Computer Science
Simulating evolution to understand a hidden switch
Some organisms evolve an internal switch that can remain hidden for generations until stress flicks it on.
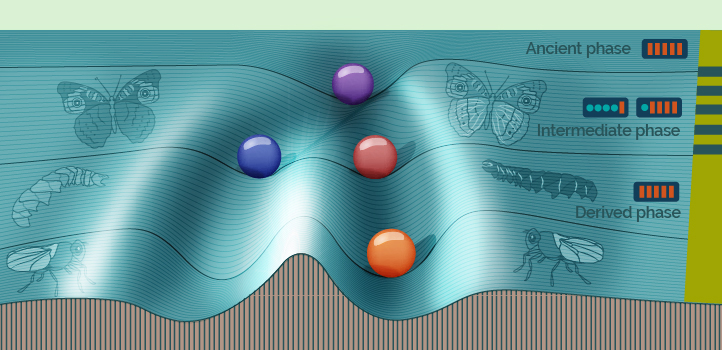
Computer simulations of cells evolving over tens of thousands of generations reveal why some organisms retain a disused switch mechanism that turns on under severe stress, changing some of their characteristics. Maintaining this “hidden” switch is one means for organisms to maintain a high degree of gene expression stability under normal conditions.
Tomato hornworm larvae are green in warmer regions, making camouflage easier, but black in cooler temperatures so that they can absorb more sunlight. This phenomenon, found in some organisms, is called phenotypic switching. Normally hidden, this switching is activated in response to dangerous genetic or environmental changes.
Scientists have typically studied this process by investigating the changes undergone by organisms under different circumstances over many generations. Several years ago, for example, a team bred generations of tobacco hornworm larvae to observe and induce color changes similar to those that occurred in their tomato hornworm relatives.
“Computer simulations, when built on reasonable assumptions and conducted under careful control, are a very powerful tool to mimic the real situation,” says KAUST computational bioscientist Xin Gao. “This helps scientists observe and understand principles that are otherwise very difficult, or impossible, to observe by wet-lab experiments.”
Gao and KAUST research scientist Hiroyuki Kuwahara designed a computer simulation of the evolution of 1,000 asexual microorganisms. Each organism was given a gene circuit model for regulating the expression of a specific protein X.
The simulation evolved the population over 90,000 generations. The original founding population had identical nonswitching gene circuits and evolved over 30,000 generations, collectively called the ancient population, under stable conditions. The next 30,000 generations, called the intermediate population, were exposed to fluctuating environments that switched every 20 generations. The final 30,000 generations, the derived population, were exposed to a stable environment.
The individuals in the ancient and derived populations, who evolved in stable environments, both had gene expression levels that were optimized for stability. But they were different: the ancient population’s stability did not involve phenotypic switching, while the derived population’s did. The difference, explains Kuwahara, stems from the intermediate population, in which switching was favored in order to deal with the fluctuating conditions.
The simulations suggest that populations of organisms maintain their switching machinery over a long period of environmental stability by gradually evolving low-threshold switches, which easily switch in fluctuating circumstances, to high-threshold switches when the environment is more stable.
This is easier, says Kuwahara, than reverting to a nonswitching state through small mutational shifts. “Instead, we end up with a type of ‘hidden’ phenotypic switching that acts like an evolutionary capacitor, storing genetic variations and releasing alternative phenotypes in the event of substantial perturbations,” Kuwahara says.
The team next plans to use computer simulations to study more complex biological systems while also interactively collaborating with researchers conducting wet-lab experiments. Their aim is to develop theoretical frameworks that can be experimentally validated.
References
- Kuwahara, H. & Gao, X. Stable maintenance of a hidden switch as a strategy to increase the gene expression stability. Nature Computational Science 1, 62–70 (2021).| article
You might also like
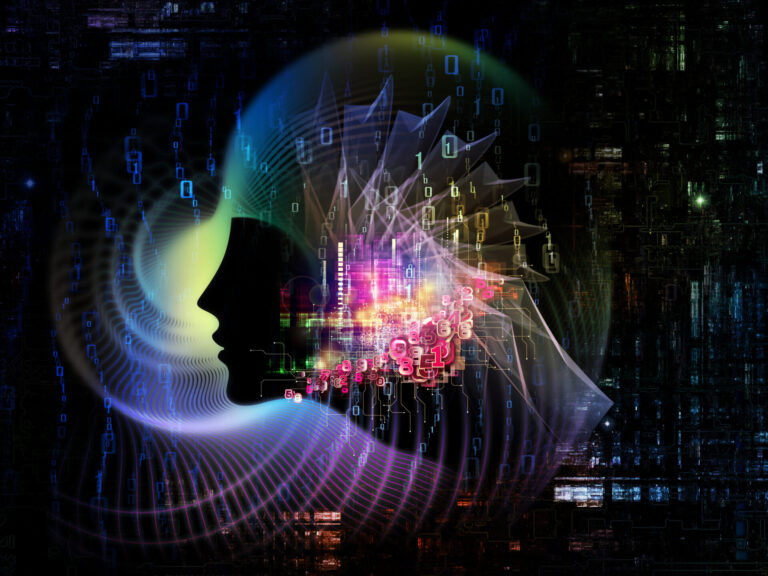
Computer Science
A blindfold approach improves machine learning privacy
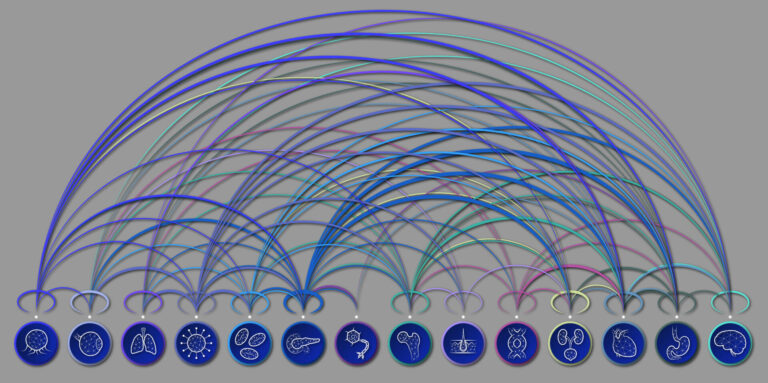
Computer Science
AI tool maps hidden links between diseases
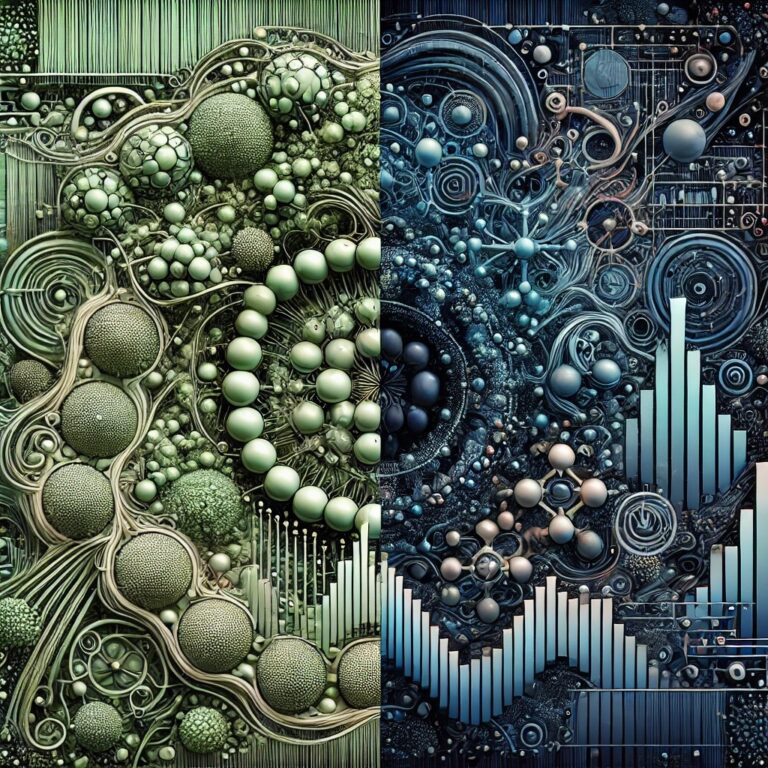
Bioscience
The theory of everything that wasn’t
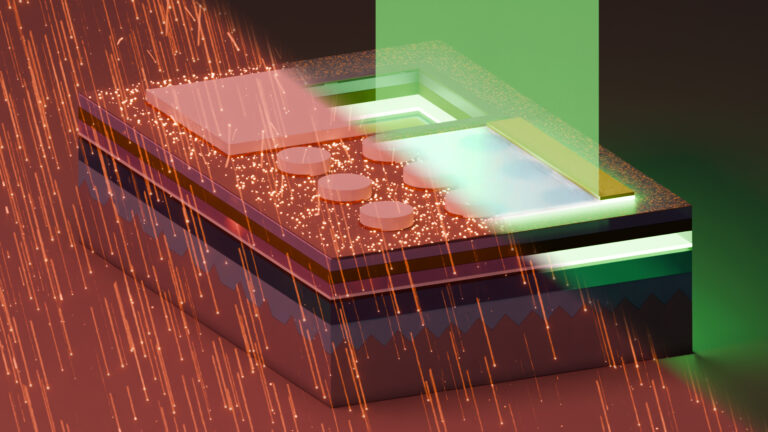
Computer Science
A new path to high-efficiency micro-LEDs
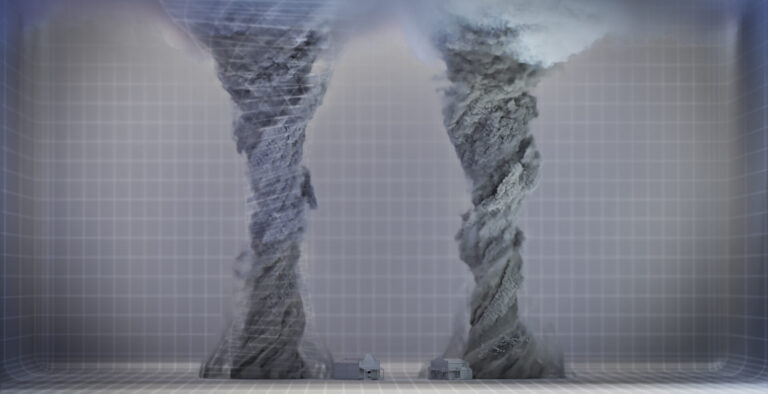
Computer Science
Cyclones meet their mathematical match
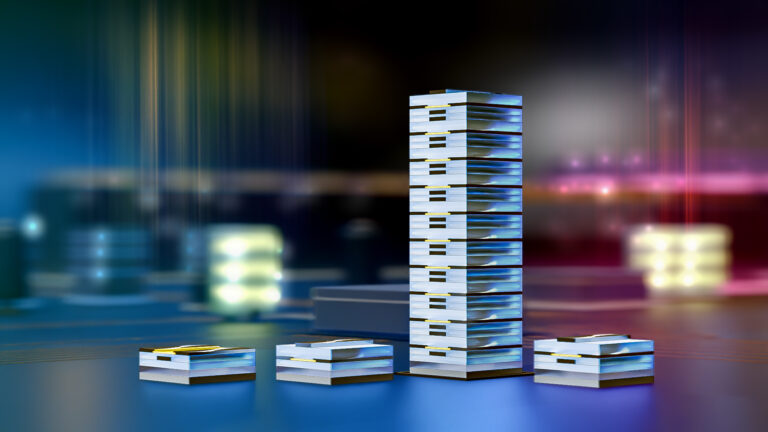
Applied Physics
Onward and upward to smaller faster devices
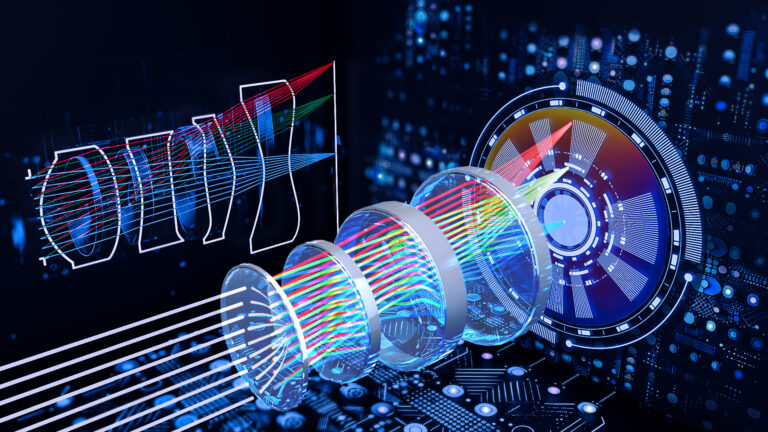
Computer Science
Deep learning improves lens design
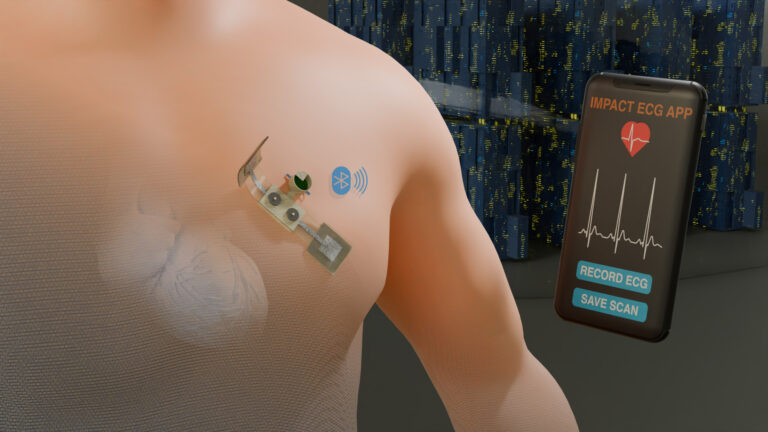
Computer Science