Electrical Engineering
Helping computers to see who we really are
Algorithms that train computers to automatically detect human activity in videos can improve online searches and real-world surveillance systems.
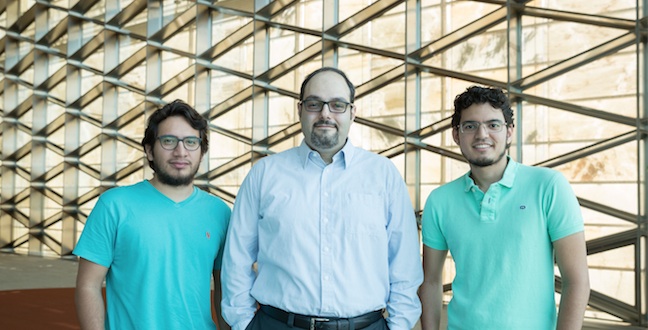
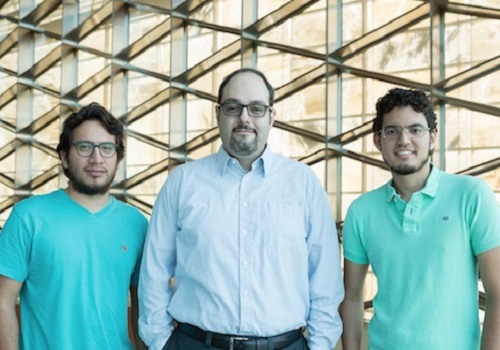
Ph.D. students Fabian Caba (left) and Victor Escorcia (right) are working with Bernard Ghanem (center) to automate visual recognition technology.
© 2016 KAUST
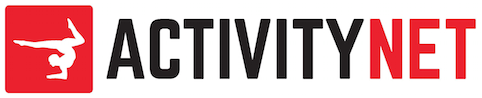
ActivityNet is a large-scale video benchmark for understanding human activities.
© 2016 ActivityNet
Recent statistics from YouTube reveal that users are uploading incredible volumes of content—nearly 1000 days’ worth of videos are added every hour to the website. While these numbers may bring joy to browsers of the internet, they challenge companies such as Google who must process enormous amounts of visual information with minimal human intervention.
Now, KAUST researchers are spearheading efforts to transform videos into tools that teach computers fundamental skills about identifying people and their behavior. And two members of the YouTube generation—Ph.D. students Fabian Caba and Victor Escorcia—are playing key roles in developing this technology, which may be applied in fields ranging from online advertising to 24-hour patient monitoring.
“Given the large amount of video data in the world, there is a need for algorithms with the ability to automatically recognize and understand human activities,” said Caba. “Finding ways for software to match humans at analyzing images, it’s one of the holy grails of computer vision research. It’s what attracted me to this domain.”
Both Caba and Escorcia are originally from Colombia, where they attended the Universidad del Norte in Barranquilla as undergraduates. Under the direction of Juan Carlos Niebles, the pair developed innovative techniques for computerized video analysis. For example, Escorcia used a Microsoft Kinect sensor to capture the body movements of actors demonstrating construction work, and then turned these poses into parameters for a machine learning algorithm. After being trained with the image data, this software automatically distinguished different construction activities in videos with an 85 percent success rate.
Caba approached the task with a system to recognize video context by looking for clues in camera motion and scene appearance. By segmenting pixel data into foreground and background, his system looked for distinct changes in appearance or perspective—the upward movement of a camera following a pole vaulter, for instance—to train machine learning software.
These projects put Caba and Escorcia in collaboration with Bernard Ghanem, an assistant professor of electrical engineering at KAUST. Previous work between Ghanem and Carlos Niebles resulted in a 2014 Google Faculty Award and inspired the undergraduate students to work with Ghanem. He introduced them to the KAUST Visiting Student Research Program (VSRP)—an opportunity for talented students to work with a faculty mentor for up to six months. They accepted the internship and soon realized that Ghanem’s expertise meshed perfectly with their career goals.
“I was looking for an opportunity in computer vision research and the VSRP gave me a hand in a sense,” said Escorcia. “Having the opportunity to work with Professor Ghanem before starting my Ph.D. program gave me a clearer idea about my research topic and the person who advises me.”
After their visit to KAUST, both students applied for and were accepted into Ph.D. programs working on automating visual recognition software. Video-analyzing algorithms rely heavily on datasets known as benchmarks that contain examples of human activities. Typically, these benchmarks have a small number of categories, and tend to over-focus on areas such as sports while underrepresenting the more regular events of daily life. As part of their studies, Caba and Escorcia are working to change this with a hugely expanded benchmark known as ActivityNet.
The ActivityNet project uses crowdsourcing, in combination with a rich taxonomy system, to classify hundreds of real-world human interactions. The researchers first searched the web for videos depicting specific activities and then used Amazon’s ‘Mechanical Turk’ web service—an on-demand, online workforce willing to perform small tasks for a fee—to filter out unrelated actions. Finally, the Mechanical Turk workers annotate the video timeframes associated with the activity.
Escorcia notes that expansive databases like ActivityNet provide a foundation for computer vision researchers to tackle machine learning problems, such as mimicking neural networks, previously considered out of scope. “ActivityNet will help us in the same way roads and the electrical grid move the world.”
Caba and Escorcia also challenged researchers to utilize their large-scale benchmarking tool at the first annual ActivityNet competition, run as part of the Conference on Computer Vision and Pattern Recognition in July 2016. This meeting used a competitive leaderboard system to motivate development of new video analysis algorithms with many possible applications.
“These methods could aid content-based video retrieval and ad placement for sites such as YouTube,” said Caba. “Moreover, these techniques can enable automated detection and recognition of abnormal or dangerous activities in security-sensitive areas such as airports or oil refineries.”
You might also like
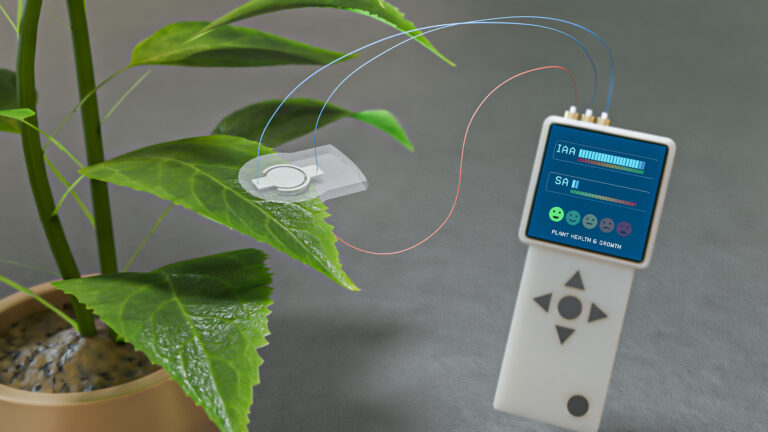
Bioengineering
Sensing stress to keep plants safe
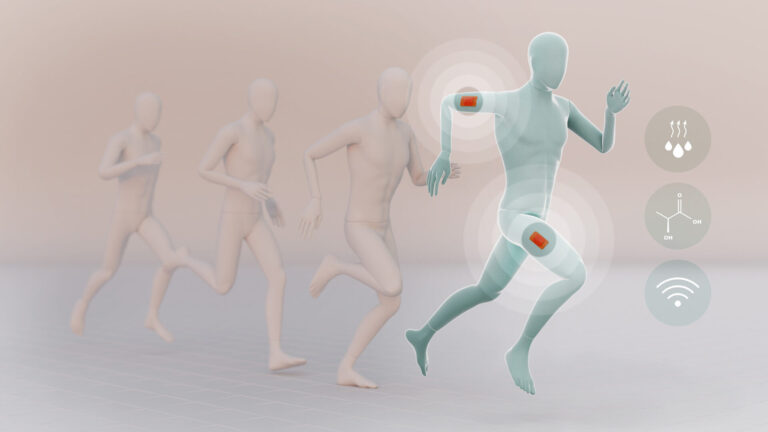
Computer Science
Sweat-sniffing sensor could make workouts smarter
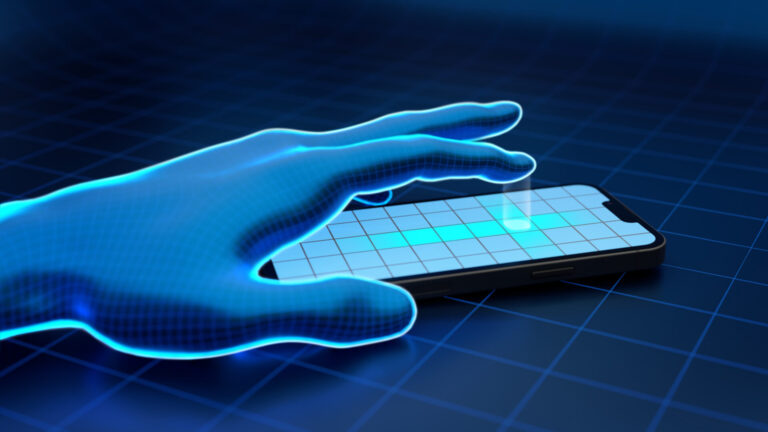
Electrical Engineering
New tech detects dehydration by touching a screen
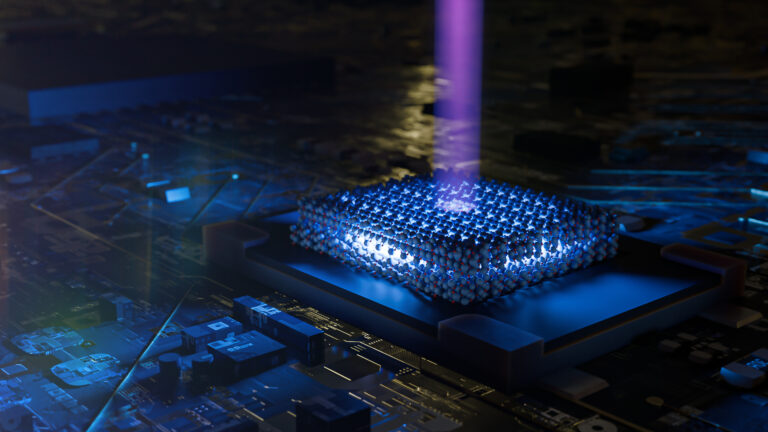
Electrical Engineering
A new interface for efficient electronics
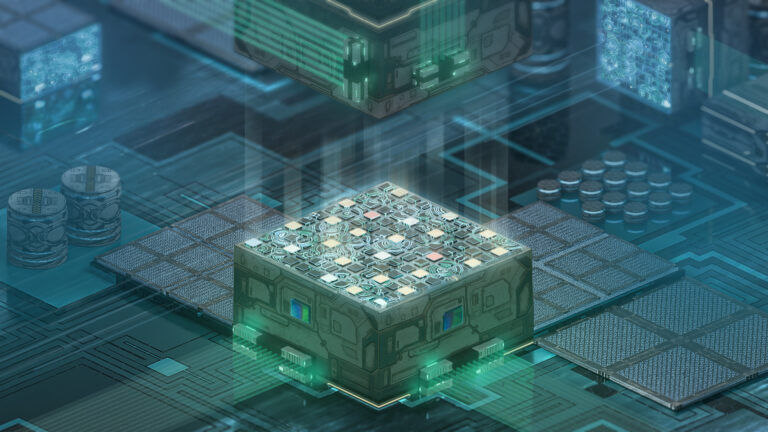
Electrical Engineering
Artificial neurons enable neuromorphic computing with light
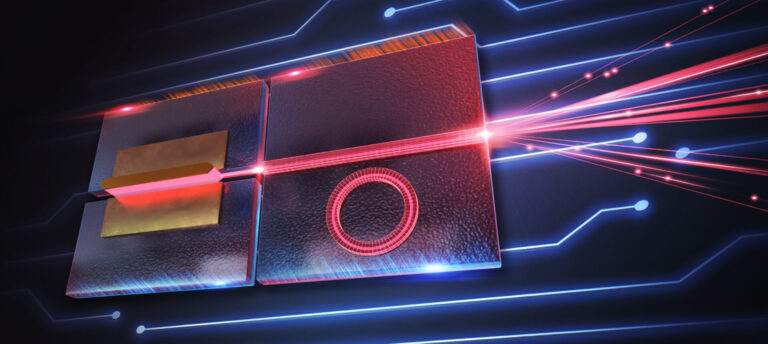
Electrical Engineering
Narrow-linewidth lasers bring low-noise answer
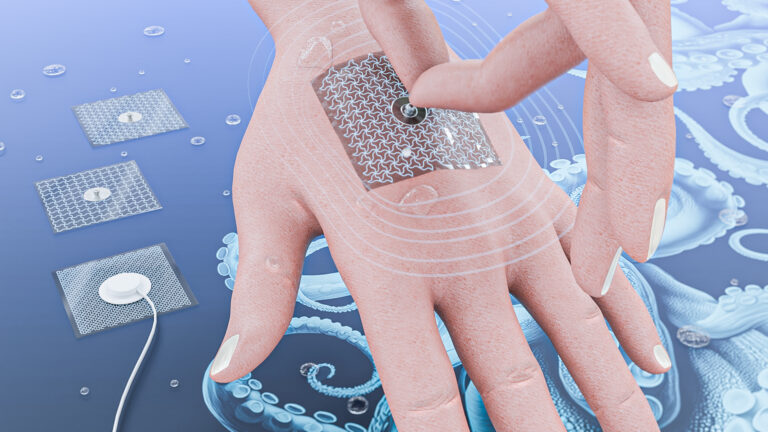
Electrical Engineering
Octopus suckers inspire sticky medical patch
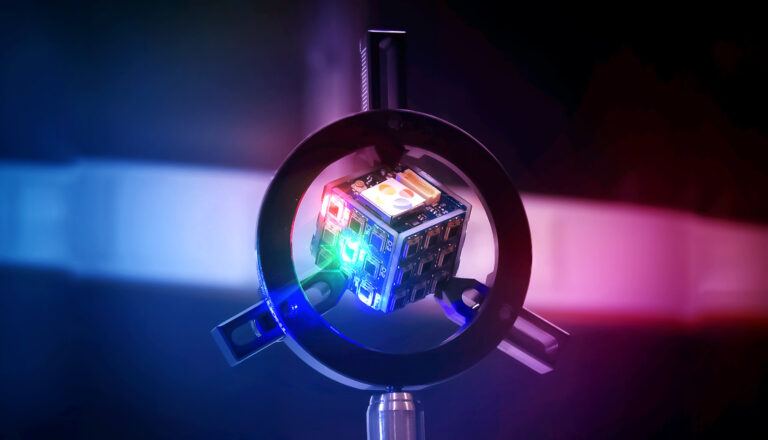
Electrical Engineering