Computer Science
Sharing movement aids learning
Automated learning of an individual’s movement patterns shared over mobile and social networks could help us to connect better.
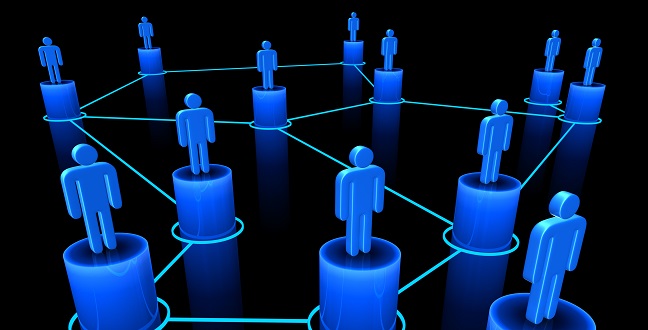
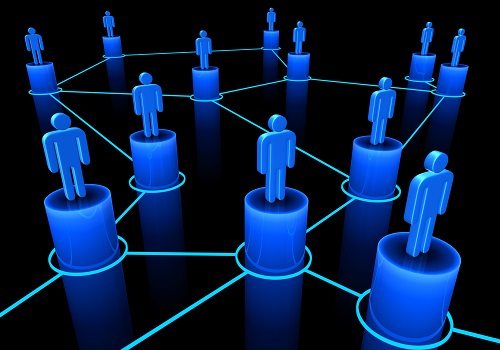
KAUST has developed a computer scheme that can learn mobility patterns and enhance the prediction of social ties and habitual activities.
© 2016 Alamy CX92KK
Information about our locations is recorded almost everywhere we go, either intentionally over social media networks like Facebook, or incidentally via our mobile phones and their cellular and Wi-Fi networks. Mining this location data is valuable for marketing and social networking, but to make effective use of the data for research, it is necessary to characterize the habitual movement patterns of individuals—something that computers have found difficult to do on their own.
KAUST researchers Xiangliang Zhang, Basma Alharbi and Abdulhakim Qahtan have now developed a computer scheme that can learn such mobility patterns and enhance the prediction of social ties and habitual activities without human intervention1.
“Our latent mobility patterns reveal our way of life, work and habits,” explained Zhang. “This makes the raw data of an individual’s movement or trajectory a rich source of information that could be used to recommend social links based on shared common interests, habits or preferences.”
There are many challenges to the automated learning of mobility patters from raw trajectory data. These include aspects such as missing data and locations, differences in the amount of data among individuals while trying to treat individuals equally and the lack of information on how socially important a particular location may be even if it is visited infrequently.
“We used a probabilistic approach to uncover the hidden patterns manifested in the observed raw movement data,” said Zhang. “Simply put, our model first describes how we think human movement data is generated, where the subject’s movements are governed by a small set of unobservable purposes that we infer and reverse engineer to reproduce the observed data.”
For example, when a subject visits a movie theater, the event is mapped to the predefined purpose of “entertainment.” An individual’s movement habits can then be learned from the subject’s purposes. The team’s model also incorporates elements that allow it to handle situations of incomplete or disparate data. It does this by considering the time a location was visited, learning from groups of individuals to strengthen sparse data and introducing a mathematical transformation to handle variance in the popularity of different places.
“Our work will be important in profiling and personalizing information services that could be used by recommendation systems and targeted advertising,” said Zhang. “However, the latent patterns manifested in such data are also important for understanding human behavior in general and could potentially be used in other areas, such as in fraud detection based on credit card usage patterns.”
References
- Alharbi, B., Qahtan, A., Zhang, X. Minimizing user involvement for learning human mobility patterns from location traces. Association for the Advancement of Artificial Intelligence, 2016. | article
You might also like
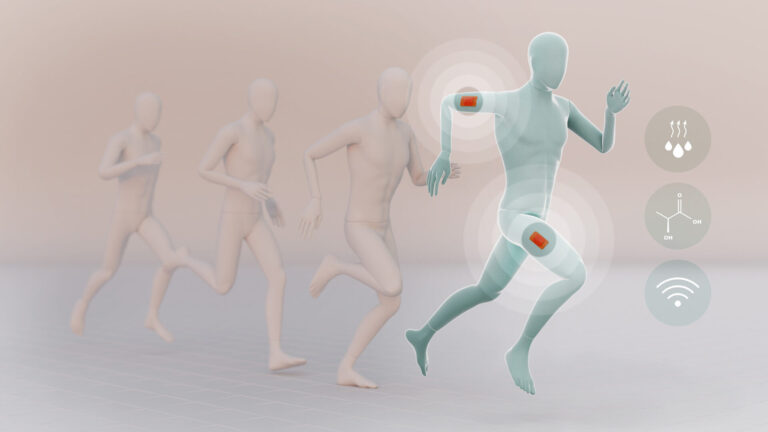
Computer Science
Sweat-sniffing sensor could make workouts smarter
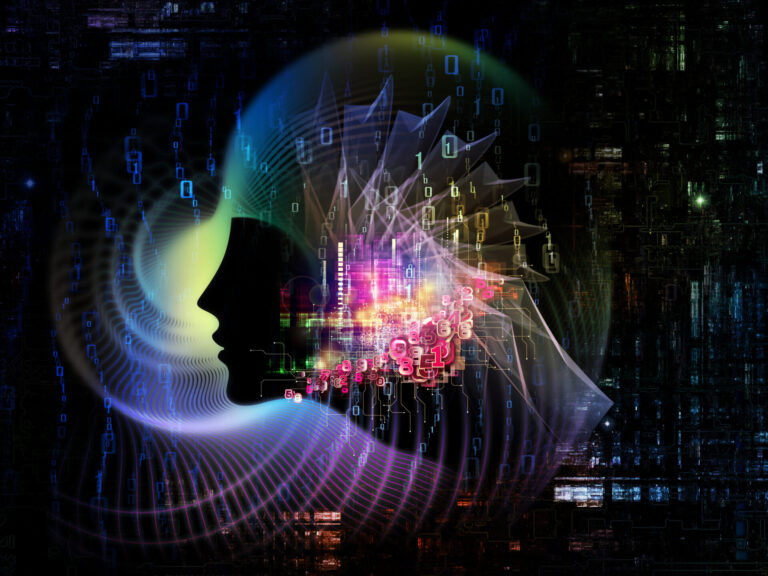
Computer Science
A blindfold approach improves machine learning privacy
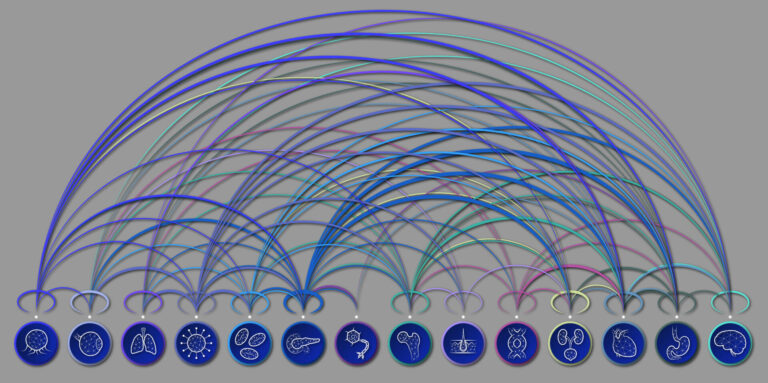
Computer Science
AI tool maps hidden links between diseases
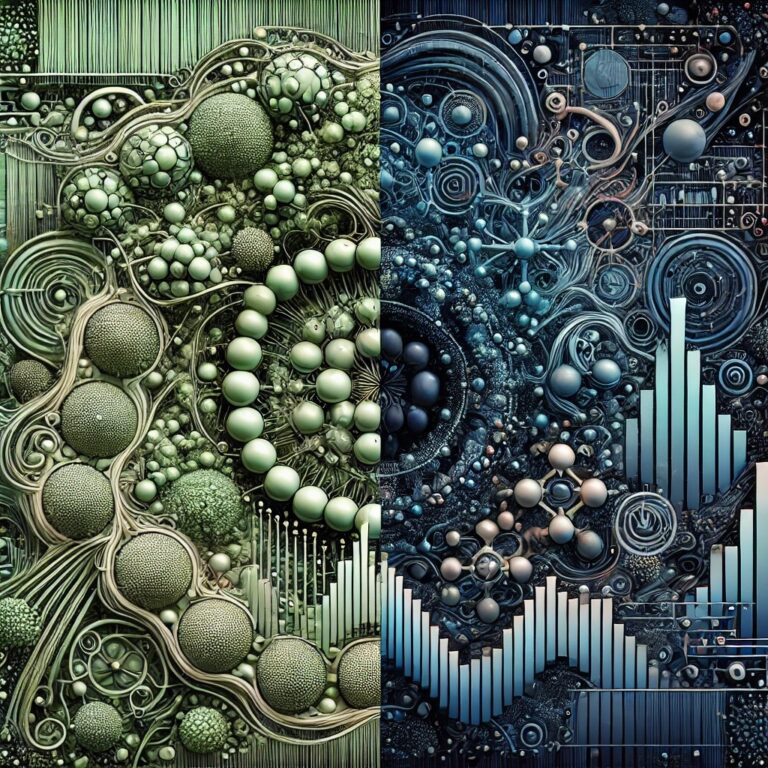
Bioscience
The theory of everything that wasn’t
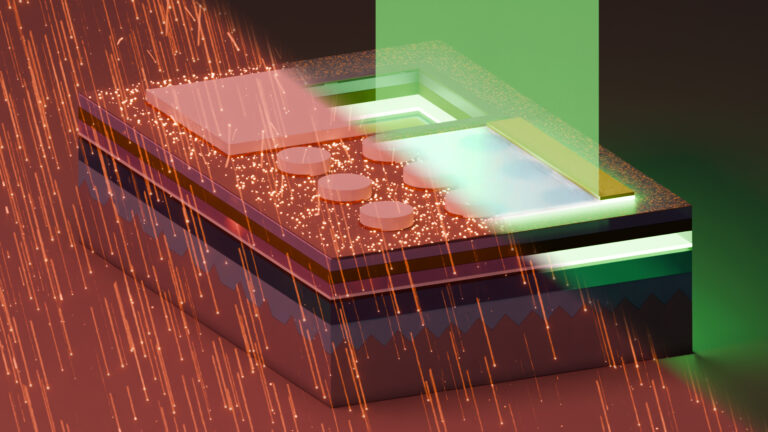
Computer Science
A new path to high-efficiency micro-LEDs
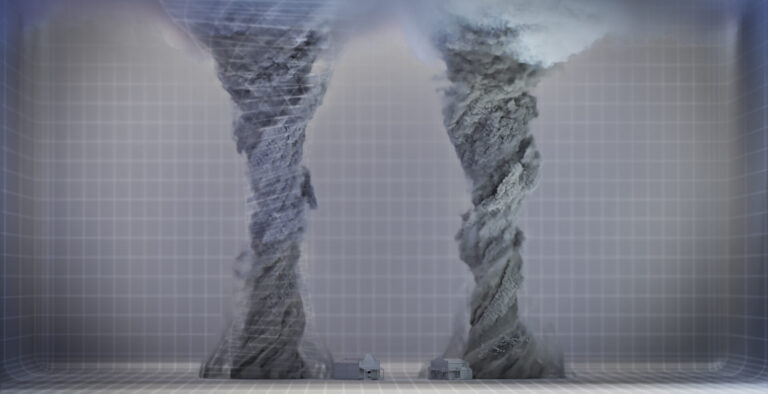
Computer Science
Cyclones meet their mathematical match
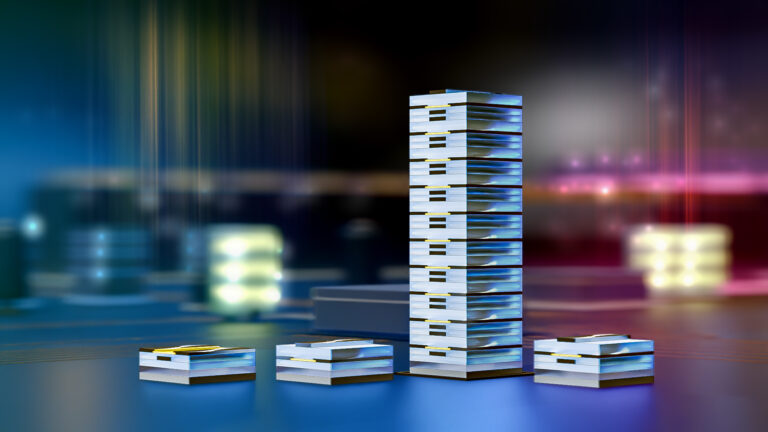
Applied Physics
Onward and upward to smaller faster devices
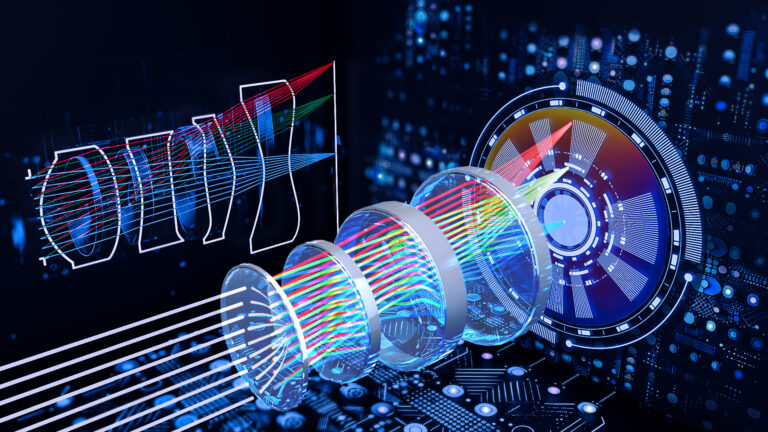
Computer Science