Electrical Engineering
Testing the blind spots in artificial intelligence
Understanding the situations when artificial intelligence can fail is critical for application of future autonomous vehicles and medical diagnostics.
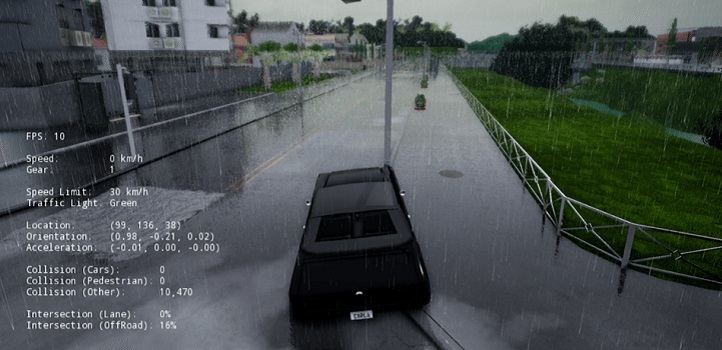
Deep-trained artificial intelligence models can make mistakes if they encounter scenes that they do not recognize, such as an object in an orientation, color, lighting or weather (like in the example above) that conflicts with the datasets used to train the model. Reproduced from reference two © 2019 KAUST
By investigating the robustness of deep learning models using a context-based approach, KAUST researchers have developed a means to predict situations in which artificial intelligence might fail.
Artificial intelligence (AI) is becoming increasingly common as a technology that helps automated systems make better and more adaptive decisions. AI is an algorithm that allows a system to learn from its environment and available inputs. In advanced applications, such as self-driving cars, AI is trained using an approach called deep learning, which relies solely on large volumes of sensor data without human involvement. However, these machine learning systems can fail when the training data misleads the decision making process.
In Bernard Ghanem’s team, Abdullah Hamdi and Matthias Müller have been researching the limitations of AI and deep neural networks for safety-critical applications, such as self-driving cars.
This simulation shows how a self-driving car system can be derailed by a change in the environmental lighting selected by the researchers’ trick algorithm; this is followed by a simulation showing how an autonomous drone is continually fooled by a course generated by the researchers’ trick algorithm.
© 2019 Abdullah Hamdi
“AI and deep learning are very powerful, but the technology can fail in rare “edge” cases, which are likely to eventually happen in real-world scenarios when these deep learning models are used in our daily lives,” he explains.
For many applications, an AI failure is merely an inconvenience as long as the AI works as expected most of the time. However, for applications, such as self-driving cars and medical diagnosis in which failure can result in death or catastrophe, repeated unexpected failures are not tolerated.
“Deep learning is successful in a wide range of tasks but no one knows exactly why it works and when it fails,” says Hamdi. “Because deep learning models will be used more and more in the future, we should not put blind faith in those that are not well understood and that can put lives at risk.”
The team has shown how these very powerful AI tools can fail badly in seemingly trivial scenarios. They developed a method to analyze these scenarios and establish “risk” maps that identify these risks in real applications.
“AI models are commonly tested for robustness using pixel perturbations, that is, adding noise to an image to try to fool the deep-trained network,” says Hamdi. “However, artificial pixel perturbations are actually unlikely to occur in real-life applications . It is much more likely that semantic or contextual scenarios occur that the networks have not been trained on.”
For example, rather than being faced with a manipulated image of an object, an autonomous driving system is more likely to be faced with an object orientation or a lighting scenario that it has not learned or encountered before; as a result, the system may not recognize what could be another vehicle or nearby pedestrian. This is the type of failure that has occurred in a number of high-profile incidents involving self-driving vehicles.
“Our approach involves training another deep network to challenge the deep learning model. This will allow us to discover the weaknesses and strengths of the AI model that will eventually be used in applications,” says Hamdi.
The researchers expect their AI approach to contribute to the development of robust AI models and the verification of deep learning technologies before they are applied.
References
You might also like
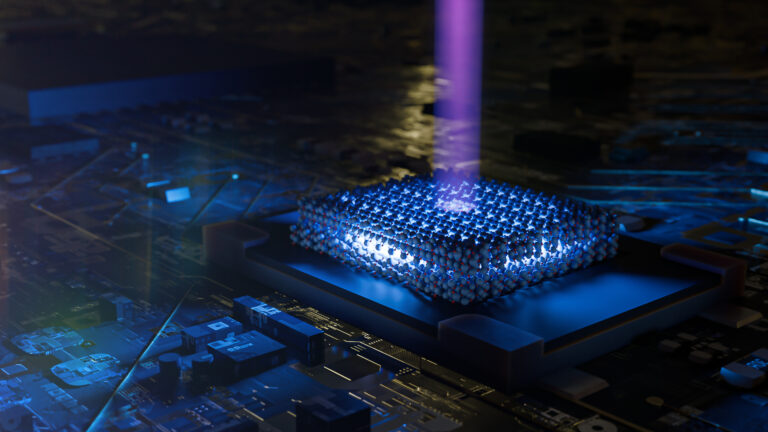
Electrical Engineering
A new interface for efficient electronics
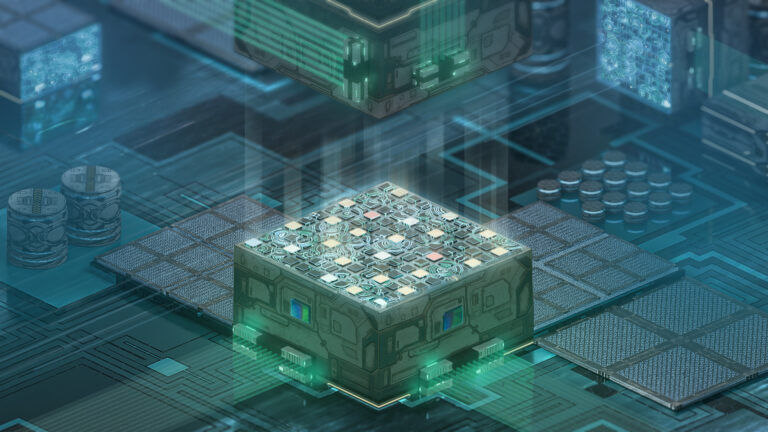
Electrical Engineering
Artificial neurons enable neuromorphic computing with light
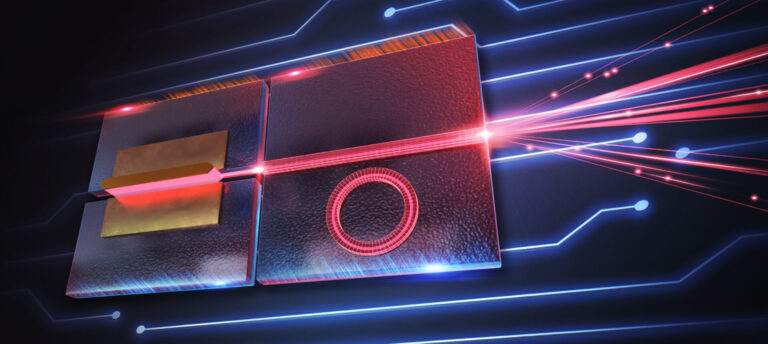
Electrical Engineering
Narrow-linewidth lasers bring low-noise answer
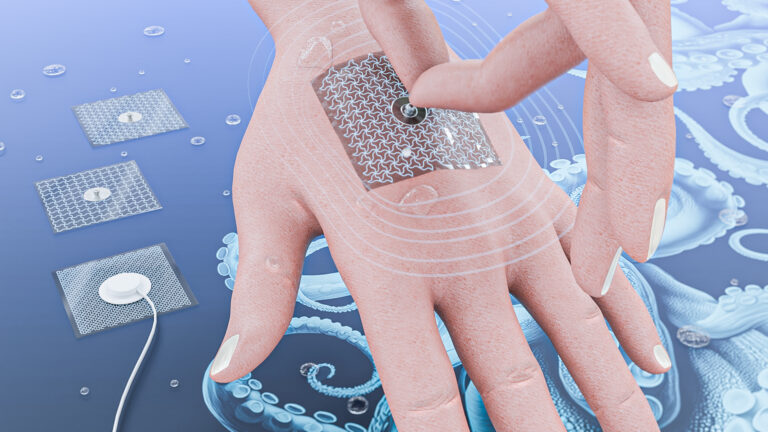
Electrical Engineering
Octopus suckers inspire sticky medical patch
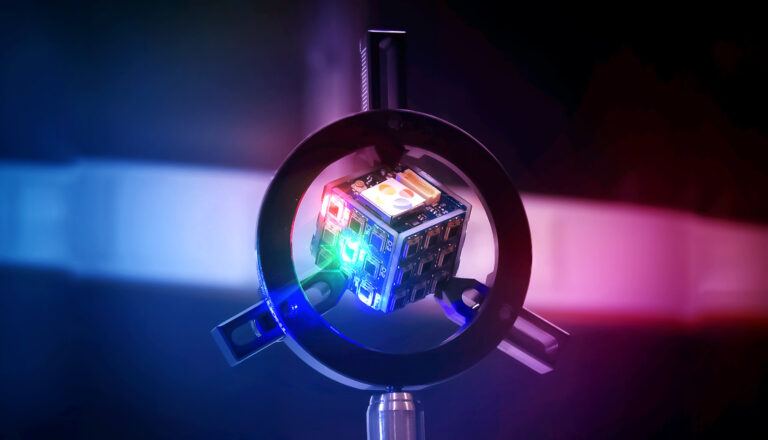
Electrical Engineering
Powering the future of the Internet of Things
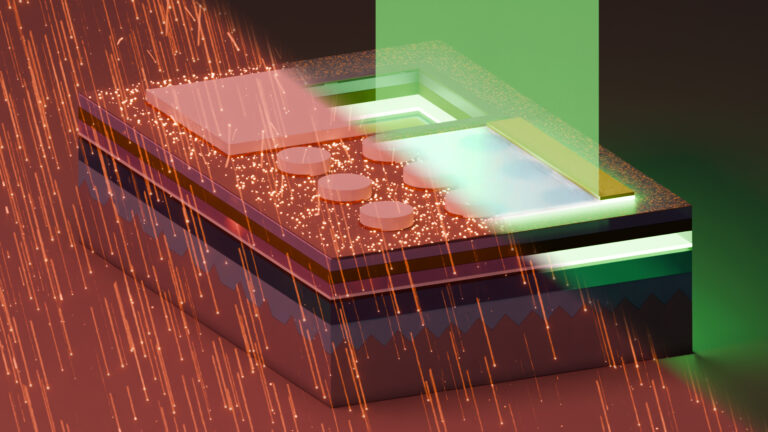
Computer Science
A new path to high-efficiency micro-LEDs
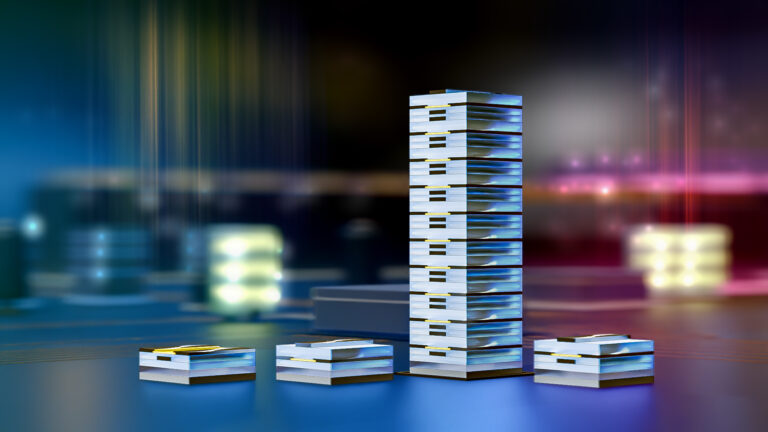
Applied Physics
Onward and upward to smaller faster devices
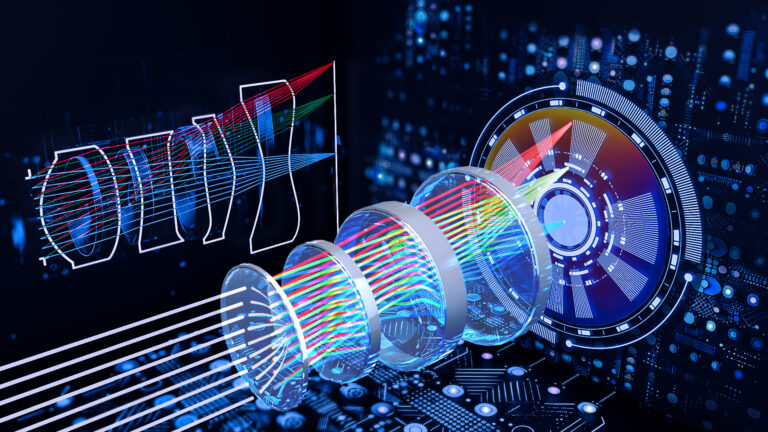
Computer Science