Electrical Engineering
Blink and you’ll miss it
A faster approach for computer detection of moving objects in video takes us a step closer to mimicking the reflexive detection ability of the animal visual system.
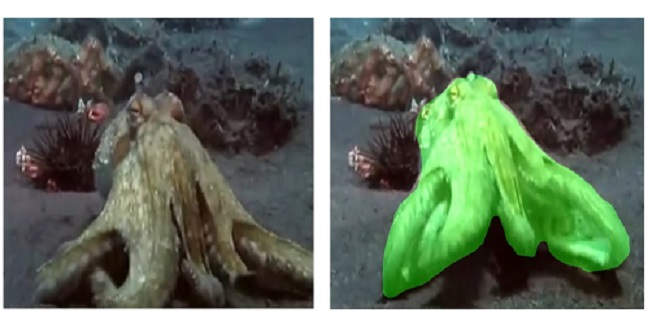

A fast motion detection algorithm breaks new ground in the rapid detection of moving objects in video sequences.
© 2017 KAUST
The detection of moving objects is one of the most fundamental and important mechanisms of the animal visual system, having evolved to quickly detect both predators and prey. Yet reproducing the ability of animals to reflexively detect moving objects has remained elusive.
Ganesh Sundaramoorthi and his KAUST Master’s student Dong Lao have developed a new approach to this problem using a statistics-based computational framework that minimizes the detection time for a given level of detection accuracy.
“Studies in human and animal vision indicate that motion plays a key role in detecting objects in a scene as the animal moves,” explains Sundaramoorthi. “Researchers have tried to use motion cues to detect objects in video, but so far the problem is unsolved.”
The difficulty in detecting moving objects in video stems from the ambiguities in computed motion between sequential frames—there are many possible motions that could create the image sequence—and the essentially infinite dimensionality of the computational problem. Detection schemes also have to deal with the apparent motion of pixels in the image, due to movement of the camera or observer, and distinguish this from object motion. Image noise and nuisances, such as changes in lighting and deformation of objects, also add to the difficulties of computing and segmenting motion.
“We address a fundamental problem that may be solved by the animal visual system—the tradeoff between speed and accuracy,” says Sundaramoorthi. “For instance, an antelope’s visual system must be able to quickly detect a cheetah or it will be eaten and can afford a high level of false detections as long as they happen quickly. We are the first to address this problem in computer vision, which is important not only in modeling the animal visual system, but also in real-world applications, such as robotic control and surveillance.”
Lao and Sundaramoorthi adapted what is known as the quickest detection framework in statistics, which converts the problem into a stochastic process with minimum detection time set according to a statistical detection threshold.
“The problem was technically challenging because the quickest detection framework is meant for 1D data not for the infinite dimensional time series of sequential video images,” says Sundaramoorthi. “However, we were able to construct the optimization tools needed to solve the problem with reasonable speed.”
Tests of the mathematical framework using a selection of videos showed that Lao and Sundaramoorthi’s detection scheme consistently beats other state-of-the-art methods in both speed and accuracy and with further optimizations could one day achieve real-time object detection.
References
- Lao, D. & Sundaramoorthi, G. Minimum delay moving object detection. Computer Vision and Pattern Recognition arXiv, 1605.07369 (2016).| article
You might also like
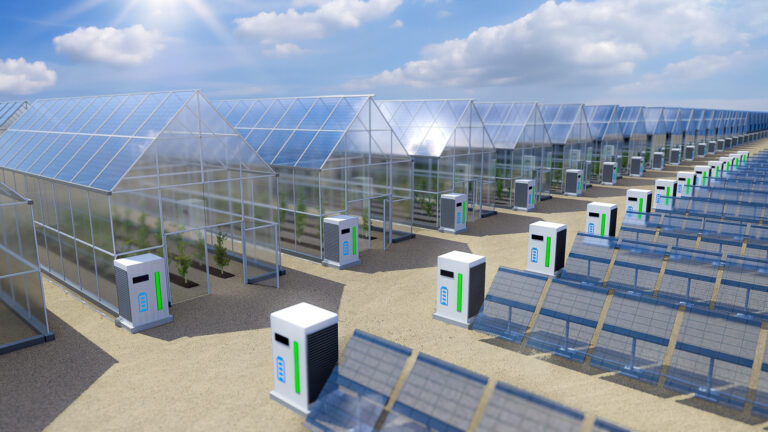
Electrical Engineering
A shade closer to more efficient organic photovoltaics
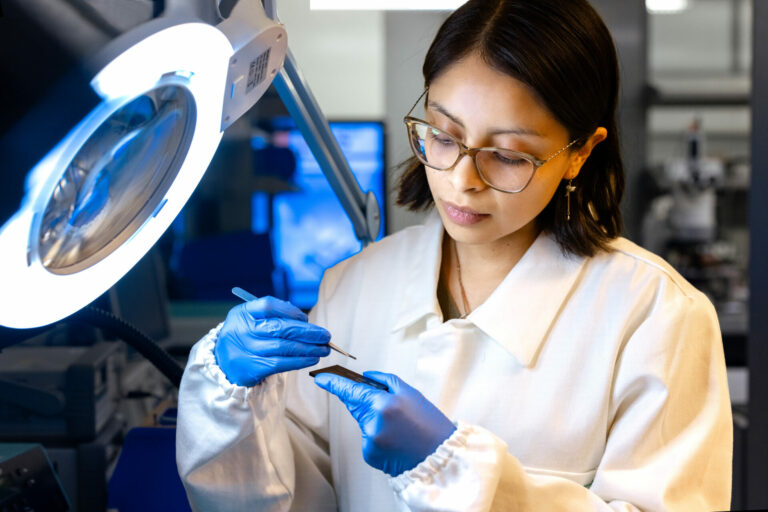
Bioengineering
Giving voice to the voiceless through assistive technology
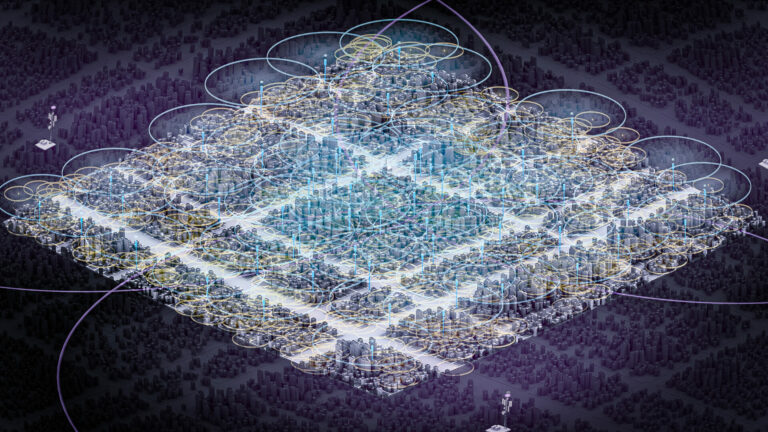
Applied Mathematics and Computational Sciences
A simple solution for frequency sharing
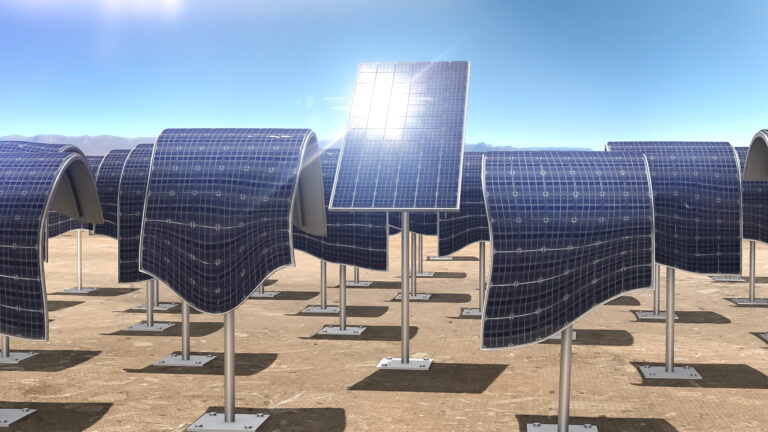
Electrical Engineering
Best thermal stability and efficiency in organic solar cells
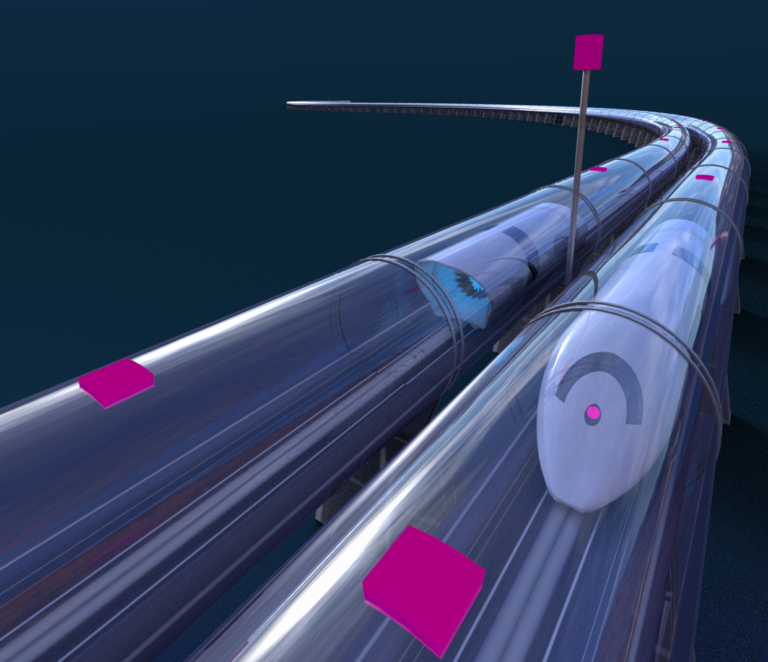
Applied Mathematics and Computational Sciences
Staying online in the high-speed tube of the future
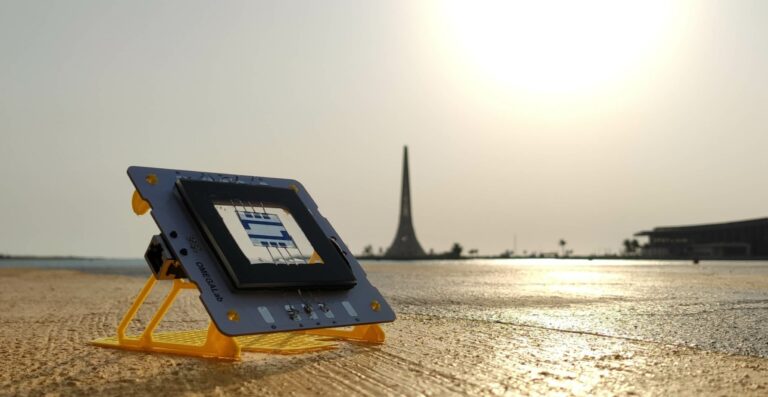
Electrical Engineering
Seeking stability to support sustainable outdoor solar cells
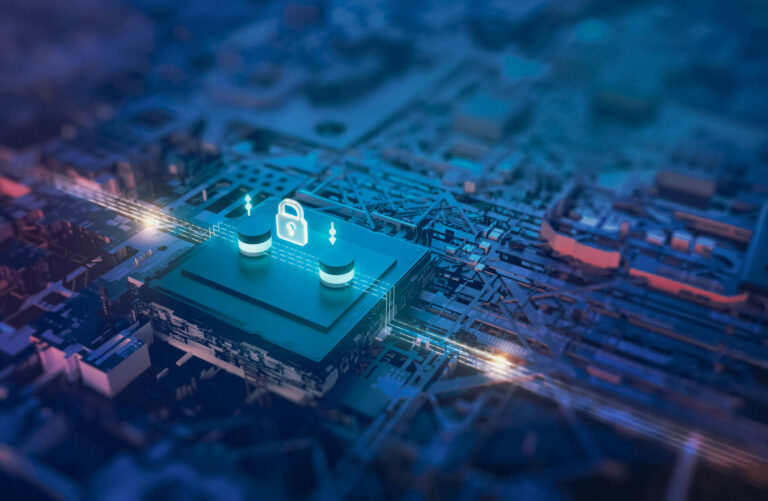
Computer Science
Outsmarting cyberattacks on microgrids
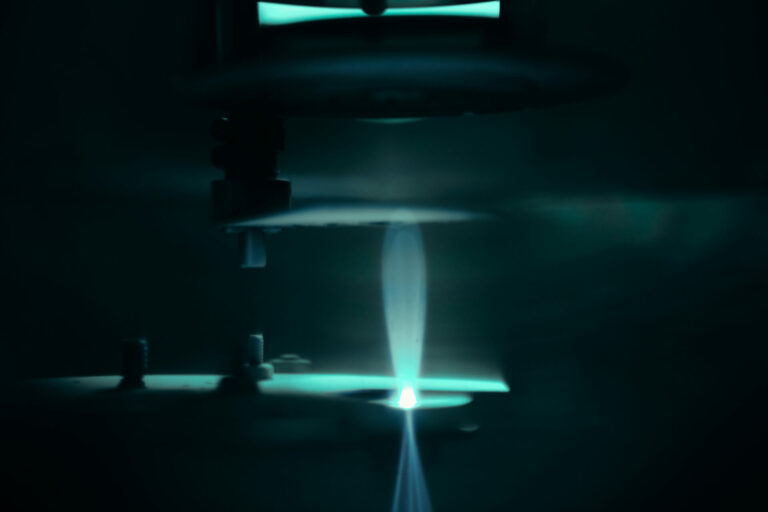
Applied Physics