Statistics
A brainwave from social networks
The statistics used to understand social networks reveal the diversity of functional connections in the brain.
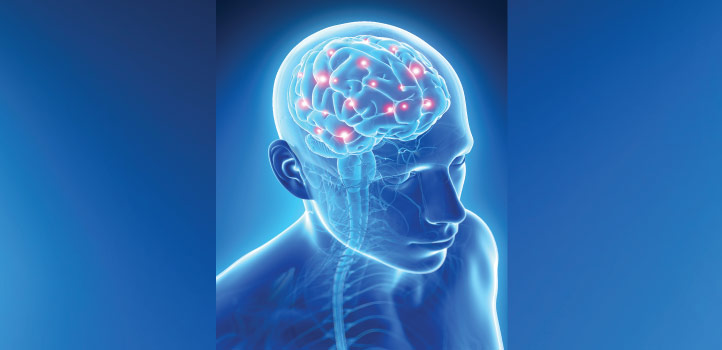
Analyzing brain activity across multiple subjects using sophisticated statistics has produced a model that better captures the diversity and dynamics of brain function. The method, developed by KAUST researchers, could help us better understand human cognition and identify the abnormal brain states that underlie many neurological diseases.
Neuroscientists use a noninvasive technique called functional magnetic resonance imaging (fMRI) to measure the activation of neurons in the brain by detecting localized changes in blood flow and blood oxygen in different brain regions. This has allowed researchers to see networked “communities” of neuronal clusters or nodes that are densely connected and respond to the same stimuli.
“The current state-of-the-art approach is to use a stochastic ‘block model’ to explain brain networks, which gives only a static description of brain function and is not realistic because brain function changes dynamically as the brain responds to new stimuli,” says group leader Hernando Ombao. “So we set out to develop a new unified statistical framework that can characterize the community structure of brain functional networks and help understand how they vary across individuals.”
Current models of the brain’s community network structure suggest that much of brain function is assortative, meaning that brain function is segregated into separate specialized information-processing regions in the brain. Although the integration of information from specialized brain regions is also known to be important in brain function, this has been very difficult to observe.
“The problem has been that brain function is traditionally analyzed by fitting models to individual subjects, which can capture localized or ‘assortative’ community structures,” explains Ombao. “But this is not well suited to picking the nonassortative integration functions, which require information to be mapped and compared among different subjects under different stimuli.”
To solve this problem, the research team — including KAUST student Meini Tang, long-time collaborator Chee-Ming Ting from Monash University in Malaysia and former KAUST postdoctoral fellow Balqis Samdin, who led the study — used a “multilayer modularity maximization method.” Recently proposed for social networks, the method has been adapted by the team to detect common brain community structures that are shared across subjects.
“Our model has revealed a more diverse community organization in addition to the typical assortative structure in brain networks associated with language processing and motor functions,” says Samdin.
“For example, we found a core-like community in the language network that seems to serve an integrative function between periphery communities in the left and right hemispheres during language comprehension,” says Samdin. “Our model is able to capture more complex intercommunity interactions related to a wider functional repertoire of brain function.”
References
-
Ting, C.-M. Samdin, S.B., Tang, M. & Ombao, H. Detecting dynamic community structure in functional brain networks across individuals: A multilayer approach. IEEE Transactions on Medical Imaging 40, 468 – 480 (2020).| article
You might also like
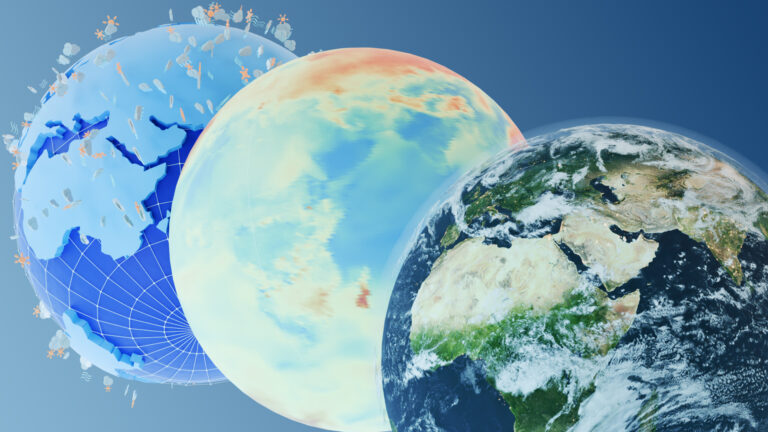
Statistics
A high-resolution boost for global climate modeling
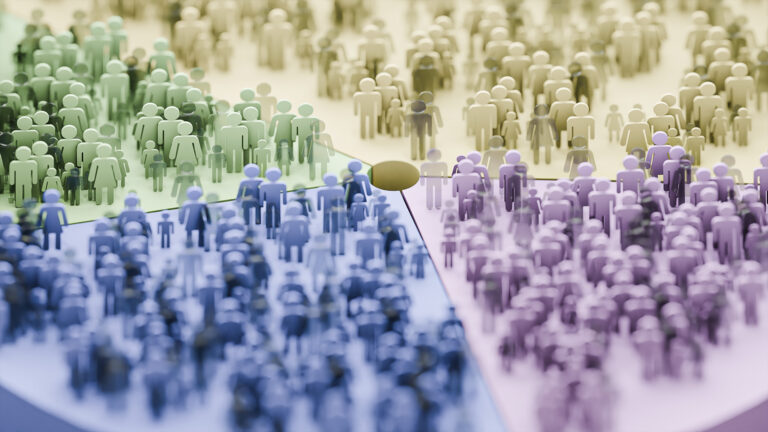
Applied Mathematics and Computational Sciences
Finer forecasting to improve public health planning
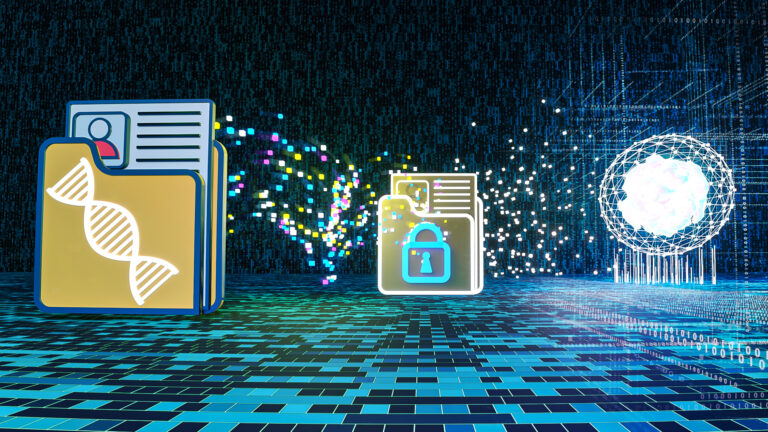
Bioengineering
Shuffling the deck for privacy
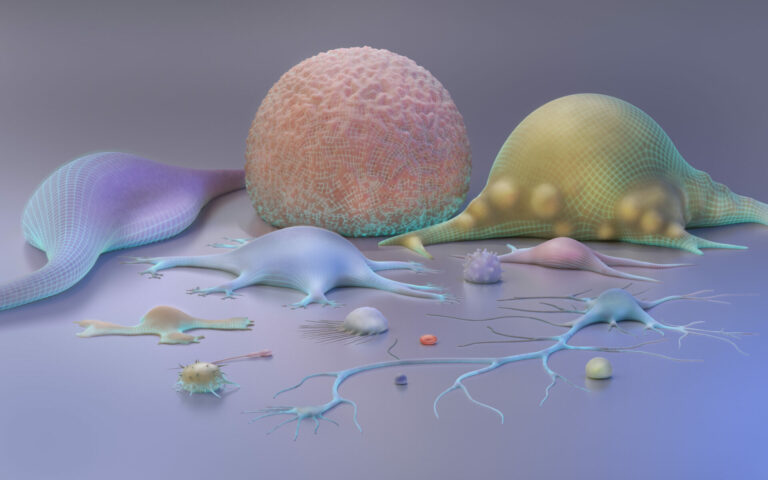
Bioengineering
AI for cells helps illuminate their identity
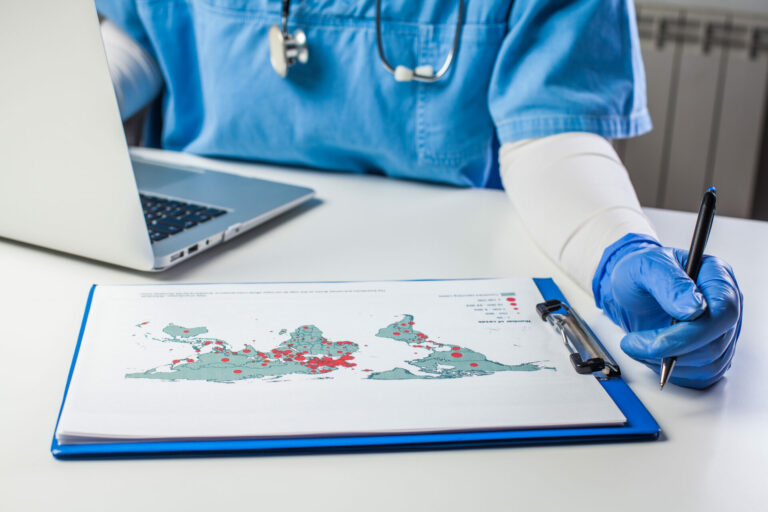
Applied Mathematics and Computational Sciences
Global look at sex differences in young people's mortality
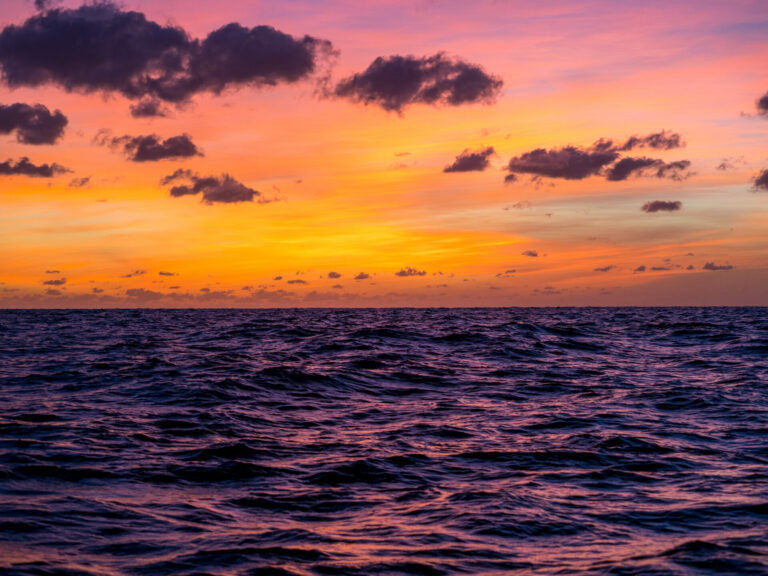
Applied Mathematics and Computational Sciences
Going likelihood-free with neural networks
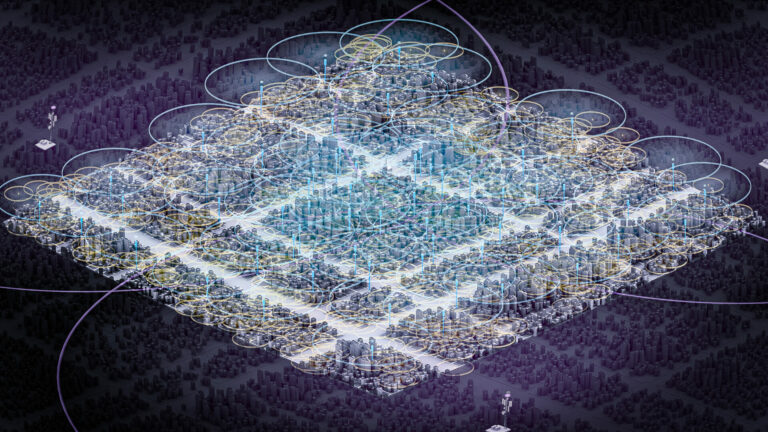
Applied Mathematics and Computational Sciences
A simple solution for frequency sharing
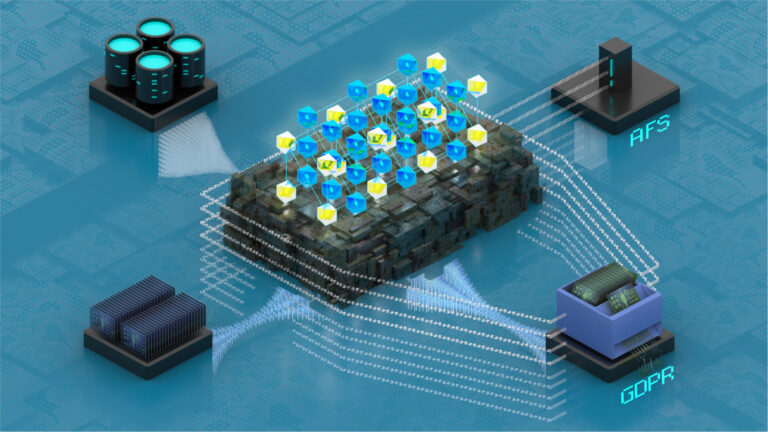
Bioengineering