Statistics
Trio of tuning tools for modeling large spatial datasets
Statistical tools help fine-tune the parameters and approximations of models used to make sense of large spatial datasets.
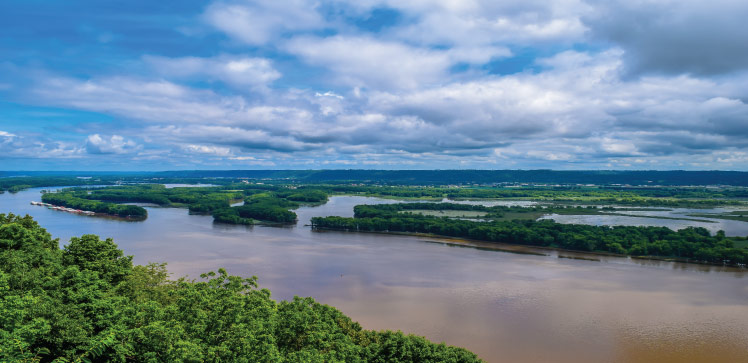
Predictive modeling of very large datasets, such as environmental measurements, across a wide area can be a highly computationally intensive exercise. These computational demands can be significantly reduced by applying various approximations, but at what cost to accuracy? KAUST researchers have now developed statistical tools that help remove the guesswork from this approximation process.
“In spatial statistics, it is extremely time consuming to fit a standard process model to large datasets using the most accurate likelihood-based methods,” says Yiping Hong, who led the research. “Approximation methods can cut down the computation time and computing resources significantly.”
Rather than model the relationship between each pair of observations explicitly using a standard process model, approximation methods try to adopt an alternative modeling structure to describe the relationships in the data. This approach is less accurate but more computationally friendly. The tile low-rank (TLR) estimation method developed by KAUST, for example, applies a block-wise approximation to reduce the computational time.
“Thus, one needs to determine some tuning parameters, such as how many blocks should be split and the precision of the block approximation,” says Hong. “For this, we developed three criteria to assess the loss of prediction efficiency, or the loss of information, when the model is approximated.”
With a lack of informative measures for evaluating the impact of approximation, Hong, along with computational scientist Sameh Abdulah and statisticians Marc Genton and Ying Sun, developed their own. The three measures — the mean loss of efficiency, the mean misspecification and a root mean square of the mean misspecification — together provide insight into the “fit” of the approximation parameters to the dataset, including prediction variability, and not just the point-by-point evaluation given by conventional prediction criterion.
“We can use our criteria to compare the prediction performance of the TLR method with different tuning parameters, which allows us to suggest the best parameters to use,” says Hong.
The team applied the method to a real dataset of high-resolution soil moisture measurements in the Mississippi Basin. By adjusting tuning parameters using the new measures, the TLR approximation provided estimates that are very close to the exact maximum likelihood estimates, with a significantly shorter computational time.
“Our criteria, which were developed to choose the tuning parameter for TLR, can also be used to tune other approximation methods,” says Hong. “We now plan to compare the performance of other approximation methods developed for large spatial datasets, which will provide valuable guidance for analysis of real data.”
References
-
Hong, Y., Abdulah, S., Genton, M. G. & Sun, Y. Efficiency assessment of approximated spatial predictions for large datasets. Spatial Statistics 43, 100517 (2021).| article
You might also like
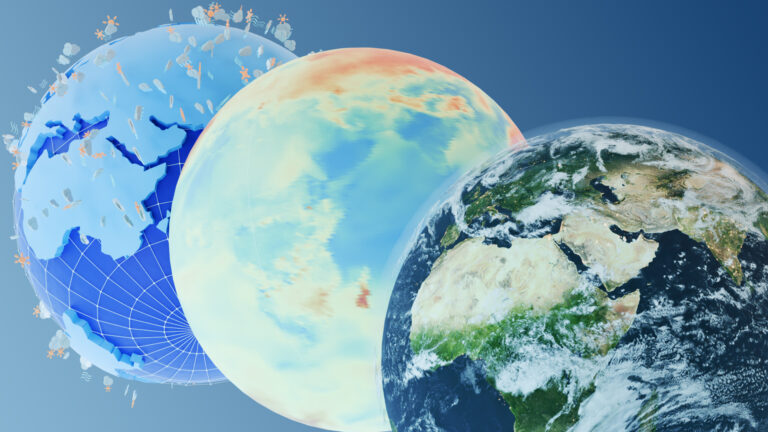
Statistics
A high-resolution boost for global climate modeling
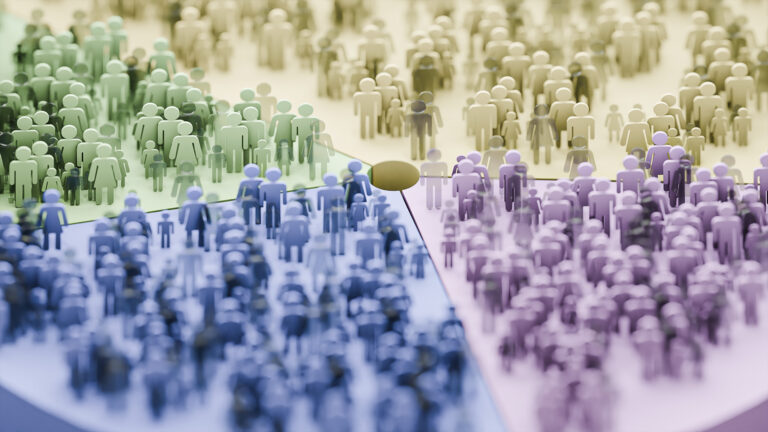
Applied Mathematics and Computational Sciences
Finer forecasting to improve public health planning
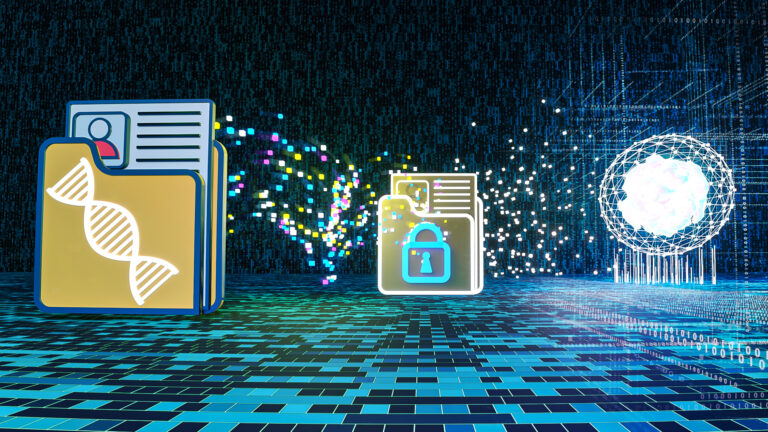
Bioengineering
Shuffling the deck for privacy
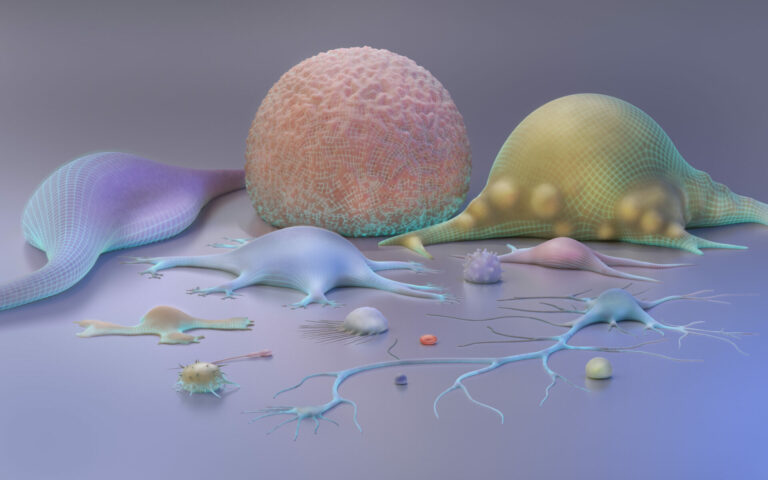
Bioengineering
AI for cells helps illuminate their identity
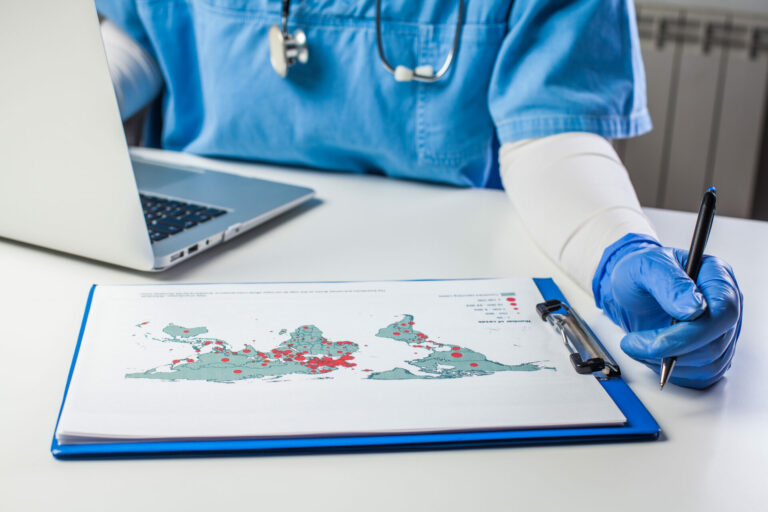
Applied Mathematics and Computational Sciences
Global look at sex differences in young people's mortality
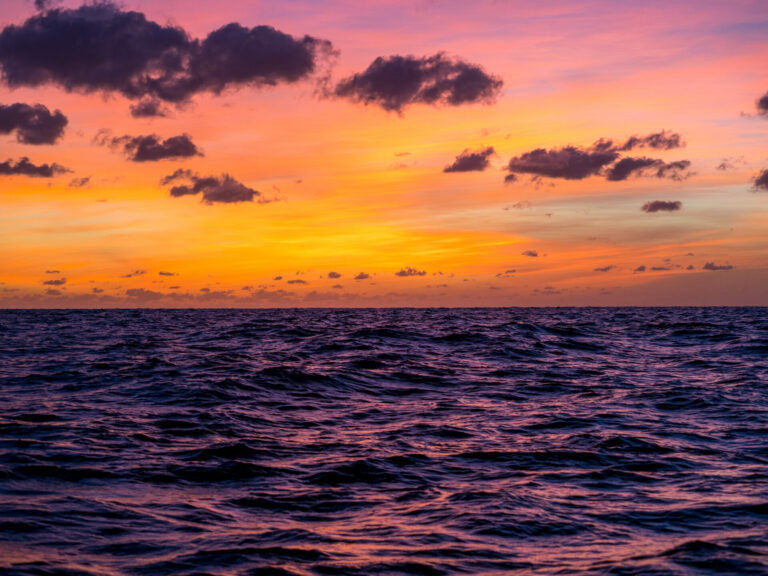
Applied Mathematics and Computational Sciences
Going likelihood-free with neural networks
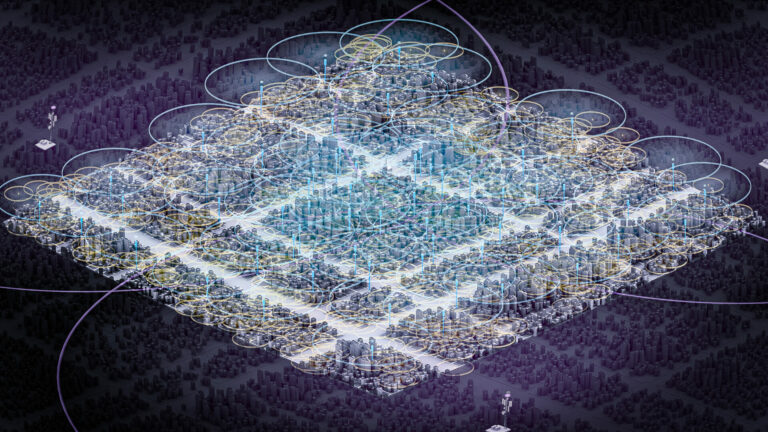
Applied Mathematics and Computational Sciences
A simple solution for frequency sharing
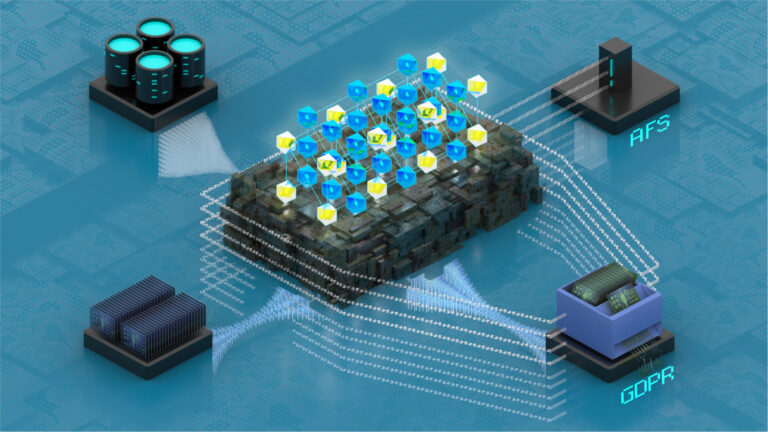
Bioengineering