Bioscience
The awesome scope of big data
Sifting through huge amounts of data may bring better understanding of whale shark social structures, protein targets for drug therapies and disease-causing genes.
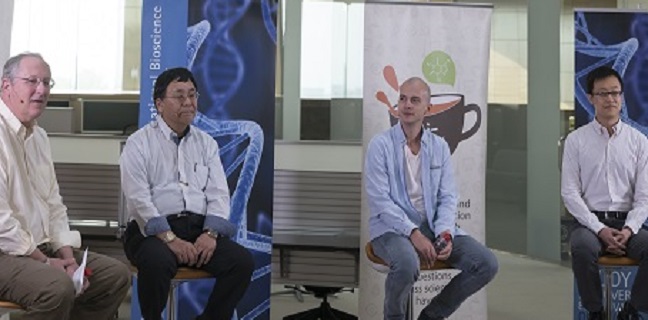
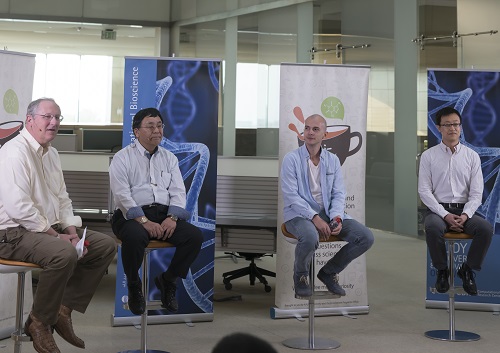
KAUST researchers discuss how they look for clues to major health questions by sifting through vast datasets. (L-R) Jim Calvin, Takashi Gojobori, Robert Hoehndorf and Xin Gao.
© 2017 KAUST
Anyone looking for answers on personalized medicine, human health, food production, the environment or ecology will turn to big data, says James Calvin, vice president of academic affairs at KAUST; “Big data is a concept that permeates all of the biological sciences.”
Researchers at the University’s Computational Bioscience Research Center work at the intersection of computer science and biology to sift through huge amounts of biomedical and biotechnological data. Their work, and that of international colleagues, could give clues to some major questions in the life sciences.
“In the last 10-15 years, technological breakthroughs have allowed us to produce more and more data,” says computer scientist Robert Hoehndorf. To put things in perspective, he says, tens of thousands of papers have been published on diabetes, producing large amounts of research data that are uploaded to databases; however, “How can we connect all these different research results to provide a big picture?” he asks. Integrating this data could allow a better understanding of disease, guiding researchers toward potential treatments.
Hoehndorf’s main area of interest is the field of symbolic artificial intelligence (AI), which explores how to make machines that are similarly intelligent to humans. AI systems are being used to study health problems and to do biomedical research, he explains. In the area of big data, these systems are being used to integrate huge amounts of data and identify consistencies and contradictions within them.
Hoehndorf and colleagues developed a computational method that allows the integration of data on tens of thousands of observable disease characteristics in yeast, fish, worms, flies, mice and humans. The method, called PhenomeNet, computes similarity between two sets of phenotypes—observable characteristics that result from the interactions of genes with the environment. This can help suggest genes that might underpin disease.
Focusing on a different target, computational scientist Xin Gao is interested in developing computational models and machine-learning techniques to analyze protein structures, determine what they look like three-dimensionally, how they function, and how their behaviors can be controlled in complex biological networks.
“I do not know the answer to whether big data can directly solve our health problems, but I do know with certainty that if these problems can be solved, then big data will be a part of the solutions,” says Gao.
Mining huge amounts of data from pharmaceutical studies can help drug development, for example, says Gao. “Drug development is an extremely expensive and time-consuming process. It routinely takes pharmaceutical companies tens of years and billions of dollars to develop one single drug. The failure rate for drug development is extremely high,” he explains. Huge amounts of computational and experimental data are generated as a result of this process. “Instead of throwing this data away after a drug has been developed, it makes a lot of sense to develop computational methods to effectively mine some knowledge and important information and hopefully reuse it in the development of future drugs.”
Gao and colleagues developed a combined approach1 involving nuclear magnetic resonance spectroscopy, single-molecule fluorescence resonance energy transfer, and molecular dynamics simulation to investigate the dynamic interactions between proteins that lack fixed 3D structures. “In reality, proteins are not rigid bodies. They have certain dynamics and kinetics in our body. In order to better find the correct matching between drugs and [targeted] proteins, you have to take such conformational changes into consideration,” he says.
Thanks to the Shaheen II supercomputer at KAUST, rated as the 15th fastest computer in the world, Gao’s team can conduct many molecular dynamics simulations on target proteins as they endeavor to make more reliable predictions for drug development.
Another KAUST researcher, bioscientist Takashi Gojobori, aims to elucidate the evolutionary origin of the neural network and its application to synthetic biology for developing bioenergy. Gojobori has also used big data with colleagues Gao, Hoehndorf and other international scientists. Together they developed a computational screening method2 that evaluates the potential of bacterial strains to produce free fatty acids that can act as precursors for energy-dense biofuels.
More recently, Gojobori has been sequencing the genomes of Red Sea whale sharks. In collaboration with KAUST marine scientist Michael Berumen, Gojobori is sifting through huge amounts of genomic data that will shed light on the social structures of whale sharks travelling in groups. The two researchers are also interested in understanding how these fish maintain high levels of energy when they must dive as deep as 200m to feed.
Gojobori has also co-authored studies with Emperor Akihito of Japan, who is a published researcher in fish science. Together, they have been analyzing nuclear and mitochondrial genes belonging to gobioid fish in order to understand how new species of these fish evolved as a result of geographical differentiation.
References
- Wu, S., Wang, D., Liu, J., Feng, Y., Weng, J., Li, Y., Gao, X., Liu, J. & Wang, W. The dynamic multisite interactions between two intrinsically disordered proteins. Angewandte Chemie 129, 7623-7627 (2017).| article
- Motwalli, O., Essack, M., Jankovic, B.R., Ji, B., Liu, X., Ansari, H.R., Hoehndorf, R., Gao, X., Arold, S. T., Mineta, K., Archer, J.A., Gojobori, T., Mijakovic, I. & Bajic, V.B. In silico screening for candidate chassis strains of free fatty acid-producing cyanobacteria. BMC Genomics 18, 33 (2017).| article
- This article was based on a KAUST Sci-Café: Can Big Data Solve My Health Problems?
You might also like
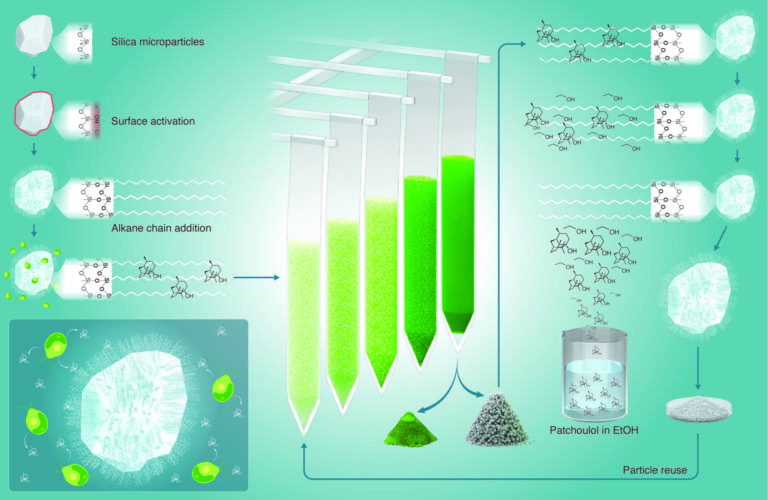
Bioengineering
High value harvests from designer algae
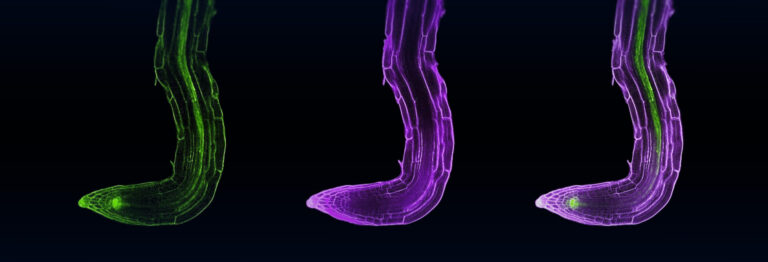
Bioscience
Digging into the world of plant-growth-promoting microbes
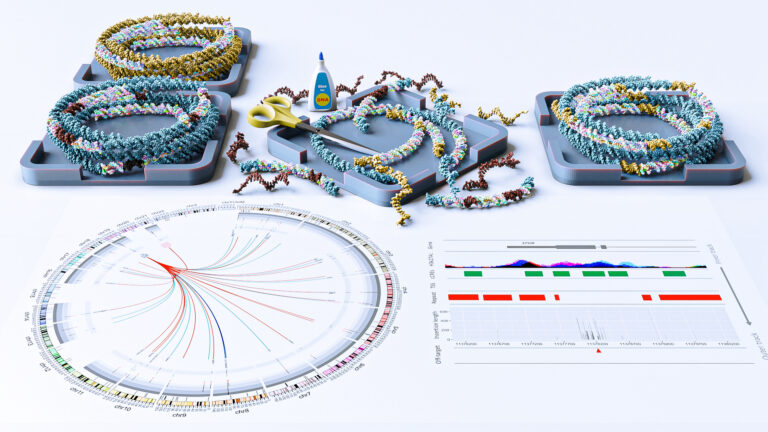
Bioengineering
Stray DNA fragments challenge CRISPR precision
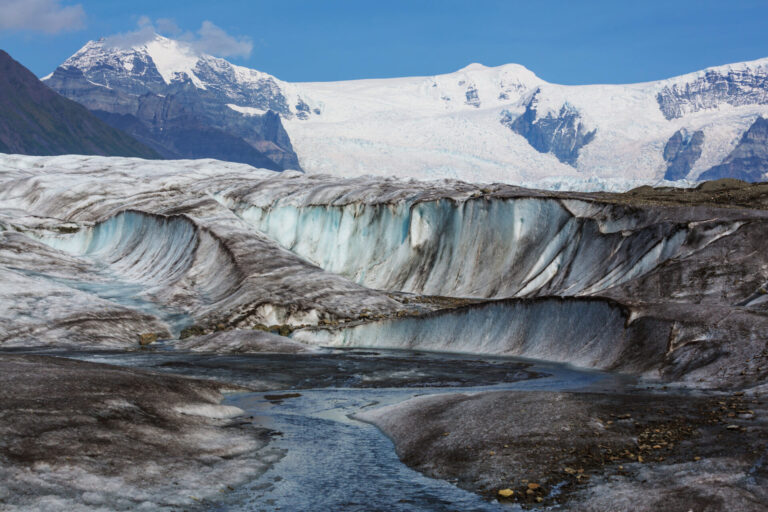
Bioscience
Unique microbiome discovered in mountain streams
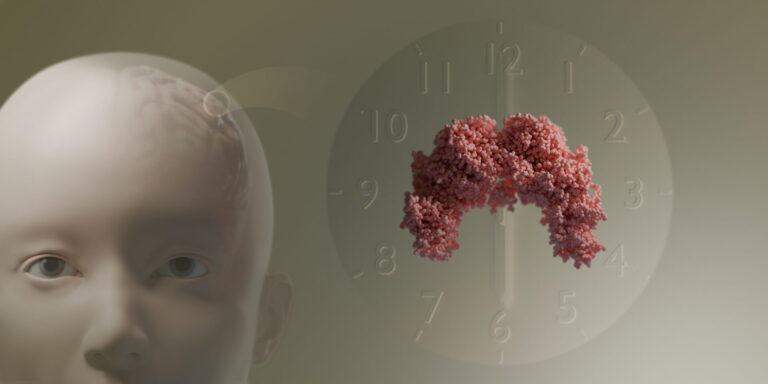
Bioscience
How a multitasking protein keeps the body’s clock in sync
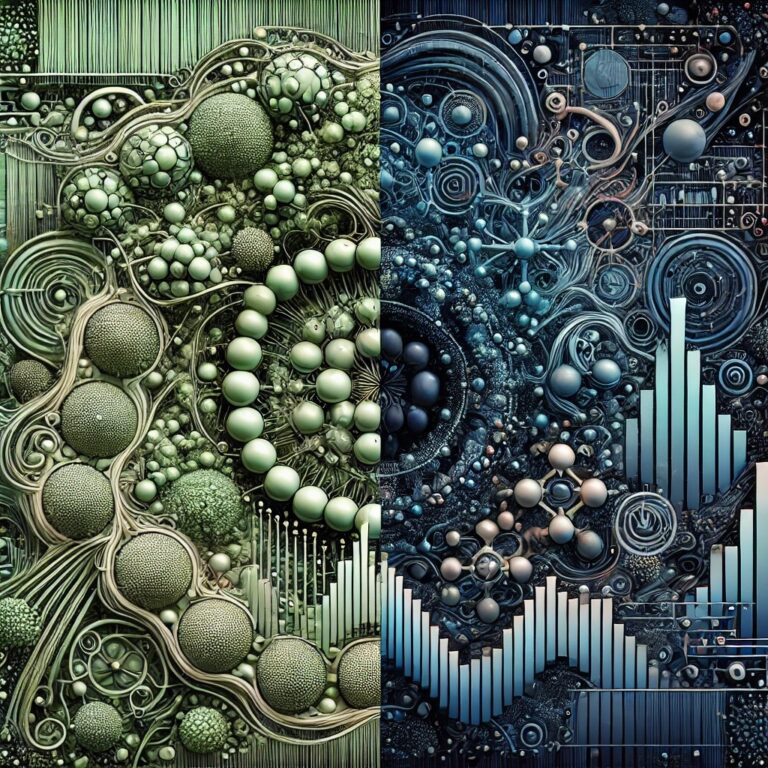
Bioscience
The theory of everything that wasn’t
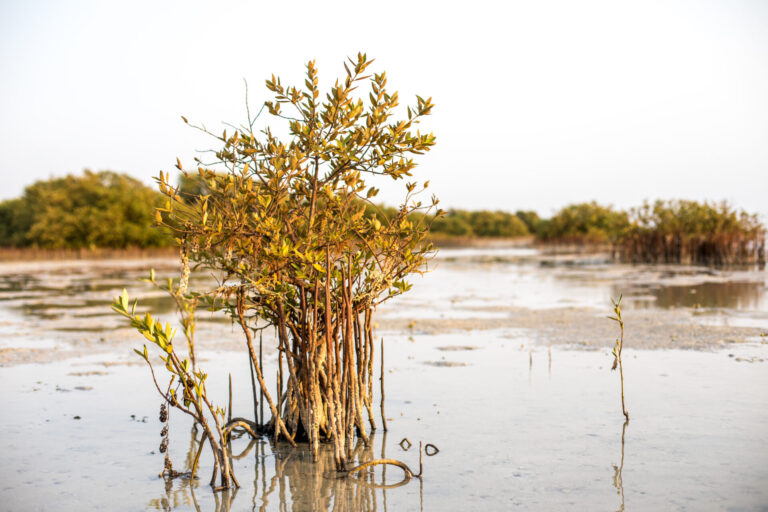
Bioscience
Mangrove microbes to munch on plastic
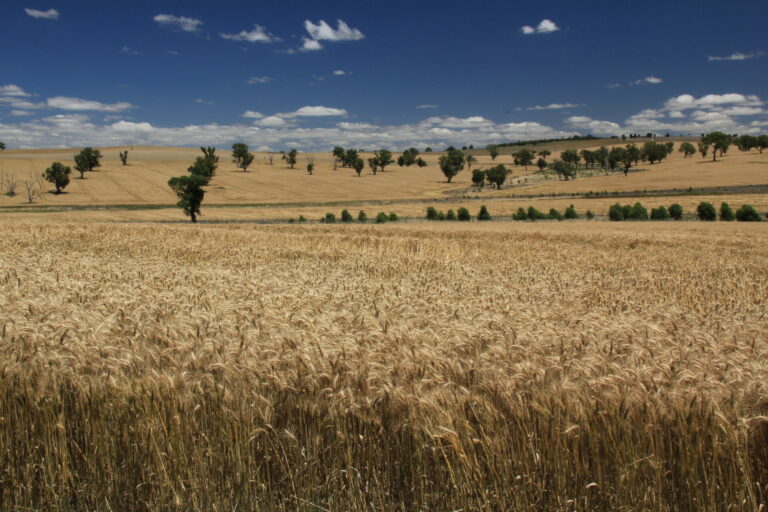
Bioscience