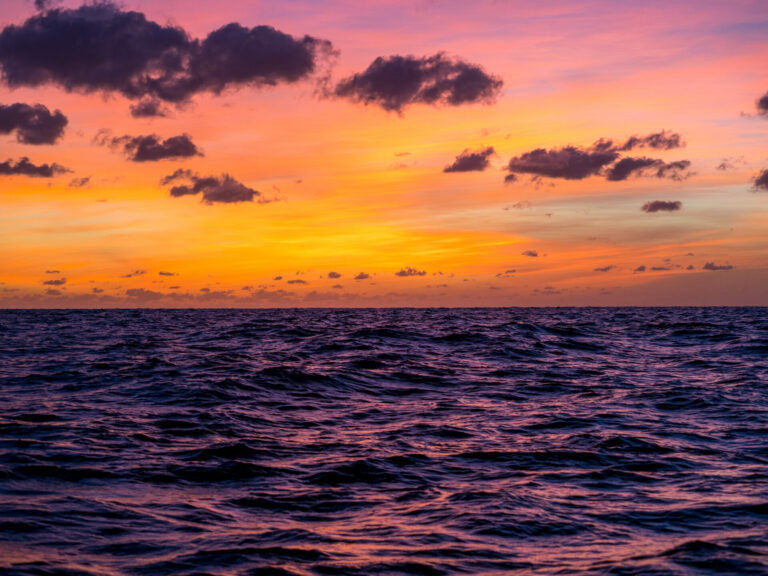
Applied Mathematics and Computational Sciences
Going likelihood-free with neural networks
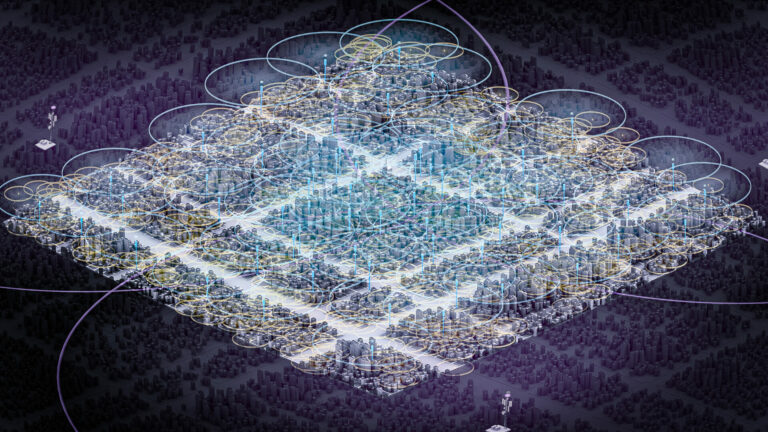
Applied Mathematics and Computational Sciences
A simple solution for frequency sharing
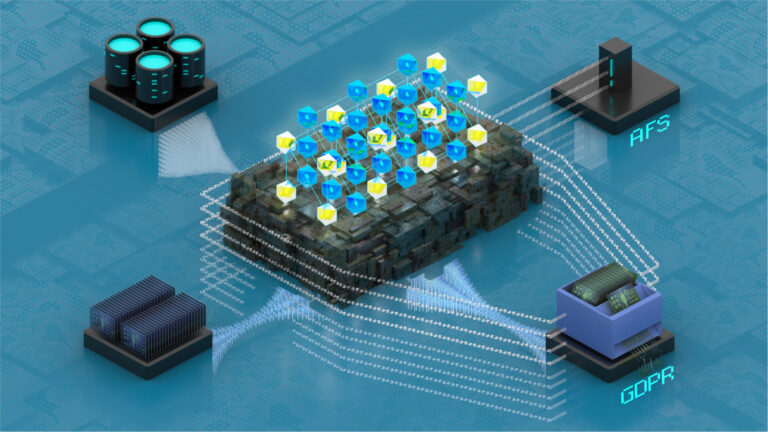
Bioengineering