Applied Mathematics and Computational Sciences
Taking the guesswork out of experimental design
A fast computational method optimizes sensor measurement networks for noisy, sparsely observed environments.
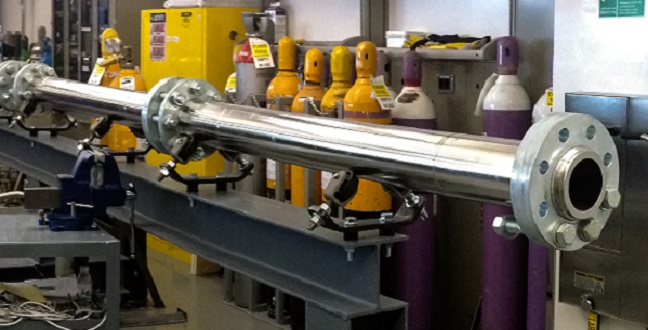
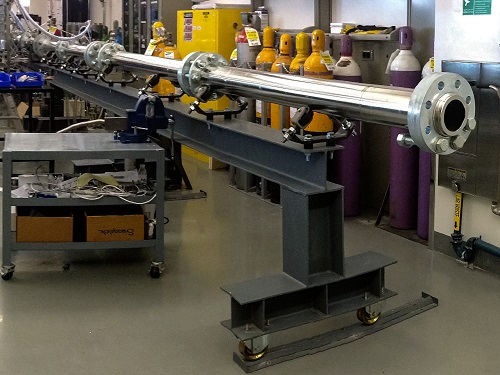
A shock-tube combustion method was used to demonstrate the effectiveness of the optimization method.
© 2015 KAUST
The capture of information about environments and processes is ubiquitous in modern society, from recording weather patterns to measuring traffic density and industrial process parameters. These measurements, when collated, provide data for predictive modelling and process optimization.
Determining exactly how many sensors to use and their optimal location, however, has significant implications for the reliability and value of the information obtained and for the cost of the measurement system itself.
Quan Long, Marco Scavino and Raul Tempone from KAUST, in collaboration with Suojin Wang from Texas A&M University in the United States, have developed a computational method that can quickly derive an optimized ‘experimental design’ for complex, noisy systems with little available data1.
“Experimental design is an important topic in engineering and science,” says Tempone. “It allows us to optimize the locations of sensors to achieve the best estimates and minimize uncertainties, especially for real, noisy measurements.”
Using an established approach known as Bayesian optimization, which combines data and contextual information in a mathematically rigorous framework, Tempone and his colleagues pooled their expertise in computational methods, statistics and numerical analysis of partial differential equations. From this they developed a scheme that could compute an optimized design even for poorly understood or ‘low-rank’ systems.
“As physical systems become increasingly complex, the optimization of experimental design becomes more computationally intensive,” says Tempone. “Our fast method can be used in situations where a large number of parameters need to be estimated while data are sparse.”
Numerical modelling schemes are commonly used to optimize measurement networks by brute-force calculation using real data. The reliability of numerical optimization, however, is only as good as the data used in the computation and — even with today’s supercomputers — the computations can take a very long time. Analytical methods, on the other hand, use computationally efficient equations to describe a system in approximate terms. Analytical schemes also generally require good data, but this obstacle was overcome through the use of some sophisticated mathematics.“
The significance of this work is that we pushed the boundary of an analytical method, called the Laplace approximation, from the conventional Gaussian scenario to low-rank distributions,” says Tempone.
The team demonstrated the effectiveness of their optimization method by applying it to a range of engineering applications, including impedance tomography, shock-tube combustion and seismic inversion. “This new method can be applied in almost any field, from medical imaging to reservoir monitoring and sensor technologies.”
References
Long, Q., Scavino, M., Tempone, R. & Wang, S. A Laplace method for under-determined Bayesian optimal experimental designs. Computer Methods in Applied Mechanics and Engineering 285, 849–876 (2015).| article
You might also like
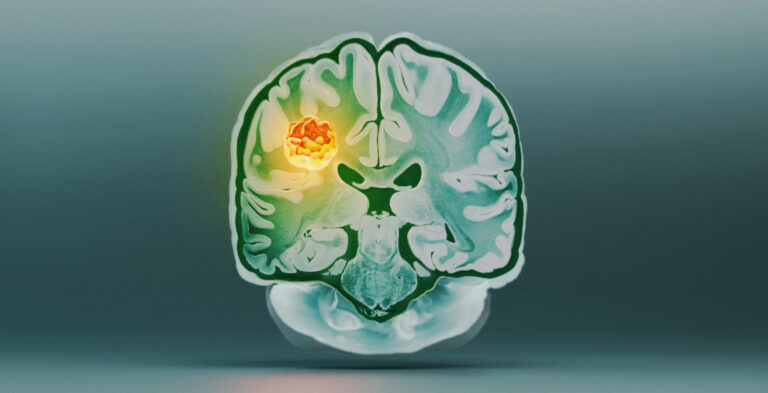
Applied Mathematics and Computational Sciences
Bringing an old proof to modern problems
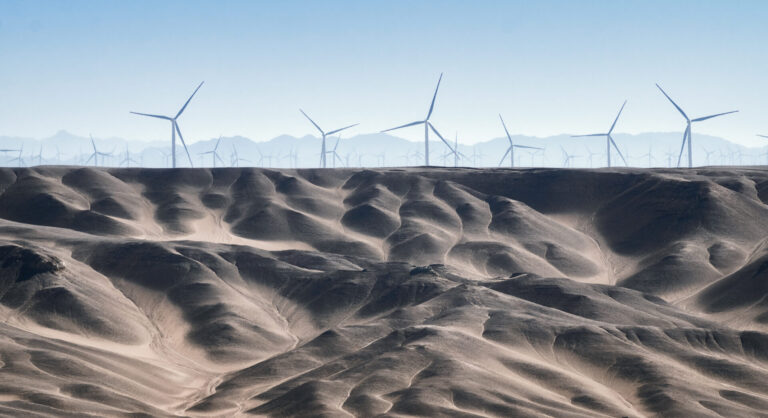
Applied Mathematics and Computational Sciences
Accounting for extreme weather to boost energy system reliability
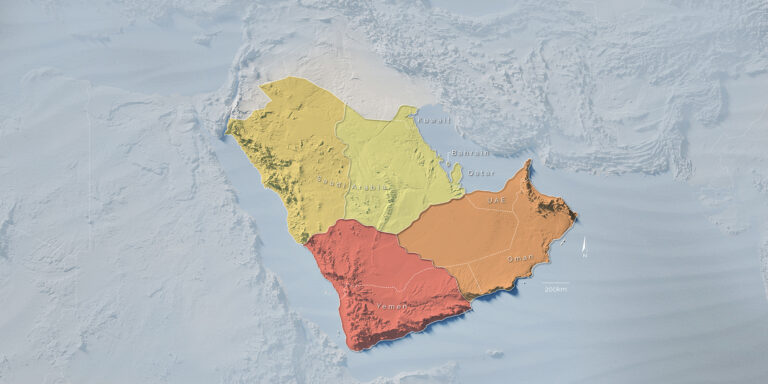
Applied Mathematics and Computational Sciences
Past and future drought patterns across the Arabian Peninsula
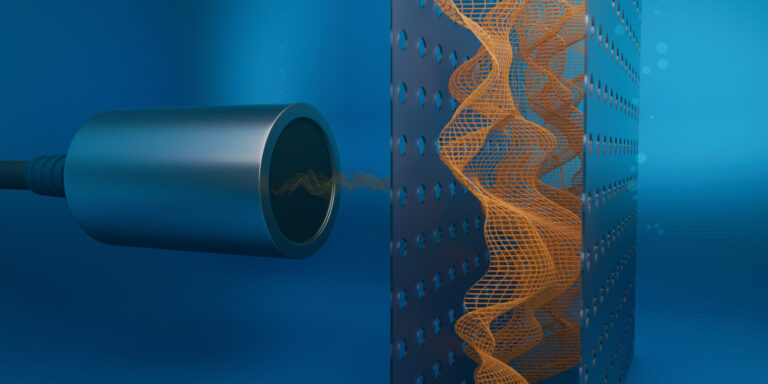
Applied Mathematics and Computational Sciences
New pattern for underwater resonators
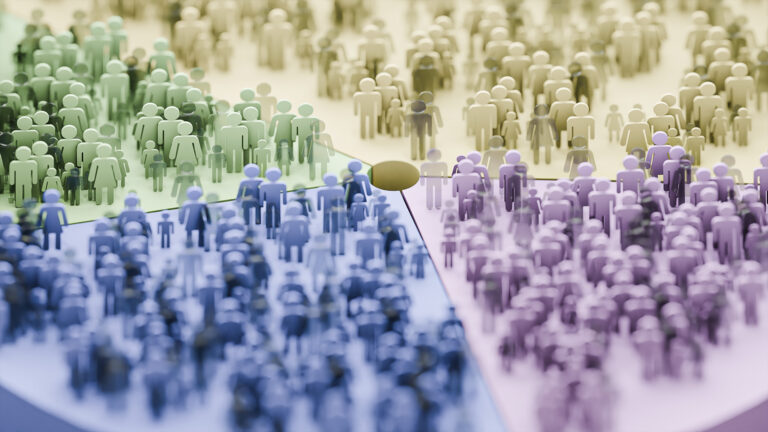
Applied Mathematics and Computational Sciences
Finer forecasting to improve public health planning
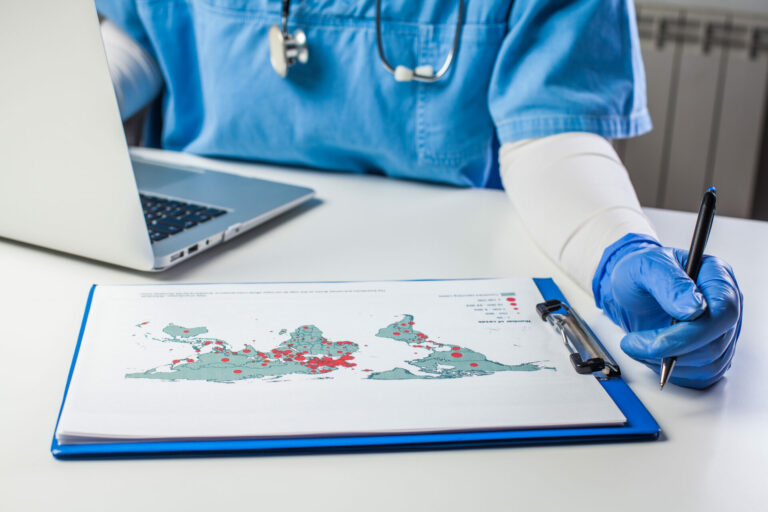
Applied Mathematics and Computational Sciences
Global look at sex differences in young people's mortality
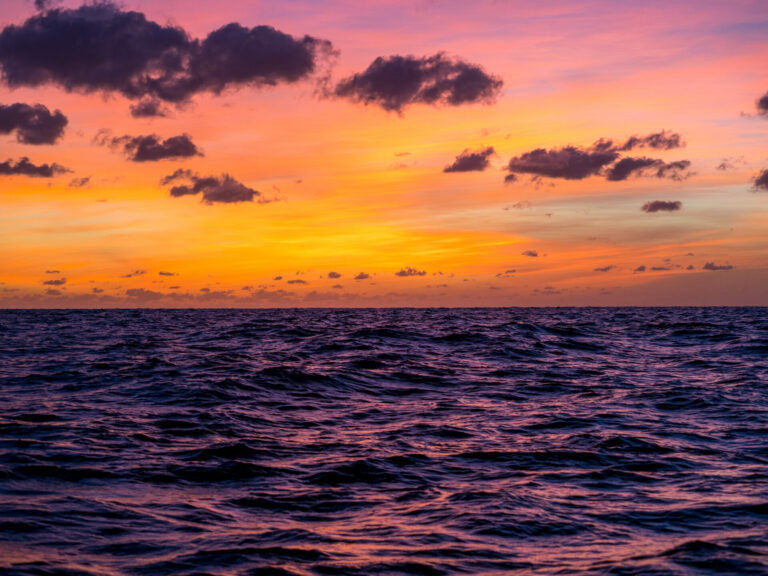
Applied Mathematics and Computational Sciences
Going likelihood-free with neural networks
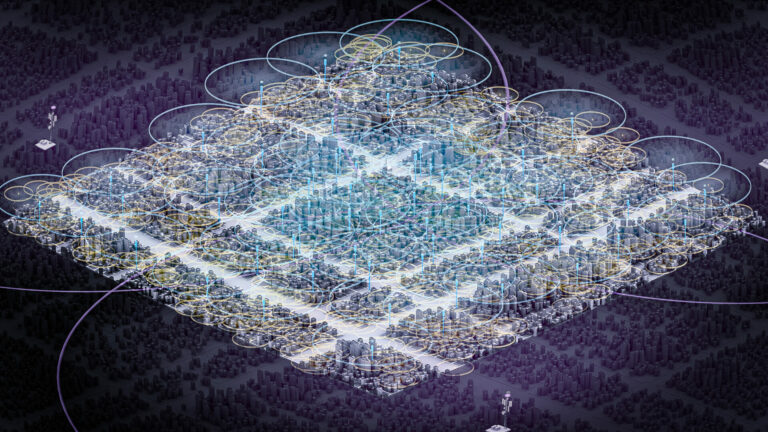
Applied Mathematics and Computational Sciences