Applied Mathematics and Computational Sciences
Enhancing understanding of genetic regulation
Researchers round up clues to track down enhancers.
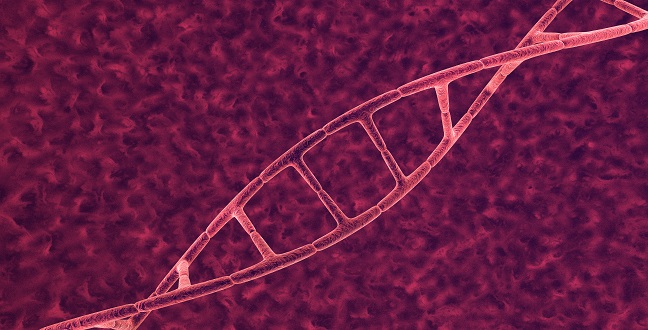
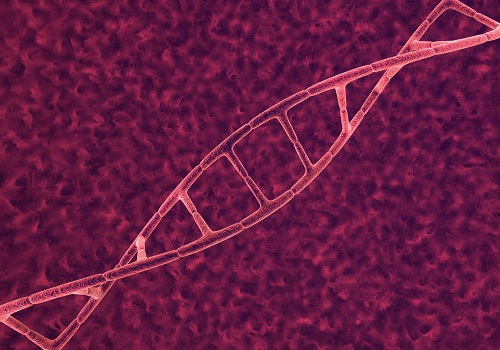
The review surveyed more than 30 computational tools and methods for identifying regulatory elements known as enhancers.
© 2016 Alamy BC3RBA
Our understanding of genetic regulation would be improved by better knowledge of regulatory elements known as enhancers, which are not located alongside the genes they control. In a recent paper published in Briefings in Bioinformatics, KAUST researchers reviewed the differences between various computational approaches to this problem and outlined some of the major challenges in the field1.
Improving our understanding of genetic regulation will have major biotechnological and medical implications, explained Vladimir Bajic from the University’s Computational Bioscience Research Center. Bajic is also the senior author of the paper.
The paper, which has been included in the International Society for Computational Biology’s list of recommended educational and training resources, surveyed more than 30 computational tools and methods for identifying enhancers.
“Each of them is based on different assumptions, and they do not produce the same or very similar results,” said Bajic. “The fact that there are so many methods indicates that there isn’t a single one which is very good, because then the others would have been sidelined.”
The development and evaluation of these tools is hindered by the lack of a “gold standard” dataset against which to test them. Producing such a dataset is a significant experimental challenge, particularly since it would have to include the full diversity of enhancer types. Bajic is confident, however, that the rapid advance of experimental biology will resolve the problem in the next few years.
While none of the tools is ideal, they have successfully identified enhancers, especially in studies with sufficient data to combine several approaches. In addition to making specific predictions, research with these tools has also generated broader insights about enhancers. For example, computational analysis revealed that the regions around predicted enhancers are enriched in binding sites for specific transcription factors.
The development of these tools can also help solve more general issues in machine learning, such as a problem known as class imbalance. If one of two classes is much more common, predictive classification algorithms can score well simply by consistently guessing the more common class, and thus often end up being “right” simply by chance. The approaches used to overcome this issue in identifying enhancers can also be applied in other domains facing the same challenge.
“This is an unfinished story,” Bajic said. “Without a gold standard, we cannot say which methods are better or worse, but our review should help the community critically assess the approaches and then hopefully invent a better way to deal with this complex problem.”
References
-
Kleftogiannis, D., Kalnis, P. & Bajic, V. Progress and challenges in bioinformatics approaches for enhancer identification. Briefings in Bioinformatics advance online publication, 3 December 2015.| article
You might also like
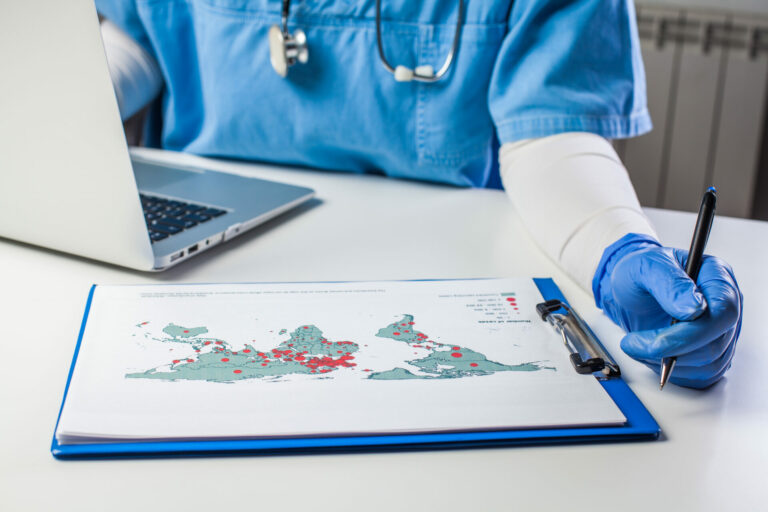
Applied Mathematics and Computational Sciences
Global look at sex differences in young people's mortality
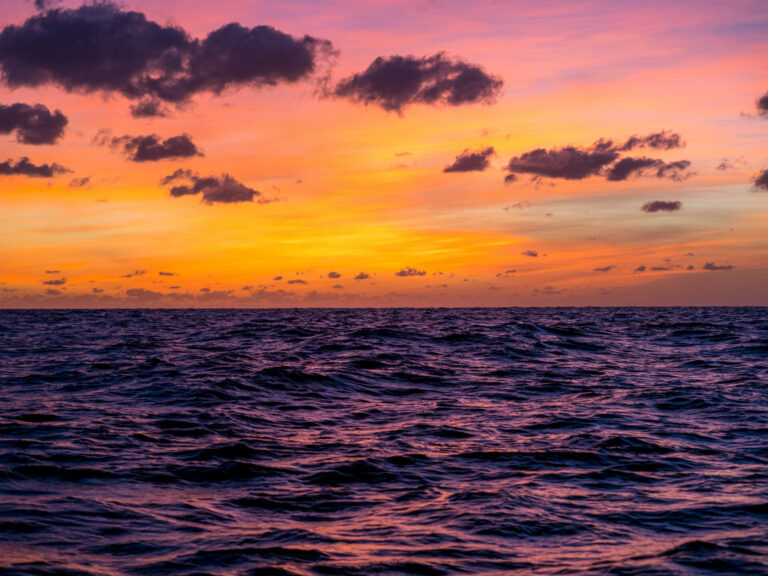
Applied Mathematics and Computational Sciences
Going likelihood-free with neural networks
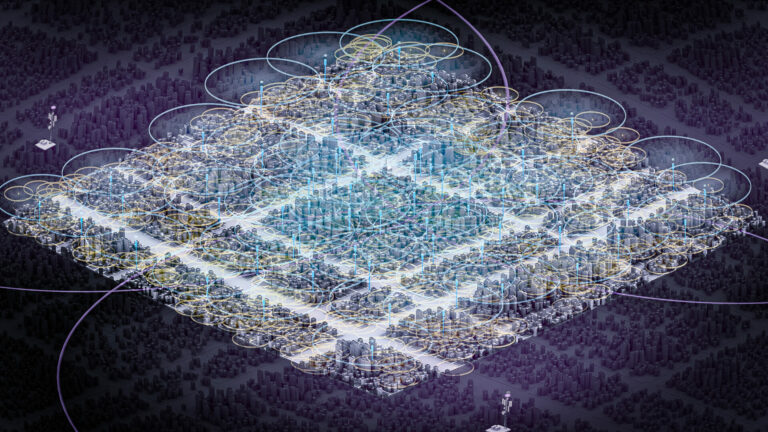
Applied Mathematics and Computational Sciences
A simple solution for frequency sharing
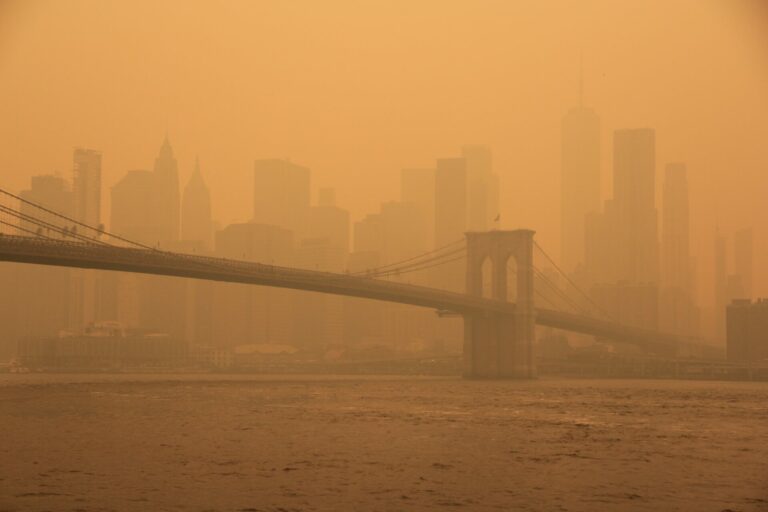
Applied Mathematics and Computational Sciences
Neural networks give deeper insights
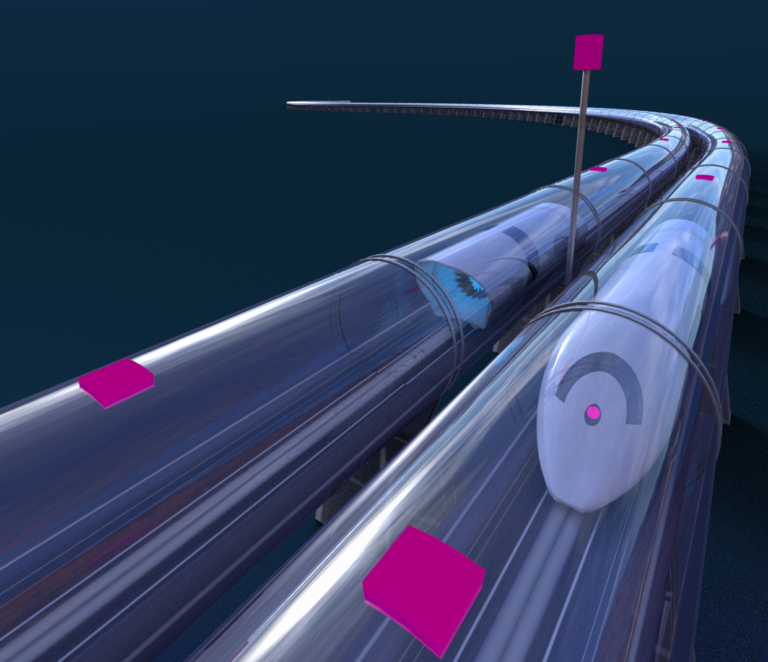
Applied Mathematics and Computational Sciences
Staying online in the high-speed tube of the future
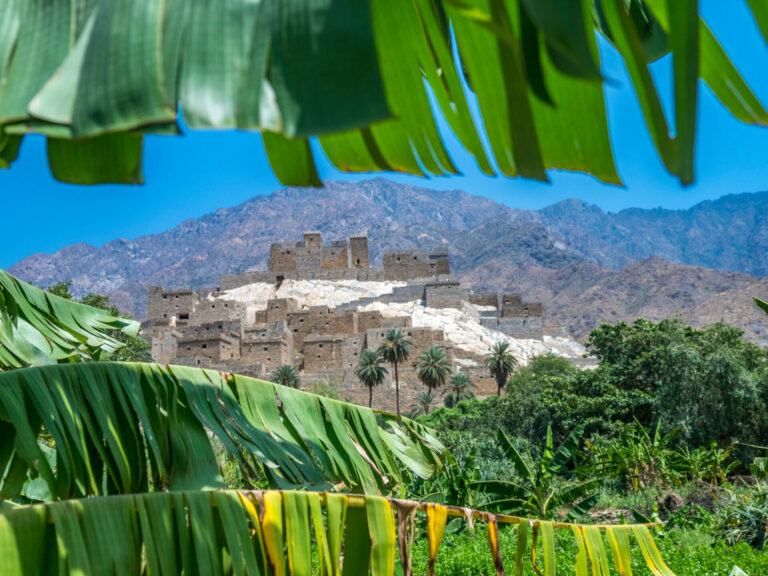
Applied Mathematics and Computational Sciences
Measuring the impact of desert greening
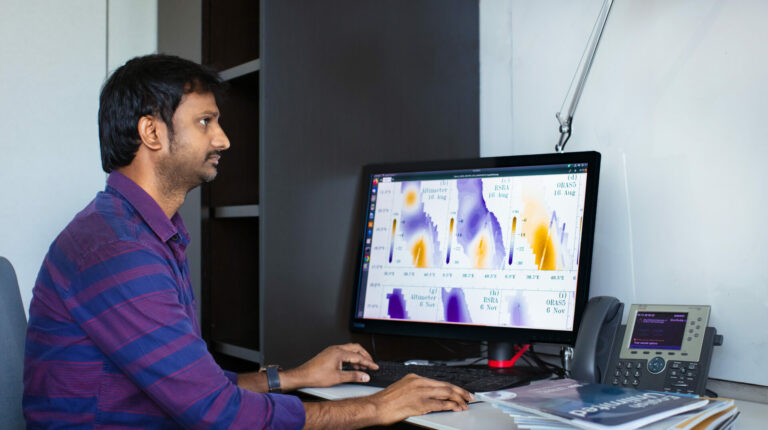
Applied Mathematics and Computational Sciences
Data-driven regional ocean models essential for planning
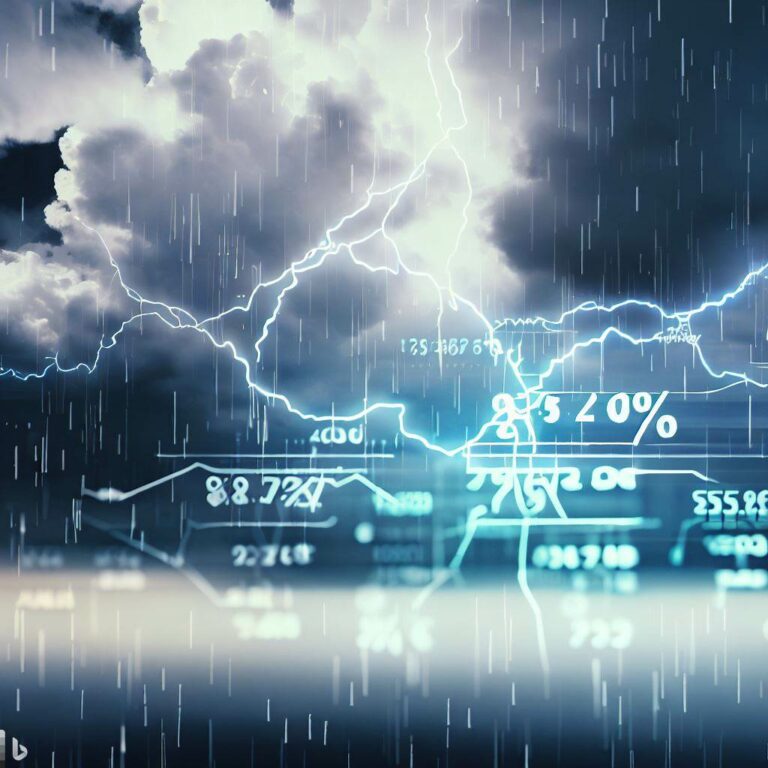
Applied Mathematics and Computational Sciences