Statistics
Getting the inside track on street design
Large-scale analysis of pedestrian data from three European cities examines how building density and street design influence pedestrian behavior.
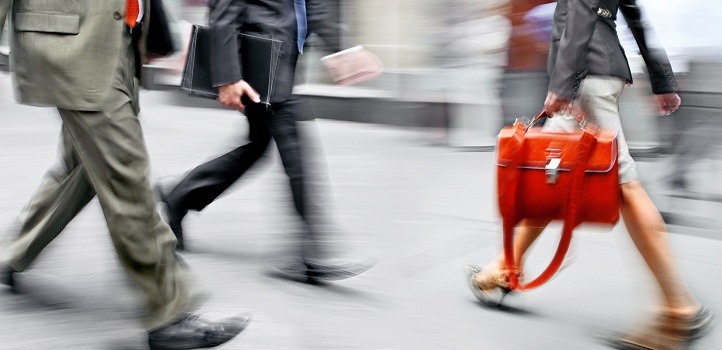
Pedestrian movements are tricky to track, but now the first large-scale statistical analysis of pedestrian flow using anonymous phone data collected in three European capital cities, London, Amsterdam and Stockholm, has been conducted by researchers from KAUST with Swedish colleagues from Gothenburg.
Analyzing the flow of pedestrians through city streets provides insights into how city design influences walking behavior. Studies of pedestrian flow inform new urban developments, enable designers to define quieter areas and “urban buzz” zones and reveal how spaces are used at different times.
“In a previous study, we found strong links between the total number of people walking on a given street in one day and certain characteristics of the urban environment,” says David Bolin at KAUST. Specifically, built density type, which is a variable based on the total floor space and ground space taken up by buildings on a street, correlated with the intensity of pedestrian flow, while the relative position of each street in a city — its “centrality” or street type — explained flow variations within each area.
Many similar studies have been hampered by methodological inconsistencies and small datasets, but this one had a large dataset.
“We took advantage of the power of large-scale data collection to determine if these same variables (density and street type) could explain both the full-day counts in different streets and the variations in flow over the day,” says Bolin. “We developed a functional ANOVA model to explore our results.”
Data was collected over three weeks in October 2017 from detection devices on almost 700 street segments across 53 neighborhoods. The detectors collected anonymous signals from mobile phones traveling at under 6 kilometers per hour to differentiate pedestrians from people traveling on transport.
“We chose streets that provided a wide mix of street types and density types from each city,” says Bolin.
Daily total pedestrian counts were influenced by built density, street type and a street’s “attraction variables,” such as the presence of local markets or public transport stops. Built density explained the fluctuations in flow across the day but street type did not. There were also differences between each city, especially in the highest density built-up areas, making it difficult to generalize the findings to other cities. The model predicted pedestrian flow for certain parts of the three cities better than others.
“The results provide insights into the importance of street and density types in designing areas with different qualities,” says Bolin. “Accurate predictions for other cities would require more data from multiple cities in different seasons.”
References
-
Bolin, D., Verendel, V., Berhauser Pont, M., Stavroulaki, I., Ivarsson, O. & Håkansson, E. Functional ANOVA modelling of pedestrian counts on streets in three European cities. Journal of the Royal Statistical Society Series A 184, 1176-1198 (2021).| article
You might also like
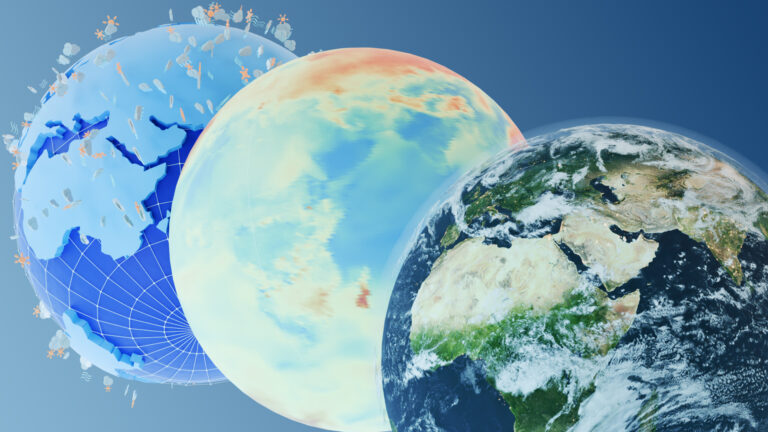
Statistics
A high-resolution boost for global climate modeling
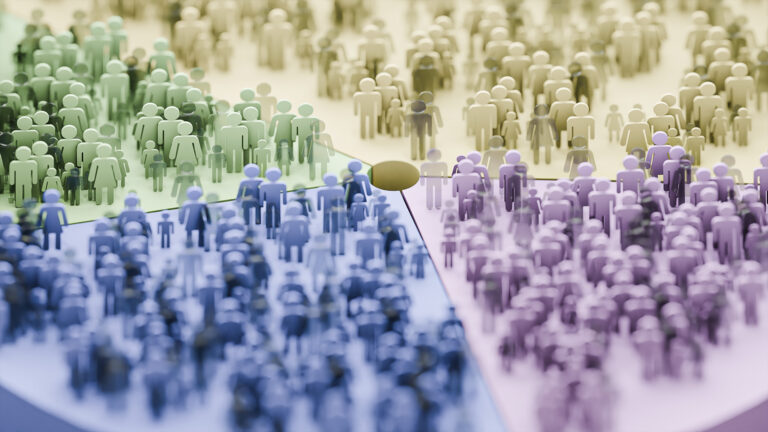
Applied Mathematics and Computational Sciences
Finer forecasting to improve public health planning
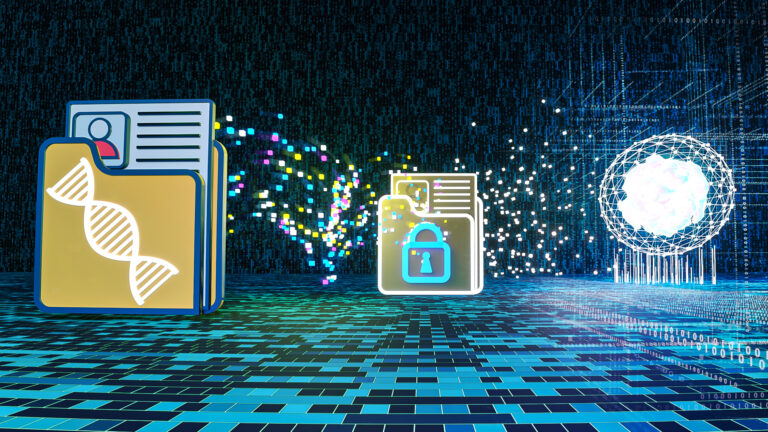
Bioengineering
Shuffling the deck for privacy
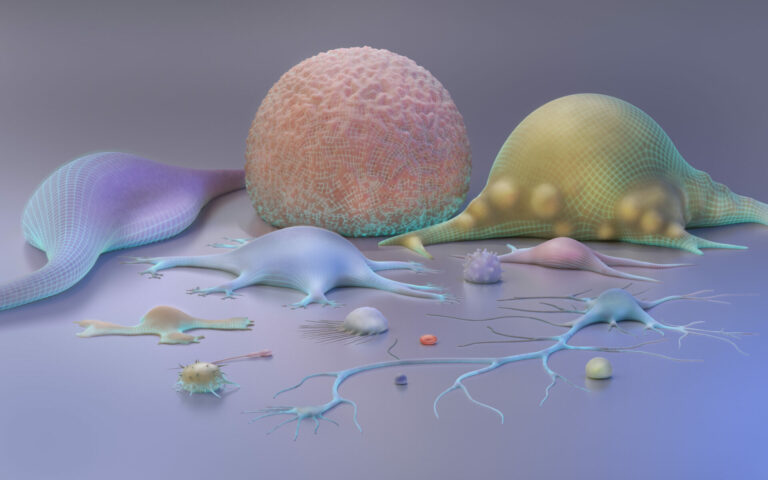
Bioengineering
AI for cells helps illuminate their identity
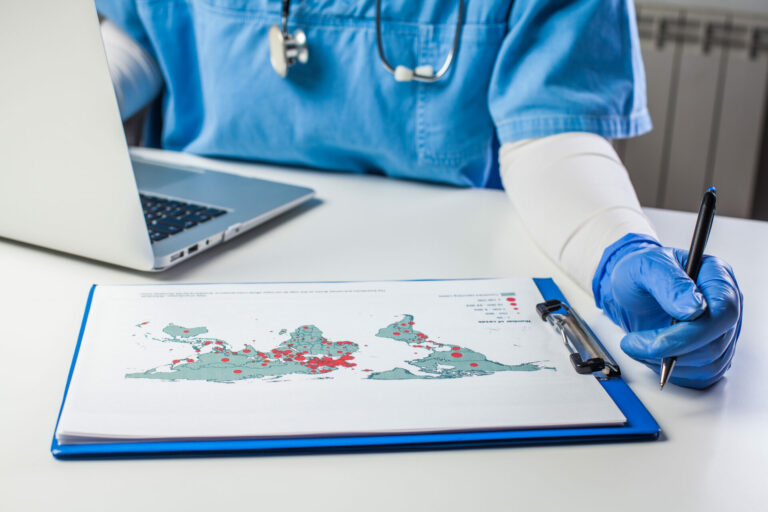
Applied Mathematics and Computational Sciences
Global look at sex differences in young people's mortality
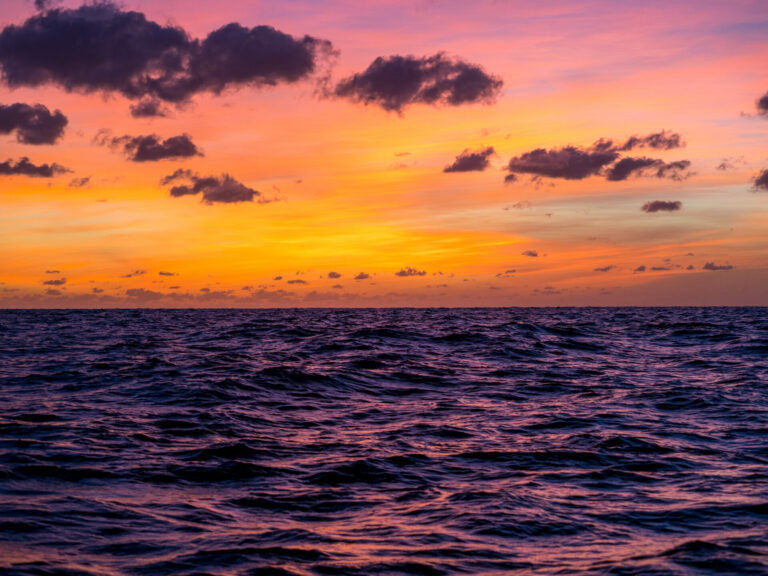
Applied Mathematics and Computational Sciences
Going likelihood-free with neural networks
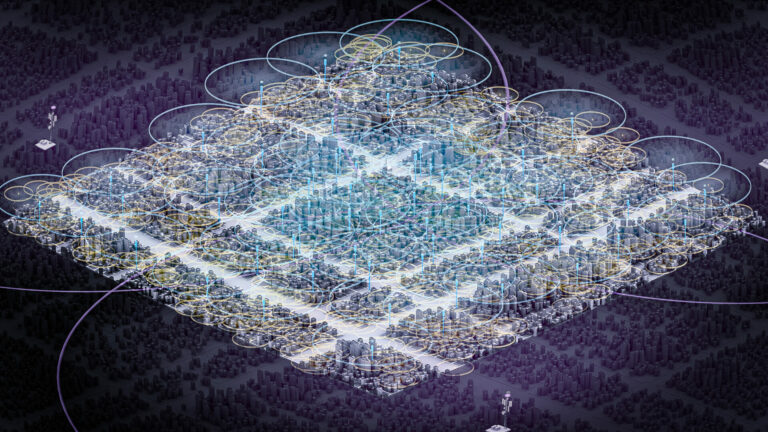
Applied Mathematics and Computational Sciences
A simple solution for frequency sharing
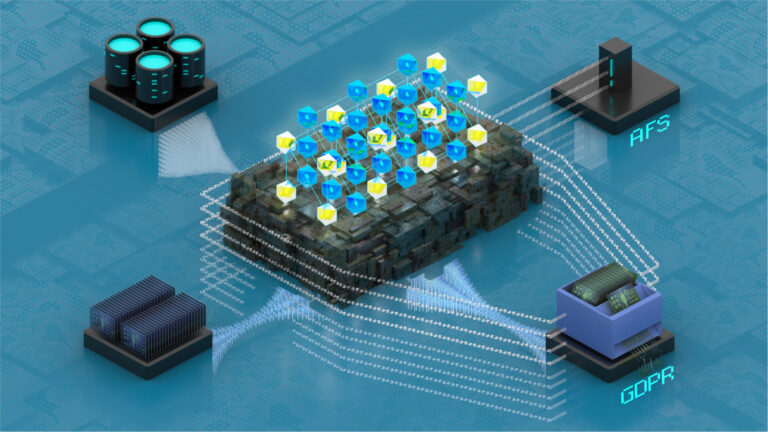
Bioengineering