Applied Mathematics and Computational Sciences
Faster prediction of wireless downtime
An efficient simulation scheme that hones in on the rarest elements in a dataset can help predict capacity exceedances in wireless networks.
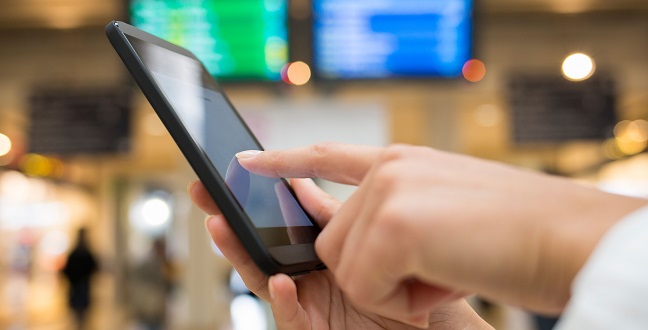
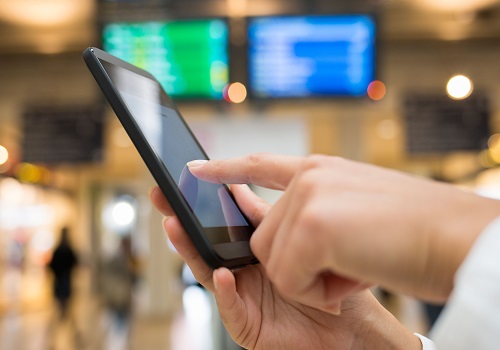
The increasing numbers of mobile devices make it important to predict wireless outage capacity quickly and accurately to minimize service disruptions.
© 2016 Alamy DG2CGW
As the number of mobile devices grows along with demand for faster connections and larger data volumes, wireless networks can easily exceed capacity, resulting in severe network slowdowns and outages. While engineers have developed various sophisticated signal processing methods to accommodate sudden changes in network loads, it has been challenging to evaluate and compare the performance of different approaches in realistic network environments. The reason for this difficulty is that network outages due to capacity saturation can be such rare events that producing simulations to identify outages can be very computationally intensive and take considerable time.
Raul Tempone and colleagues from the KAUST Computer, Electrical and Mathematical Science and Engineering Division have now applied an importance sampling technique that can simulate rare events for the problem of wireless outage capacity1.
“The outage capacity is one of the most important performance metrics of wireless communication systems,” explained Tempone. “It measures the percentage of time that the communication system undergoes an outage, which is typically in the order of one second per million or more. There are no efficient analytical solutions to this problem, and to simulate this situation using conventional simulation methods might take more than a billion simulation runs.”
Motivated by the need for a much faster simulation method, Tempone and his team turned to importance sampling. This is a well-known approach through which a clever problem transformation makes it possible to sample more frequently from the event of interest. This effectively turns rare events in the original problem into non-rare events in the transformed problem. For example, for a typical outage capacity of the order of one in 100 million, the importance sampling approach allows the outage capacity to be estimated in 100 million times fewer simulation runs than conventional methods, dramatically reducing the time needed for estimation.
Unlike existing methods for estimating outage capacity that are only applicable to specific scenarios, the importance sampling approach is generic, making it suitable for a wide range of challenging network scenarios.
“Despite continuous advances in the concept of importance sampling in the field of rare events simulations, its popularity among researchers in the field of wireless communication systems is still quite limited,” Tempone said. “Our work is the first to bridge the gap between the framework of rare event algorithms and the evaluation of outage capacity for wireless communication systems.”
References
-
Rached, N.B., Kammoun, A., Alouini, M.-S., & Tempone, R. Unified importance sampling schemes for efficient simulation of outage capacity over generalized fading channels. IEEE Journal of Selected Topics in Signal Processing 10, 376–388 (2016). | article
You might also like
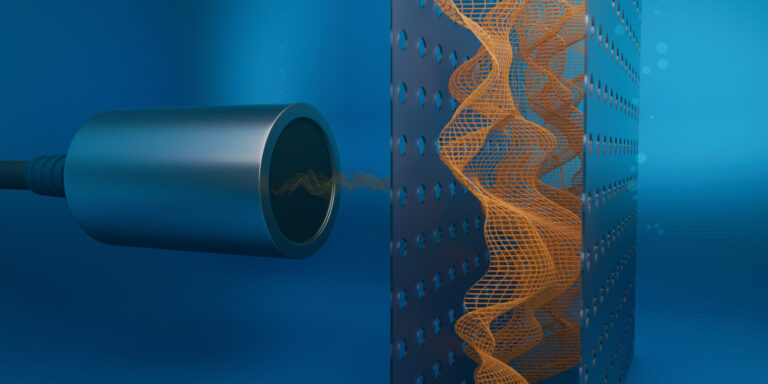
Applied Mathematics and Computational Sciences
New pattern for underwater resonators
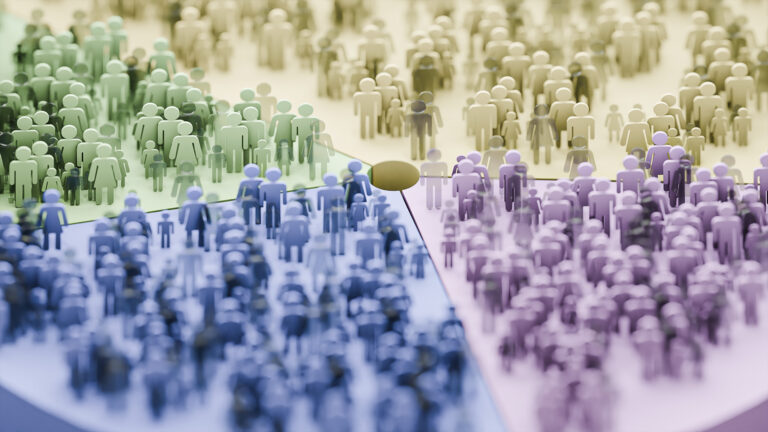
Applied Mathematics and Computational Sciences
Finer forecasting to improve public health planning
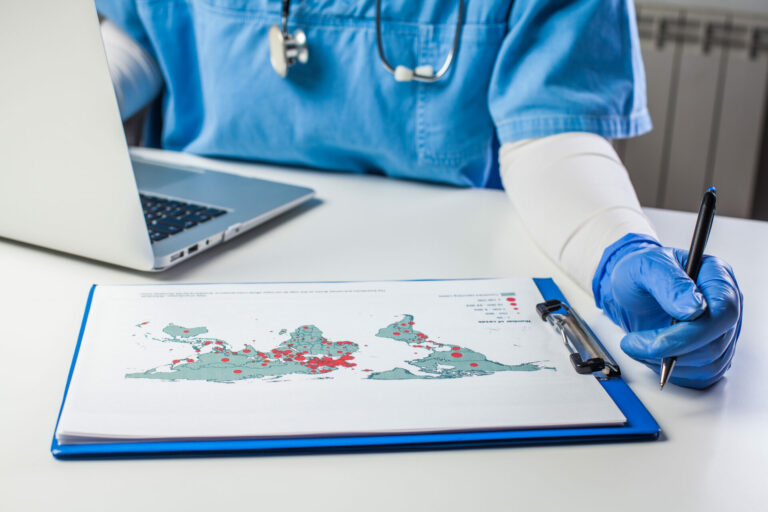
Applied Mathematics and Computational Sciences
Global look at sex differences in young people's mortality
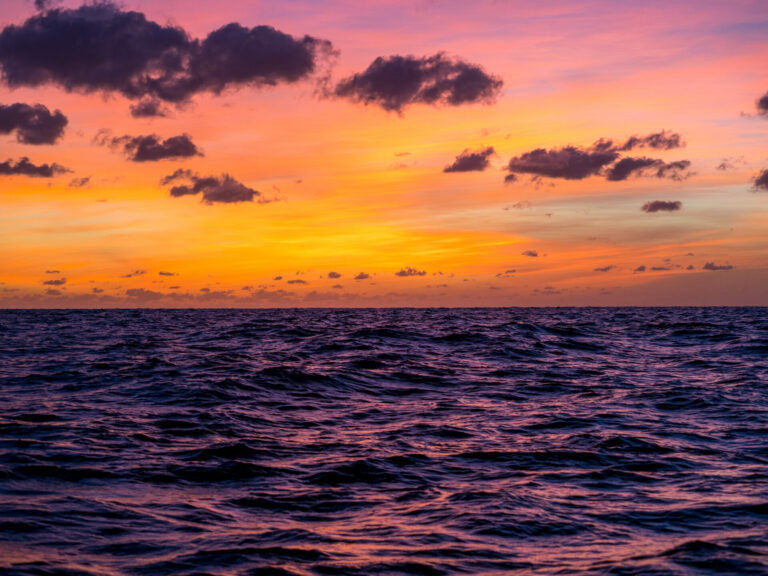
Applied Mathematics and Computational Sciences
Going likelihood-free with neural networks
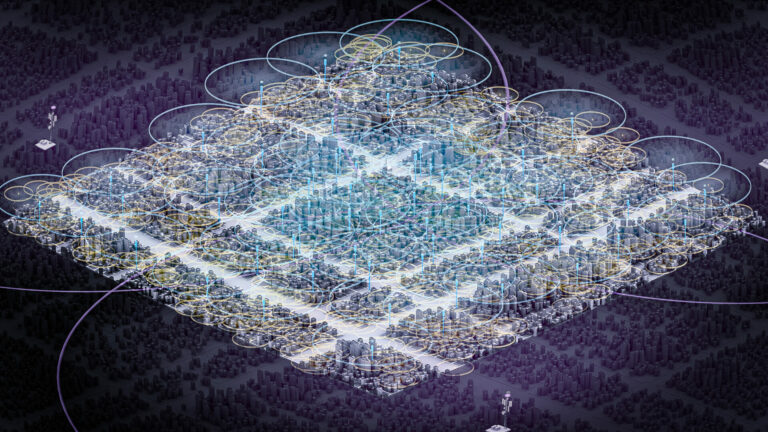
Applied Mathematics and Computational Sciences
A simple solution for frequency sharing
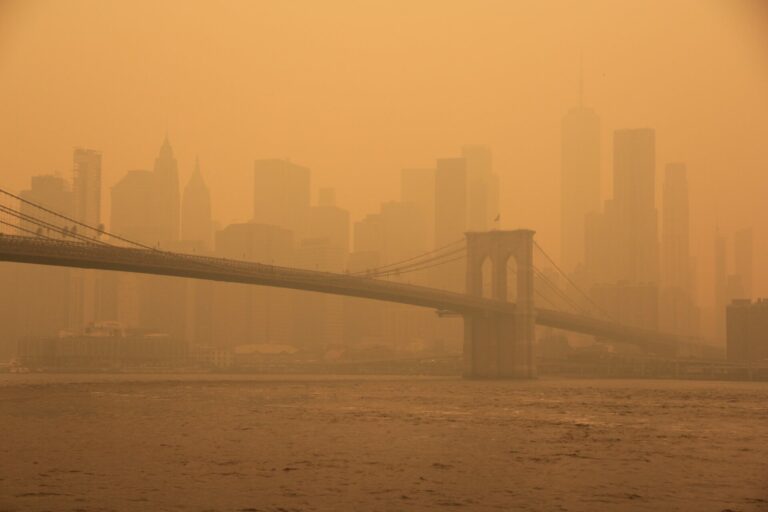
Applied Mathematics and Computational Sciences
Neural networks give deeper insights
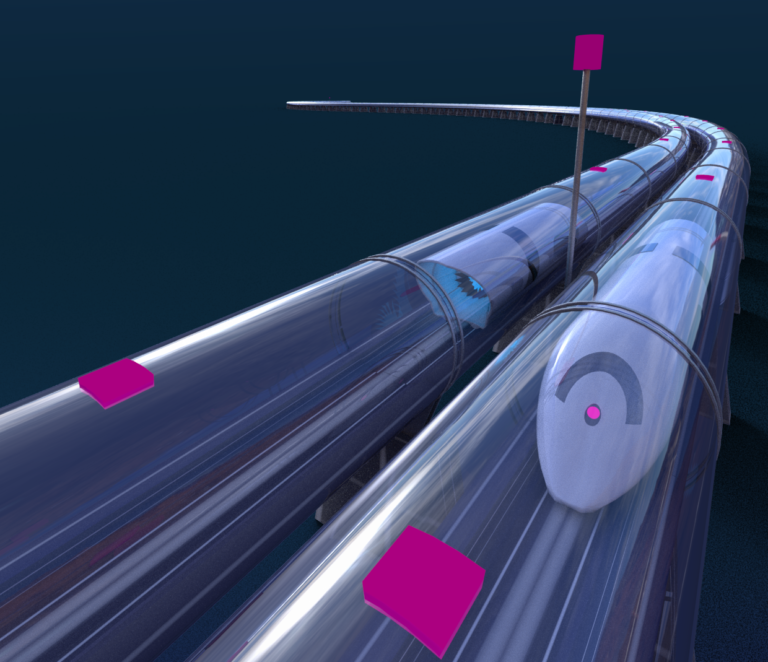
Applied Mathematics and Computational Sciences
Staying online in the high-speed tube of the future
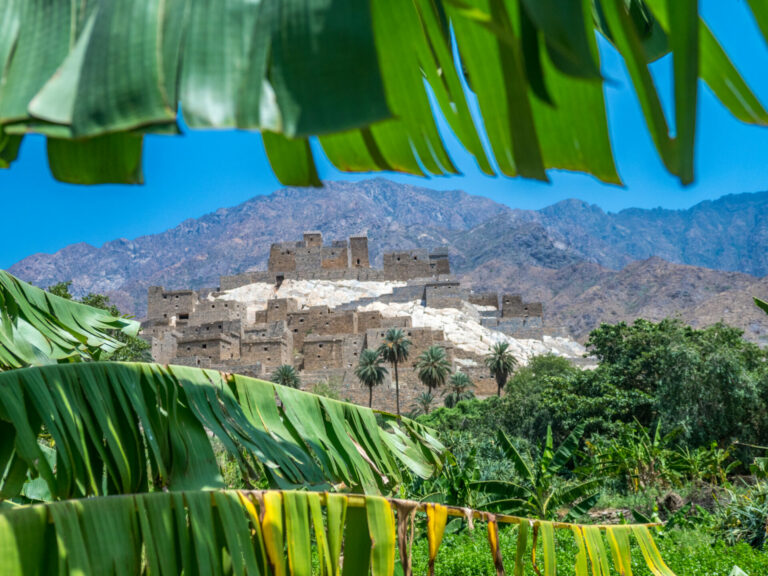
Applied Mathematics and Computational Sciences