Statistics
Beating the curse of dimensionality
A partial matching approach can overcome the dimensionality "curse" of continuous measurements over time to yield more accurate future predictions.
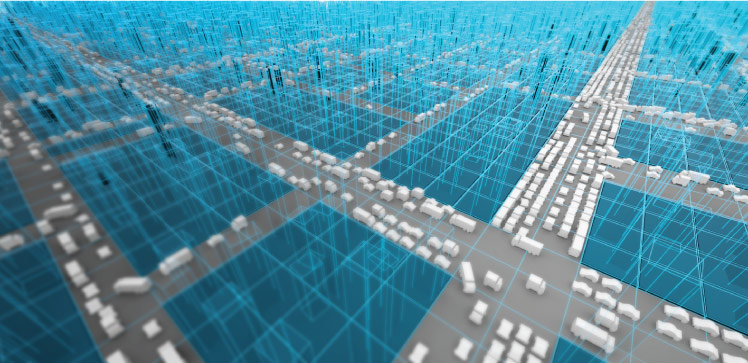
By scanning past data for both partial and complete matches to current observations, a KAUST-led research team has developed a prediction scheme that can more reliably forecast the future trajectory of environmental parameters.
The collection of data at regular intervals over time is common in many fields but particularly so in environmental, transportation and biological research. Such data are used to monitor and record the current state and also to help predict what might come in the future. A typical approach is to look for previous patterns or trajectories in the data that match the current trajectory.
However, in practice, there are never any complete matches, and so the predictor needs to find smaller and smaller time windows in past data that provide a partial match. This results in a loss of context and any broader trends that might have given a better prediction, while possibly drawing in random noise.
“Predicting future time-series trajectories is challenging in that the trajectories are composed of many sequential observations or ‘dimensions,’ which limits multivariate prediction approaches,” says Hernando Ombao from KAUST. “This is known as the curse of dimensionality.”
To overcome these challenges, postdoc Shuhao Jiao developed a method called partial functional prediction (PFP) that integrates information from all past complete and partial trajectories. This optimized approach uses all the available data, capturing both long-term trends and well-matched partial trajectories.
“By smoothing the trajectories, we can transform the curse into a blessing by capturing the big picture of the dynamic information of trajectories,” Jiao says. “Our method incorporates both crosstrajectory and intratrajectory dependence, which previous methods have not achieved.”
The approach involves a step-wise procedure where the data are first analyzed for longer complete trajectories, the “residual” partial components are then extracted as fragments independent of past trends and anything left over is assigned to random noise. The three functions are then applied to the prediction window.
The team, together with collaborator Alexander Aue from the University of California, demonstrated their method on the prediction of fine particulate matter in the air and traffic flow and showed that their PFP method gave far more accurate predictions than existing methods, particularly for longer term forecasts.
“Our method shows that by incorporating dependence information within and across trajectories, it is possible to achieve a pronounced improvement in the prediction of future trajectories,” Ombao says.
References
-
Jiao, S., Aue, A. & Ombao, H. Functional time series prediction under partial observation of the future curve. Journal of the American Statistical Association, advance online publication 6 July 2021.| article
You might also like
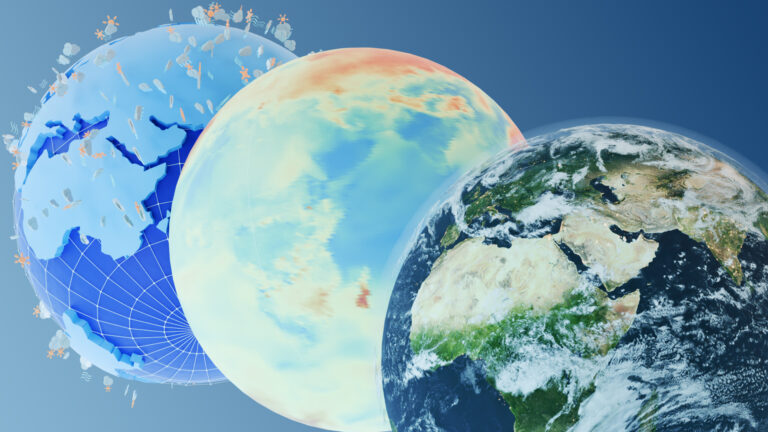
Statistics
A high-resolution boost for global climate modeling
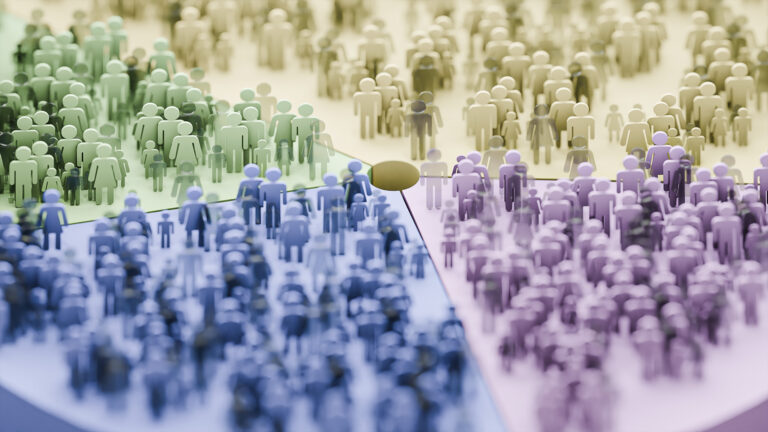
Applied Mathematics and Computational Sciences
Finer forecasting to improve public health planning
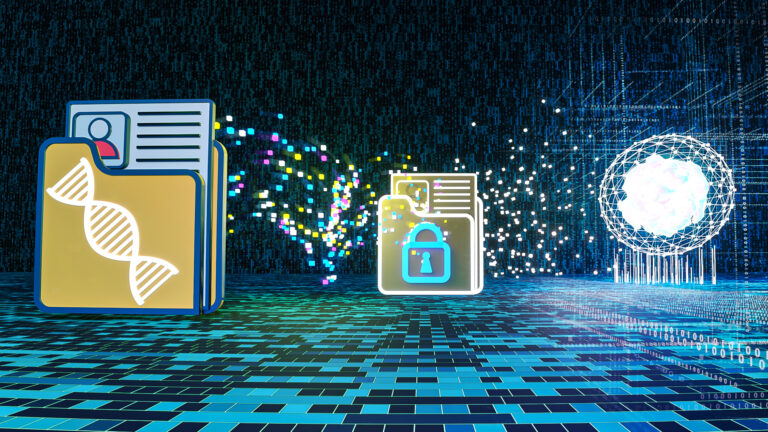
Bioengineering
Shuffling the deck for privacy
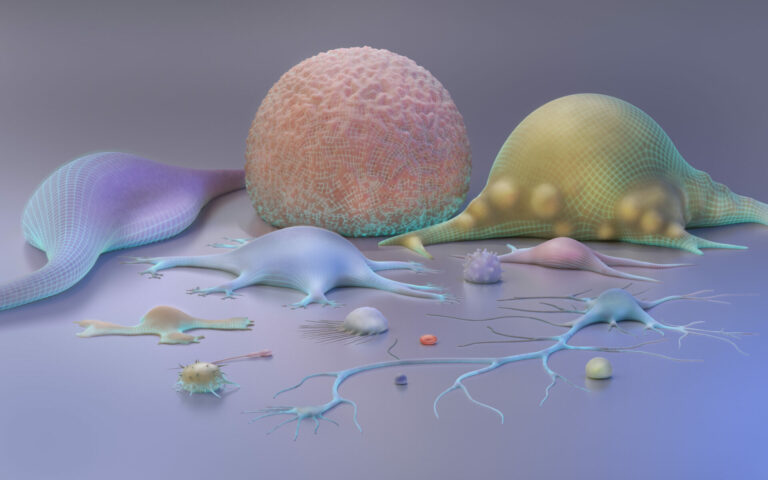
Bioengineering
AI for cells helps illuminate their identity
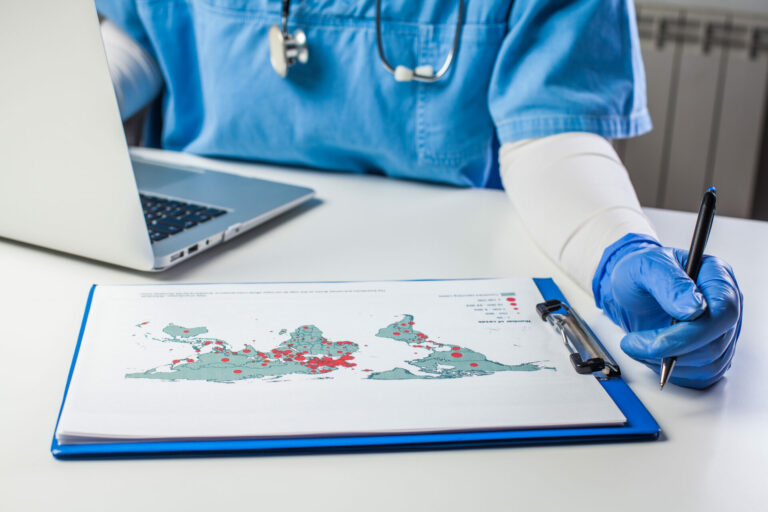
Applied Mathematics and Computational Sciences
Global look at sex differences in young people's mortality
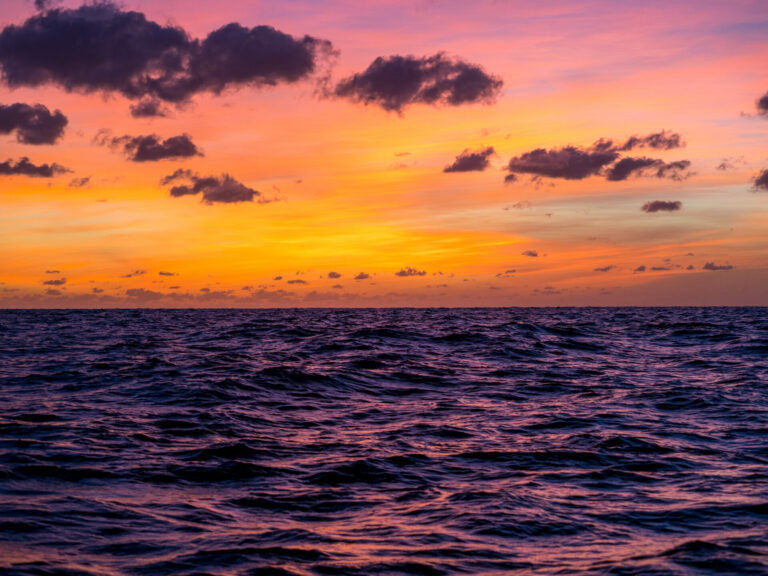
Applied Mathematics and Computational Sciences
Going likelihood-free with neural networks
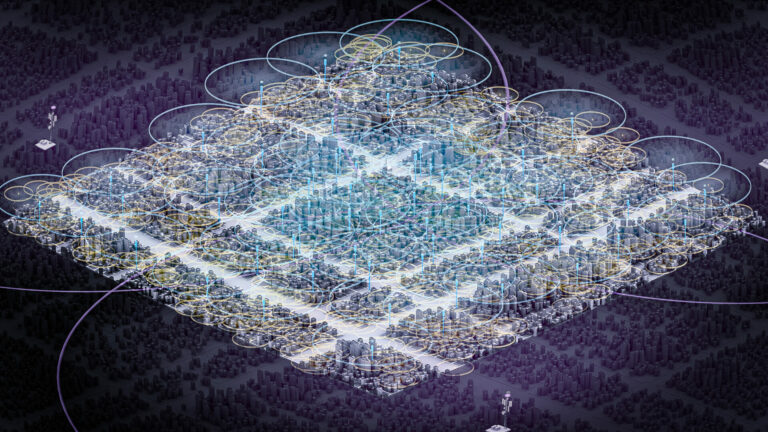
Applied Mathematics and Computational Sciences
A simple solution for frequency sharing
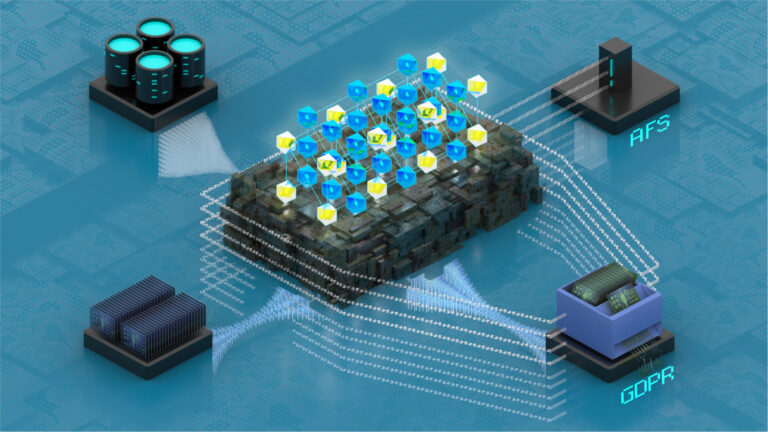
Bioengineering