Computer Science
Divided attention could ease wireless congestion
Predicting wireless traffic using artificial intelligence could improve the reliability of future wireless communications.
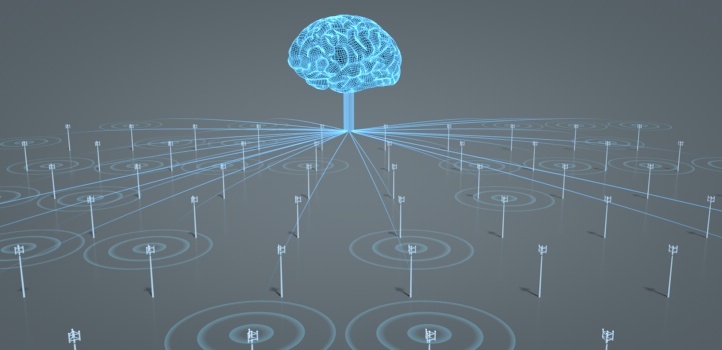
The prediction of future wireless traffic volumes using artificial intelligence (AI) would allow communication systems to automatically adjust network resources to maximize reliability. KAUST researchers have now developed a more accurate “dual attention” prediction scheme that minimizes the volume of prediction data that needs to be transferred across the network.
With 5G wireless communication technology now being deployed around the world, researchers are looking ahead to what 6G could offer. One emerging idea is to use AI to coordinate communication resources by learning from historical patterns of network usage across the network over time. The main problem is that the transmission of usage data from nodes to a central database — where the AI can do its magic — introduces a substantial bandwidth overhead that negates much of the potential benefits.
Chuanting Zhang and colleagues Shuping Dang, Basem Shihada and Mohamed-Slim Alouini addressed this issue by decentralizing the prediction model.
“Wireless traffic prediction could play a central role in network management as the basis for intelligent communication systems,” says Zhang. “AI techniques such as deep neural networks are able to accurately model the complicated spatio-temporal nonlinear correlations in wireless traffic. However, as different base stations can have very different traffic patterns, it is quite challenging to develop a prediction model that performs well on all base stations at once.”
Zhang’s team developed a hierarchical “dual attention” scheme that combines a central global model with local models at each base station. Their scheme weighs the influence of the local models according to network location and then sends only a limited amount of information from the base stations at each update. The result is a hybrid, low-overhead prediction model that provides a high-quality forecast of the spatial and temporal change in network usage over time.
The framework — called FedDA or dual attention-based federated learning — also enables clustering of base stations based on geolocation to obtain further efficiencies and improvements in prediction accuracy. Using two datasets, the researchers demonstrated that FedDA delivers consistently better prediction performance than other methods for SMS messaging, calls and internet traffic.
“With this method, we have decentralized wireless traffic prediction and also implemented dual-attention global model optimization by paying attention to both the current knowledge of the central server and the information of local clients.” says Zhang. “Each updated global model can then be deployed to each base station to predict and adapt to new traffic patterns.”
References
-
Zhang, C. Dang, S, Shihada B. & Alouini, M. -S. Dual attention-based federated learning for wireless traffic prediction. IEEE Co ference on Computer Communications, published online 26 July 2021.| article
You might also like
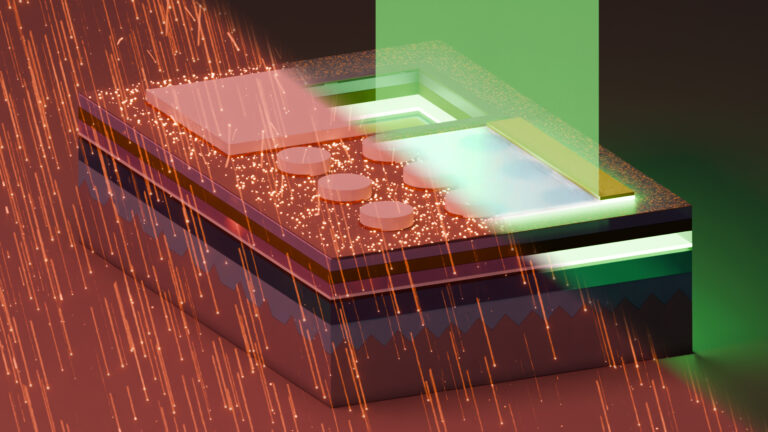
Computer Science
A new path to high-efficiency micro-LEDs
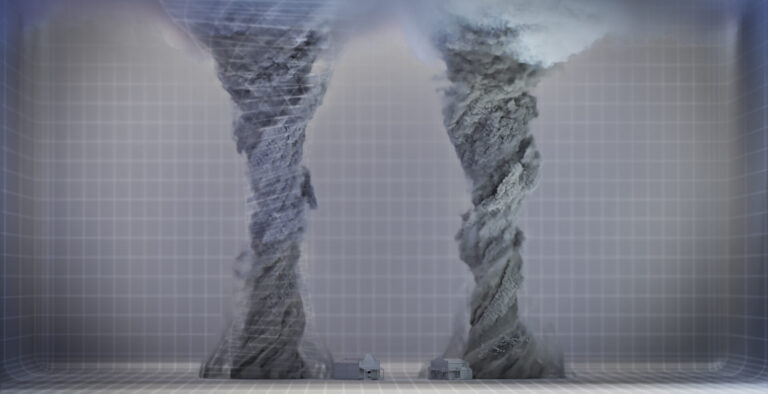
Computer Science
Cyclones meet their mathematical match
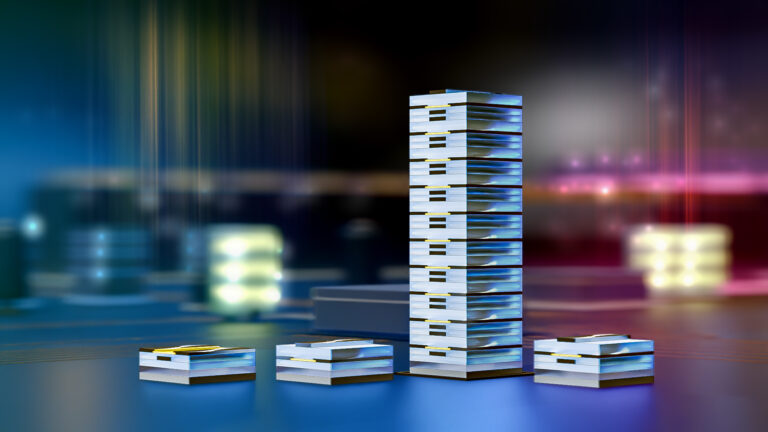
Applied Physics
Onward and upward to smaller faster devices
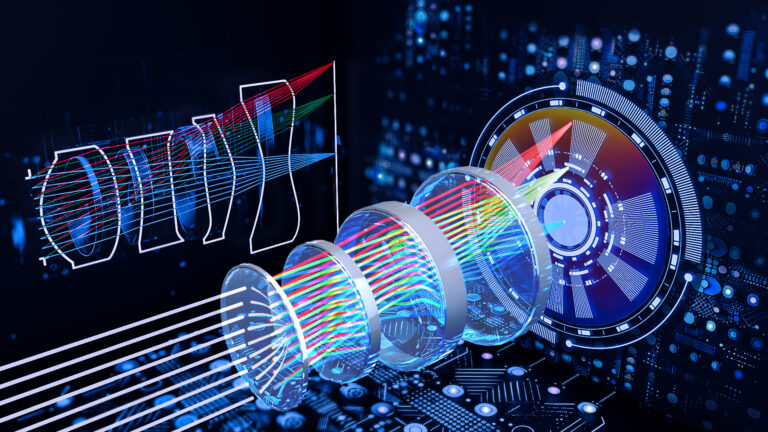
Computer Science
Deep learning improves lens design
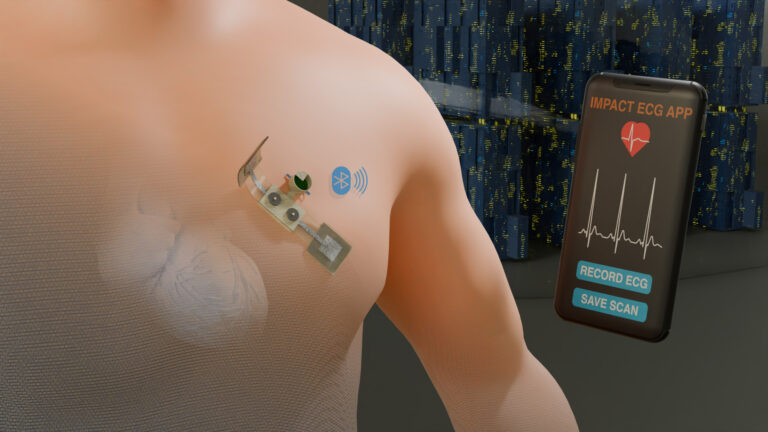
Computer Science
Better connection for monitoring helps heart patients and healthcare staff
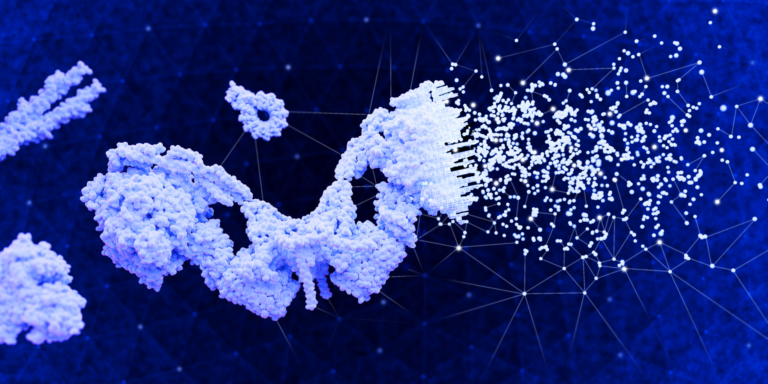
Bioengineering
AI tool predicts function of unknown proteins
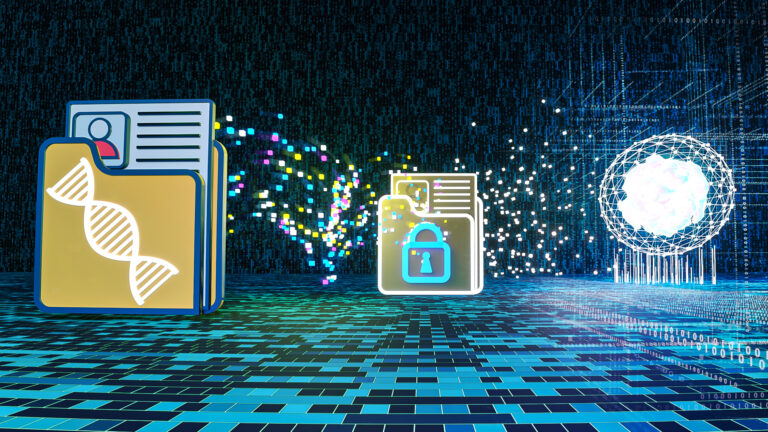
Bioengineering
Shuffling the deck for privacy
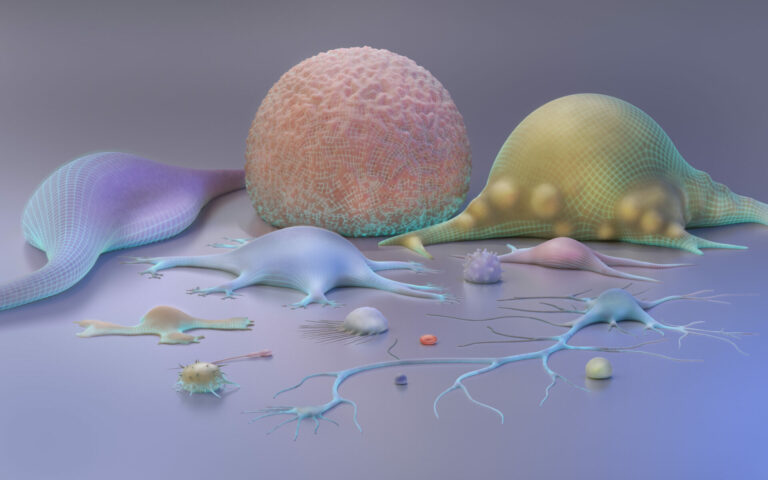
Bioengineering